Google AI: Predicting Heart Disease in the Blink of an Eye
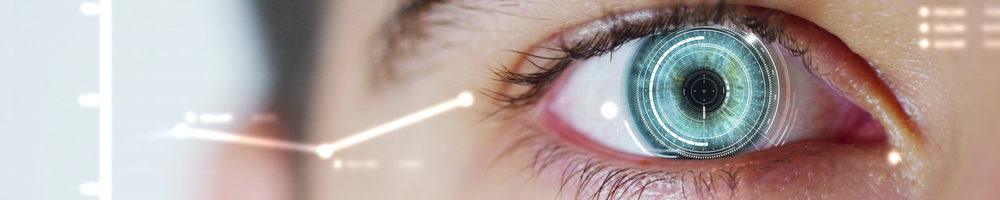
Google AI researchers use machine learning to predict risk factors for cardiovascular disease using photographs of the retina.
Background and Current Methods
New research from Google and its health tech division, Verily, indicates that there may soon be a far easier way to evaluate a patient’s risk of cardiovascular disease, the world’s leading cause of death [1]. Google researchers have used machine learning to “extract new knowledge from retinal fundus images” in order to predict if a patient is at risk of suffering from a cardiovascular event, such as a heart attack, within five years [2].
Currently, doctors rely on blood tests to determine a patient’s risk of suffering from a cardiovascular event [3]. Blood tests use invasive methods of drawing blood and require time to test and analyze the results. Google’s research indicates that retinal exams can be used instead, which are less invasive, easier to obtain, and faster to analyze with machine learning.
Google’s Breakthrough Research
Researchers at Google used retinal fundal images, which show blood vessels at the back of the eye, to predict various risk factors, such as age, gender, blood pressure, and smoking, that influence major adverse cardiac events. The risk factor predictions are then used as inputs to an algorithm to predict the risk of a cardiovascular event [4].
Google researchers used deep learning, a machine learning technique which “allows computational models that are composed of multiple processing layers to learn representations of data with multiple levels of abstraction” [5]. Data from 284,335 patients was used to train the deep learning algorithm, which then predicted cardiovascular risk factors for nearly 12,025 patients with very high accuracy. For instance, the algorithm “was able to predict a person’s age to within 3.26 years, smoking status with 71% accuracy, and blood pressure within 11 units of the upper number reported in their measurement”, which a doctor cannot typically predict [2]. The algorithm then used the entire retinal image to determine the association between the image and the risk of heart attack or stroke. The algorithm was able to identify a patient at risk of a cardiovascular event with 70% accuracy, which is competitive with the European SCORE method, currently used to predict risk based on blood tests, at 72% accuracy [2].
Additionally, the system can generate attention maps that visually highlight how the algorithm is arriving at its conclusion. In the image below, the attention map indicates areas that correlate to various factors in green [6]. This is particularly exciting since it provides insight into which aspects of the retina contributed most to the algorithm, providing a view into a typically opaque process of computing in machine learning. This provides additional information for doctors to better understand and trust the algorithm, since they can see how it is working.
Short and Medium Term Next Steps
In the short term, Google’s management is focused on validating these preliminary results. The current study is limited since it only used a 45° field of view and it used a smaller data set than average for deep learning analysis [7]. The algorithm will be validated and tested using larger data sets in the short term. In the medium term, Google plans to begin initial testing with patients in real-time. This will allow the researchers to continue to feed new images into the algorithm in order to validate its results and more accurately predict a patient’s risk of a cardiovascular event.
I would recommend that Google evaluate additional methods for obtaining retinal images in the short to medium term. While it will be groundbreaking to eliminate the need for blood testing for cardiovascular evaluation, Google can further eliminate patient barriers by making it easy for patients to take a simple photo with their iPhone to obtain a retinal image. This will allow patients to complete their cardiovascular evaluation from the comfort of their own home, rather than visiting a doctor’s office to obtain a retinal image.
Open Questions
- Evaluating patient health using AI and machine learning introduces the risk of hidden machine bias, which may result in incorrect diagnoses. How can Google use AI and machine learning to support medical professionals in their clinical work without relying too heavily on the machine to determine important patient information?
- Machine learning in health care requires a significant amount of data from healthy and unhealthy patients to improve the accuracy of the algorithm. Would you be willing to share your health data with companies like Google in order to support the development of machine learning algorithms for applications in health care?
(Word count: 762 words)
Sources:
[1] “The Top 10 Causes Of Death”. 2018. World Health Organization. http://www.who.int/en/news-room/fact-sheets/detail/the-top-10-causes-of-death.
