Visual Deep Learning – The Power of Images at Pinterest
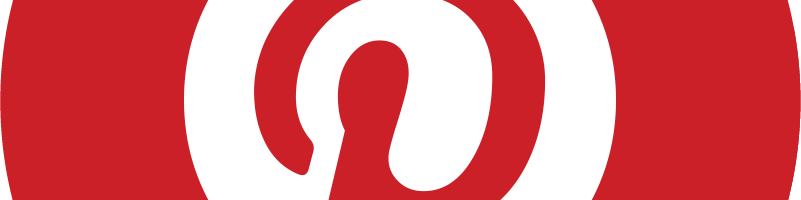
Pinterest – leading the field in extracting information from visual content.
Machine Learning at Pinterest
The ability to recognize and classify images is fundamental to Pinterest, a web and mobile company where users can discover and curate digital vision boards. At the core of this social media company is robust machine learning and deep learning. Ranging from the Discovery team providing recommendations and re-pin recommendations, to the Visual Discovery team creating algorithms to do object recognition and related object suggestions, to the Monetization team developing ad performance algorithms[i] – Pinterest’s data science team is at the cutting edge.
Why Pinterest Uses Machine Learning
Pinners (term used by Pinterest for their customers) use Pinterest to search hundreds of thousands of photos to “discover and save ideas.”[ii] Given the large number of Pinner interactions with the website or app, the company has a rich abundance of data. As reported, “Pinterest hosts more than 30 billion Pins (and growing) with rich contextual and visual information. Tens of millions of Pinners (users) interact with the site every day.”[iii]
To continue growing, Pinterest needs to succeed on two fronts – recommendation and search. If the promoted pins, advertisement pins, or related pins from other Pinners are not accurate, the Pinner is likely to churn. In this example, Pinterest has failed to recommend good pins on the user’s home feed. Similarly, Pinners are likely to stop using Pinterest if, when using the search function, they find irrelevant images that do not relate to their search. This is fundamentally why Pinterest uses machine learning. They need to make sense of their wide array of data and use this data to accurately recommend and provide search capabilities.
How Pinterest Uses Machine Learning
To provide high quality recommendations, Pinterest has developed Related Pins (2014). Related Pins helps Pinners find the best content on their home feed, by estimating the “relevance score of how likely a Pinner will interact with a Pin.”[iv] Using deep learning to generate these recommendations, testing has shown “increased engagement with Related Pins by 5%.”[v]
Similarly, for search, Pinterest has developed machine learning tools – including Pinterest Lens, which allows a user to upload a picture and be provided similar images (such as recipes, desserts, or fruit platters after uploading a photo of a blueberry). [vi]
Programs like the ones above are what the organization has been doing to address the need for machine learning in the short-term. Additionally, Pinterest has both grown their own team, the Pinterest Labs, while also aggressively acquiring companies already in this space. In 2014 Pinterest acquired VisualGraph, and in 2015 Kosei, “a machine learning company that specialized in commercial applications of machine learning tech (such as content discovery and recommendation algorithms).”[vii], [viii] Through these types of acquisitions, Pinterest is positioning itself well for the future medium and long-term growth. They are becoming industry experts in this field, publishing reports and leading talks with academics about machine learning in search and recommendation.
Additional Levers to Pull and Open Questions
While Pinterest is providing cutting edge visual recognition algorithms, I think management needs to consider ways for Pinners to provide additional data. Currently the data that is being collected is passive data, as in data not explicitly supplied by the Pinner. I believe there is an opportunity to further improve the recommendation and search algorithms by implementing a “pin not relevant” button. This will allow Pinners to indicate they are not interested, and therefore providing a direct cue that the recommendation or search algorithm has failed.
