Drowning in Data: The Incoming Tech-sunami in People Analytics

Big data is here and it is set to revolutionise the way we work with each other. How do we best prepare for the incoming wave?
Imagine being able to predict which employees will work well together or how well connected the CEO of a company is as compared to the Project Manager. Tomas Chamorro-Premuzic’s recent article published in the Harvard Business Review entitled Tech Is Transforming People Analytics. Is That a Good Thing? explains that the incoming wave of technological and data-driven solutions to common human resource problems will change the way we work. But this is beyond a silver bullet solution. There are the risks of over-surveillance, crossing ethical boundaries into people’s personal lives, and the potential for over-dependency and inappropriately falling into the trap of the correlation-causation fallacy. So what does this all mean and what can we do about it?
Humans like to think that they are quite logical but there is always a healthy dose of bias and instinct that tend to form part of our judgments. For example, we struggle to conceptualize true randomness. If the winning lottery numbers were 1, 2, 3, 4, 5, 6, 7, and 8, a large majority would believe that the system was rigged. This is despite the lottery number sequence of 45, 23, 74, 12, 3, 63, 13, and 9 having the same probability of occurring, yet we wouldn’t question this. This phenomenon becomes important because data analysis involves taking seemingly ‘non-sensical’ data and modeling it into revealing important relationships. Sometimes these relationships will be true, and others might be through sheer coincidence. And it is during these scenarios that the relationship between two or more variables may not be causal and just be coincidental. Imagine analyzing data that implied that people who were shorter had higher productivity levels and that based on that, you hired only people of a certain height, only to find out that this wasn’t true. Think of the ramifications in terms of your brand perception, future productivity, and workplace culture (not to mention the ethical questions too!).
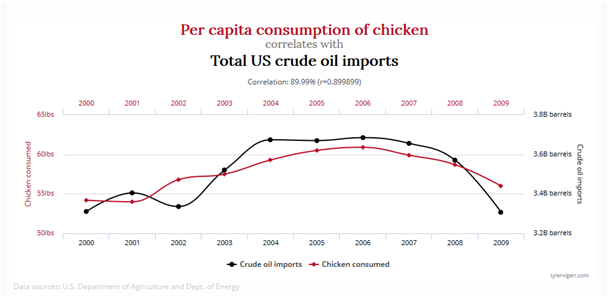
That is why, in this era of ‘big data’, it is crucial that hypothesis and statistical training is given to future practitioners. Laying out clear and descriptive questions to test mitigates drawing inappropriate conclusions and costly decisions. It also helps in understanding the ethical ramifications of future choices, thereby contributing to which data should and should not be collected.
Underlying this whole process is getting businesses to reach a consensus on how data concerning workforce performance should be used. Going through this process promotes best practices with regard to data collection, handling, and analysis that all businesses can benefit from in making informed choices about their workforce. By teaming up together, industry standards in People Analytics provide mutual benefits across industries in meeting acceptable standards. This reduces the potential of businesses making inappropriate conclusions that could be costly or breaching ethical standards that land them in legal trouble. It also protects workers in knowing that their performance is for the betterment of themselves as a developing employee and the organization that they work for. In doing so, human resource departments will be able to surf the incoming waves of big data rather than flail and tumble in the sea.
Hold on a minute, so are you telling me that I have been stocking up on chicken with the recent jump in gas prices for no reason? What a mind cluck… Jokes aside, I really appreciated your thoughts on this growing trend in people analytics. With the rise of technology and expansion of data science, I absolutely agree with your assessment that there is benefit and value to promoting “best practices with regard to data collection, handling, and analysis.” What resonated with me from your opinions here is that there is a careful balancing act between the human and resources, just as much so between the people and analytics. Veering too far from the center runs the risk of acting on intuition versus evidence or lacking sense in a wave of data overload. No doubt that it’s your awareness of all this that makes you such a strong leader. Thanks for the awesome share, Sam!
This got me thinking about how much I struggled initially in LPA despite being highly educated (compared to the general population) and relatively quantitatively trained (relative the key term there). I also remember feeling the temptation to draw easy conclusions as we were doing our LPA assignments. Though there are many in the workforce that are rigorously trained in statistical analysis, I wonder that the trajectory of growth in the sheer volume of data and analytical jobs may quickly outpace the appropriately trained labor pool. The supply demand imbalance here has potentially dangerous implications and feels inevitable – 20 years from now, I’m sure LPA will have plenty of cases about people analytics gone wrong with grim consequences for the company and its employees. It’s important as managers to keep this in mind and ensure we have the proper talent in place first before we jump into the mountains of data at our disposal.
Totally agree, Sam. Big data, machine learning, and AI somehow became buzzwords and everyone wants to use them but there are caveats to using data. I almost want to say that high school programs have to be gradually adapted. They should start educating their students (and future workforce) in applied data science, even the less mathematically gifted students. We should educate our students on how to use I, how to interpret it, and the risks of using data.
Thanks for sharing this opinion, Sam. It is important to keep a critical stance on the use and value of data and refine the way we train our teams not only in the technical but also in the critical aspect of data analysis, to ensure we are able to see beyond initial conclusions and provide better value.