[2] Loria, Kevin. 2018. “Google Has Developed A Way To Predict Your Risk Of A Heart Attack Just By Scanning Your Eye”. Business Insider. https://www.businessinsider.com/google-verily-predicts-cardiovascular-disease-with-eye-scans-2018-2.
[3] “Blood Tests For Heart Disease”. 2018. Mayo Clinic. https://www.mayoclinic.org/diseases-conditions/heart-disease/in-depth/heart-disease/art-20049357.
[4] Poplin, Ryan, Avinash V. Varadarajan, Katy Blumer, Yun Liu, Michael V. McConnell, Greg S. Corrado, Lily Peng, and Dale R. Webster. 2018. “Prediction Of Cardiovascular Risk Factors From Retinal Fundus Photographs Via Deep Learning”. Nature Biomedical Engineering 2 (3): 158-164. doi:10.1038/s41551-018-0195-0.
[5] LeCun, Yann, Yoshua Bengio, and Geoffrey Hinton. 2015. “Deep Learning”. Nature 521 (7553): 436-444. doi:10.1038/nature14539.
[6] Li, Abner, and Abner Li. 2018. “Alphabet’s Verily Analyzing Retinas W/ Machine Learning To Predict Heart Disease”. 9To5google. https://9to5google.com/2018/02/19/alphabet-verily-eyes-heart-disease/.
[7] “Google’s AI Uses Retinal Images To Reveal Cardiovascular Risk”. 2018. Medscape. https://www.medscape.com/viewarticle/893223.
In 2014, approximately 12 million Americans were misdiagnosed (~1 out of every 20 patients) and ~50% of those misdiagnoses resulted in real harm. [1] While I do worry about the risk of hidden machine bias and potential incorrect diagnosis in the field of medicine, I would hope that the use of machine learning and AI will improve the misdiagnosis rate through continuous improvement as more and more samples are added to the studies, creating a stronger, more accurate feedback loop. I do think that machine learning and AI are capable of transforming the healthcare industry and delivering simpler, more accurate diagnoses and treatments, but I think it is critical to retain human contact in the healthcare industry, as interaction with highly trained doctors brings patients comfort when undergoing trying or stressful times.
With regards to your second question, I would be willing to share my health data with Google to help improve their algorithms and contribute to better, simpler, more accurate healthcare diagnoses and treatments. However, I would only do this if Google kept everything I shared with them highly confidential and it was on a no-names basis, as this information is highly personal.
[1] https://www.cbsnews.com/news/12-million-americans-misdiagnosed-each-year-study-says/.
Interesting article!
For the first question, in my opinion, machine “bias” is always going to be an issue, but the extent of it for the short and long term is virtually non-existent for several reasons: First, machine learning relays heavily on the data set provided, as the we feed it with more data, the correct predictions rate will increase, and as you stated, this is their main short term goal.
Secondly, although the prediction rate of the machine is lower than that of doctors, this is just temporarily, for example, an algorithm in detecting skin cancer (which is also done using image processing) has already outperforms doctors by a significant number (95% vs 85.5%) [1]. So, until the prediction rate of the machine is lower than the prediction rate of the doctors, it can serve merely as an addition to the arsenal of tools doctors use for their predictions.
Regarding your second question, I think this is an extremely important issue, especially for a company such as google that its own business model right now relies on “selling” its customer information to advertising companies. As a result, it will have to put a strong emphasis on persuading their users that the data will not be used by the company for any other purpose than improving the accuracy of the algorithms.
[1] https://www.theguardian.com/society/2018/may/29/skin-cancer-computer-learns-to-detect-skin-cancer-more-accurately-than-a-doctor
The question you raise on hidden machine bias is an important one with many implications in not only the medical but the legal field as well. Who would be to blame in such instances? In the paper ‘Black Box Medicine’, Price notes that: “a large, rich dataset and machine learning techniques enable many predictions based on complex connections between patient characteristics and expected treatment results without explicitly identifying or understanding those connections.” However, by shifting pieces of the decision-making process to an algorithm, increased reliance on artificial intelligence and machine learning could complicate potential malpractice claims when doctors pursue improper treatment as the result of an algorithm error. I therefore agree with your choice of words in that AI merely ‘supports’ medical professionals rather than substitutes what they are currently doing. I would therefore believe the best course of action at the moment would be to focus on the complementarity of these tools to existing procedures rather than thinking about any potential substitution.
On your second point, I am pretty torn on this issue. There is a clear benefit from having a more reliant database to improve the algorithm for the greater societal good. However, it is not clear how these data can be used by the broader industry. It is not inconceivable to envision insurance companies being able to charge premiums based on the propensity towards having certain cardiovascular conditions which might have negative implications for consumers.