As Pinterest continues to improve their machine learning capabilities I wonder what other companies or social media adjacent companies they could partner with. The wealth of data, specially the wealth of adjacency data, would be relevant for other companies. For example, if a Pinner is likely to pin certain types of dresses and certain types of shoes – this might encourage a designer or retailer to create and package these types of products together. Does Pinterest see their data as a source of additional revenue? Would they ever consider creating their own product lines, like Stitch Fix, using the information they currently have to validate likely high-selling products?
(Word count: 798)
i. Michael Lopp, “The future of machine learning at Pinterest”, Pinterest Labs, January 21, 2015, https://medium.com/@Pinterest_Engineering/the-future-of-machine-learning-at-pinterest-88e6d4bf1968, accessed November 2018.
ii. Dmitry Kisyuk et. al., “Human Curation and Convnets: Powering Item-to-Item Recommendations on Pinterest”, Pinterest Labs, November 12, 2015, https://arxiv.org/pdf/1511.04003.pdf, accessed November 2018.
iii. Yunsong Guo, “Pinnability: Machine learning in the home feed”, March 20, 2015, https://medium.com/@Pinterest_Engineering/pinnability-machine-learning-in-the-home-feed-64be2074bf60, accessed November 2018.
iv. Yunsong Guo, “Pinnability: Machine learning in the home feed”.
v. Kevin Ma, “Applying deep learning to Related Pins”, January 12, 2017, https://medium.com/the-graph/applying-deep-learning-to-related-pins-a6fee3c92f5e, accessed November 2018.
vi. Andrew Zhai et. Al., “Visual Discovery at Pinterest”.
vii. Michael Lopp, “The future of machine learning at Pinterest”.
viii. Cooper Smith, “SOCIAL INSIGHTS: Facebook and Pinterest Bulk Up Their Big Data Teams”, Business Insider Intelligence, Jan 7, 2014, https://intelligence.businessinsider.com/pinterest-bulks-up-its-big-data-team-2014-1, accessed November 2018.
In a world where we commercialize data all the time, I think selling its data would be a significant revenue generator for Pintrest. That being said, I have some ethical qualms with the selling of consumer data, particularly without the express permission of the consumer.
As a sometimes-user of Pinterest, I find the information in this article both highly interesting and also generally concerning. I completely agree with the author that in order to scale its operations and provide trusted, relevant information to users, it needs to rely heavily on its machine learning capabilities. Additionally, in order to continue to expand, I imagine that more emphasis will need to be placed on suggestions such as the one the author has shared above – a “pin not relevant” button. Each time I use Pinterest, I am pleasantly surprised by how accurate and relevant the pins are to whatever topic I am researching/learning about – overall, the company seems to have understood my likes and dislikes through past interactions with the interface.
On the other hand, I am worried that Pinterest will share my data (or has already shared my data) to further increase its revenue. The data and interactions on Pinterest are very valuable to many companies, especially those that need to understand the types of advertisements to show consumers to encourage them to purchase goods. We are already inundated with advertisements and buttons stating “click here to purchase” – I am worried that Pinterest will continue to grow this space and will ultimately become an online shopping portal itself. I think one of the only ways to discourage this from happening is to highlight the true value proposition of Pinterest to its management team – that it is best for browsing, finding ideas, getting inspired – and not for shopping and ad-blocking.
You suggest the option for the users to override the ML algorithm by implementing a “pin not relevant” button, but shouldn’t the machine be let run free, in the spirit that “consumers don’t really know what they want”?
Thank you for your contribution. Pinterest seems like a leading innovator in visual deep learning. It has cash, resources, and a high-potential business model to push new innovations. I would go a step further – what if Pinterest could morph relevant pins into new content? Just like a designer pulling together several client ideas? Intellectual Property rights would not be breached since the algorithm is simply getting inspiration to create a work of “art”. The customer on the other hand could have a more meaningful and value additive interaction with the service and stay locked in for a longer time. Moreover, what if you could morph some Amazon products into the picture, e.g. room design with Amazon basics bed frame? We are still debating the visual recognition potential of algorithms, but how about we make a leap towards visual creation?