Hiring Data Scientists in Football – Where are the Best People for People Analytics?
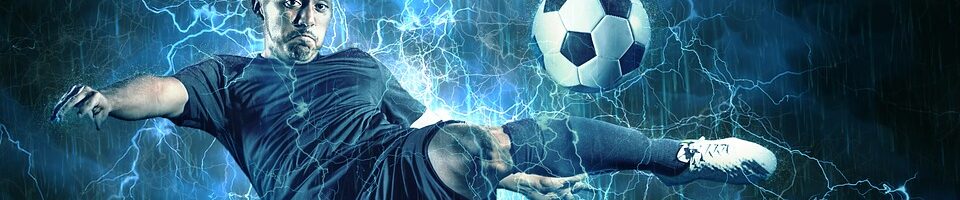
People analytics in sports and especially football is not new and supported teams finding the best players – but how do teams find the best data scientists for the job?
In a recent extensive piece on the use of data science in analyzing player performance in football (or soccer, for our US readers), New York Times author Rory Smith argues that while the use of data to support team management decisions like transfers, lineups and on-pitch tactics is now widely accepted, acquiring the right data science talent to drive these efforts remains a challenging task for teams.
The article describes the story of German Bundesliga team Eintracht Frankfurt and how their new sporting director hired his old team’s head of data analytics as one of the first steps after his appointment. Although largely unnoticed by media and fans, the move exemplified how rare good and proven talent in the football data analytics field currently is and which interesting challenges the market for such skills faces.
A lot of us remember reading the book or (like in my case as a die-hard Brad Pitt fan) watching the movie Moneyball, where the seemingly disadvantages baseball team Oakland Athletics uses a data-driven approach to scouting and game tactics to go on a record winning streak and disrupt the world of sports by proving that data can more than supplement manager experience. In football, a significantly more dynamic and interactive game, setting up hypotheses, deriving inferences or even predicting future outcomes is as challenging as it gets. And just like in the case of baseball, football coaches and scouts are usually strong characters that consider themselves more than capable of making the right decision based on their experience and game philosophy.
The article points out that even though most teams have embraced the usefulness of player data analysis and even data on coach behavior, the struggle lies in attributing performance to the benefits of player analytics while controlling for other variables. There are no treatment and control groups in football and running experiments by simulating real matches would still introduce noise, not to mention the costs and additional strain on player fitness.
An additional barrier in truly analyzing the impact of player analytics in football is the secrecy of teams on their methods and results. Thus, while a hired data scientists may be the best in the field and create incredible results (think Jonah Hill from Moneyball), the reality is that the public or even other team’s management will likely not find out, or as Smith puts it:“Sophistication of analysis and sophistication of presentation aren’t always the same thing.”
Personally, as a football fan and LPA player, I found the article insightful and somewhat amusing. It seems like the best way to solve the issue of hidden talents and unclear links between data scientist and team performance would be to gather more data, specifically on the data scientists, comparing their backgrounds, approaches and tactics. The article mentions first headhunting agencies to be evolving in the field, who likely focus on exactly that (and will require data scientists to support them). The industry seems to be growing and football fans, knowingly or not, are paying for it.
Hi Georgiy, I like your take. As a sports analytics geek, I never really considered how teams recruit technical talent. Because sport analytics has to do with setting the lineup, optimizing play-calling, valuing players and managing salary cap, the focus is always on the talent and team performance which means these analytics teams really never get any love. I’m actually not even sure if the GM on most teams hires data scientists, or sets the vision for the analytics team. It’s likely that interaction between Billy Beane and Peter Brand (Brad Pitt and Jonah Hill) in Moneyball probably isn’t how it actually works in most organizations. Then, you raise another good point: as an added complexity football is hard to model. Modern tech like non-invasive player and ball tracking helps, but still modeling football requires some serious data science skills. It will be interesting to see how clubs manage and recruit off-the-field talent moving forward.
Georgiy, I enjoyed reading this as a non-football fan 😉
The oberservation around sophistication on analysis vs. presentation seemed like a great comment on the general struggle of people analytics (or even us in People Analytics at times). Do you think creating a simple and intutive presentation of findings is even harder in this field given the complexitiy of human interactions?
Hey Georgiy – Amazing piece! As a football fan myself, I found your piece insightful and amusing. I have never thought of sports teams as a good fit for People Analytics but it seems quite a good fit indeed. I must agree with the notion that it is in fact a lot more complicated to apply people analytics to sports than it is to HR departments. However, the teams that do seem to benefit greatly (if er are to use the examples you provide in your blog). Perhaps what we need in sports to truly spearhead the use of PA is an analytics translator. What I mean by this is a person who does not necessarily have a data science background but can act as a bridge between the sports team and the data scientists. This McKinsey article – https://www.mckinsey.com/business-functions/mckinsey-analytics/our-insights/analytics-translator – explains the concept very well.