Zoba: an AI-driven demand forecasting and optimization SaaS for shared mobility companies
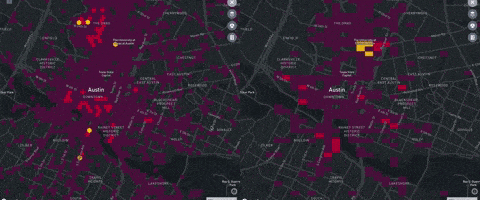
As shared mobility providers grow and need to validate multifaceted business models, Zoba brings a modular solution to increase the companies' ability to predict demand and optimize their fleets.
Background
Zoba is an early-stage spatial analytics start-up that offers demand-forecasting and optimization software to Micromobility and Car-Sharing companies. Customers can plug-and-play Zoba’s machine learning algorithms and combine their own data together with Zoba’s expertise (and featured data) to predict demand and optimize their fleet distribution to increase its utilization rate.

Micromobility and Car-Sharing solutions are expected to grow with a CAGR between 20 and 25% in the coming 5 years[1], with the Micromobility market reaching 300 USD billion by 2030[2]. The attractiveness of these two markets has brought together big OEM’s, mobility giants and an overwhelming quantity of local start-ups: all of them trying to validate a multifaceted business model in which operational excellence is critical to surviving in a fierce and thin-margins market.
Operational excellence requires adequate demand forecasting, disentangling utilization (the rides that consumers take) from actual demand (the rides that users would take if provided with proper supply). Only then, companies can optimize demand-supply tradeoffs by implementing rebalancing or variable pricing tactics.
A Scalable, Best-in-class and Real-Time Solution
Zoba offers its customers a scalable, best-in-class and real-time software that offsets the drawbacks of outsourcing the demand forecasting and fleet distribution algorithms.
- Flexible scalability: Through a simple API call, Zoba’s solution can handle the customer’s event data from day-one. Building those capabilities in-house is particularly challenging for companies in the space, where limited funding or human capital hinders the scalability of developing forecasting and optimization tools and slows down its development. Instead, Zoba offers a “turn-key” solution.
- Best-in-class: Zoba can develop highly accurate demand forecasting solutions by feeding its machine learning algorithms with larger sets of data than the ones that in-house solutions can access to. In fact, Zoba captures multiple information sources, including both customer’s generated data and external featured data. For example, a common pitfall of in-house built demand forecasting methods of Micromobility operators is to assume that high usage areas are the ones with high demand. In reality, “they are actually observing what is in large part a byproduct of where they have historically placed supply.”[3]To operators navigating these demand-supply feedback loops, Zoba can bring best-in-class solutions to predict actual demand and boost their utilization rates.
- Real-time decision-making: In addition to offering more accurate models in “stable” conditions, Zoba’s prediction and optimization package provides customers with the capability of capturing sudden variations (such as weather) in demand and redefine their fleet distribution to better adapt to these events and maximize their profitability.
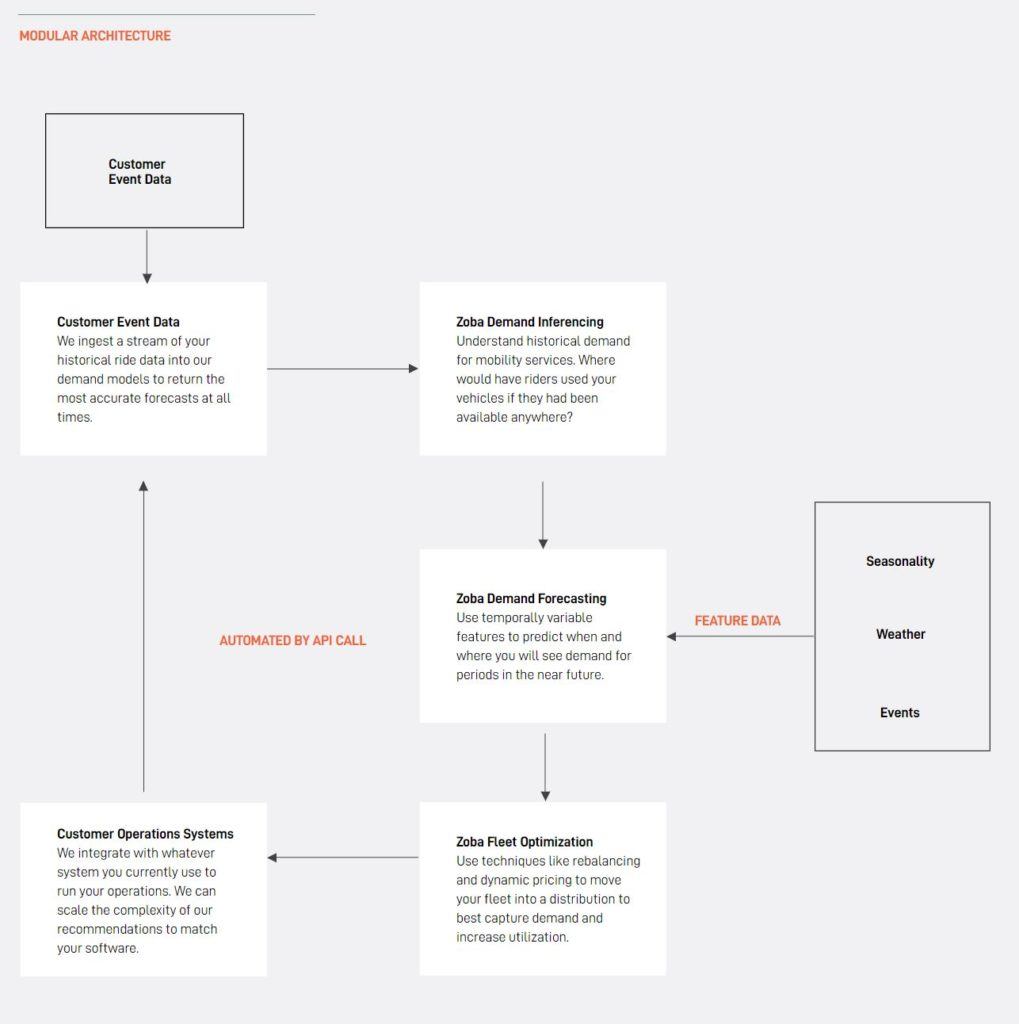
SaaS value capturing
Zoba charges shared mobility companies based on the metered access to its service. Since the company needs to navigate a wide array of customers (and their different sales cycles), going from small operators to OEM’s and large mobility players, its pricing scheme is probably developed on a case-by-case approach.
Main opportunities to expand value creation and value capture
- Profit-sharing agreements to boost data flywheel: on top of offering free-trials of its solution (up to one location selected by prospective customers), Zoba could expand its market penetration by engaging in cost-savings/profit sharing agreements (with upper and lower limits) based on the results generated by Zoba’s software implementation. This could reduce customer acquisition friction and speed up the data flywheel to improve its machine learning algorithms.
- Expand customer base to aggregators and planners: since the sharing mobility sector is part of a larger urban network, the estimation of actual demand (not supply!) for each vertical mode of transportation is highly valuable for mobility end-to-end platforms, city planners, public transportation authorities and routing planners and mapping services. Putting in place proper confidentiality agreements with its current customers, Zoba could capture value by offering the aforementioned players an updated and accurate aggregated demand data-platform that can overperform current mobility data specification programs implemented by cities.
Main challenges and risk mitigation alternatives
- Increasing consolidation of shared mobility players: particularly in the micro-mobility landscape, there is an increasing consolidation (driven by regulation scrutiny and market forces) trend that will end with 3-4 main players per each market. As players become larger and their business models more developed, it could be feasible for these players to build Zoba’s capabilities in-house. In order to mitigate this risk and increase the stickiness and robustness of the solution, Zoba could double down on its prediction and optimization capabilities by including additional variables related to other areas of its customer operations and by modelling, for example, the impact of Marketing or Product changes on customer behavior and demand.
- Local environments and volatile regulatory landscape: as the company starts to expand both in the U.S. and internationally, its solution will need to adapt its prediction capabilities to the particularities of each local market and the volatile regulatory landscape of each location. In this scheme, ad-hoc partnerships with local software providers and global contracts with major OEM’s and global mobility players could help Zoba expand its business in a sustainable and scalable way.
Sources
[1] Preeti Wadhwani and Prasenjit Saha, “Car Sharing Market Size By Model (P2P, Station-Based, Free-Floating), By Business Model (Round Trip, One Way), By Application (Business, Private), Industry Analysis Report, Regional Outlook, Application Potential, Price Trend, Competitive Market Share & Forecast, 2020 – 2026”, Global Market Insights, April, 2020 https://www.gminsights.com/industry-analysis/carsharing-market, accessed April 2020.
[2]Kersten Heineke, Benedikt Kloss, Darius Scurtu, and Florian Weig. “Sizing the Micromobility market”, McKinsey and Company, January, 2019, https://www.mckinsey.com/industries/automotive-and-assembly/our-insights/micromobilitys-15000-mile-checkup, accessed April 2020.
[3] Zoba, “Beware the heatmap: feedback loops in shared mobility”, Medium (blog), September 30, 2019, https://medium.com/@zobatech/beware-the-heatmap-feedback-loops-in-shared-mobility-9e675414dd73, accessed April 2020.
[4] Zoba, “Product”, https://www.zoba.com/product, accessed April 2020.
[5] Zoba, “Blog”, https://www.zoba.com/blog, accessed April 2020
[6] Megan Rose Dickey, “Zoba raises $3 million to help mobility companies predict demand”, TechCrunch, Feb. 2019, https://techcrunch.com/2019/02/21/zoba-raises-3-million-to-help-mobility-companies-predict-demand/, accessed April 2020.
[7] Frederick Daso, “Zoba, A Harvard Spatial Analytics Startup, Offers Logistics-As-A-Service To All”, Forbes, Feb. 2020, https://www.forbes.com/sites/frederickdaso/2020/02/04/zoba-a-harvard-spatial-analytics-startup-offers-logistics-as-a-service-to-all/#7fa9883a65d3, accessed April 2020.
Great article, thanks for sharing. It’s a fascinating business and one that will become more relevant as cities gradually become smart. I think you were spot on with the challenge of disintermediation as companies consolidate in markets. One of the topics I am curious about when it comes to any AI/ML platform is – who owns the data? Given how valuable data is to large enterprises, data platforms wrestle with customer reluctance to turn over company data even if it does improve margins. I wonder if Zoba experiences this friction, and if so, could finding ways to properly solve for data ownership disincentivize companies from disintermediating?
Thanks for the article. I agree that the company technology is very interesting and can be applied to much more than just micromobility/car-sharing space, the potential of which is quite limited given the small number of players in this space. Targeting transportation authorities is a great way for the government to utilize its resources more effectively and cut unnecessary spending.
Great article! I wonder if as the industry becomes more and more consolidated, and the ability to accurately forecasts demand becomes a more important competitive advantage, if Zoba’s customers start demanding any sort of exclusivity. Specially considering there might be questions as to the ownership of the data Zoba is using, companies may choose to use this to prevent its usage in services provided to competitors.