Visa and Big Data
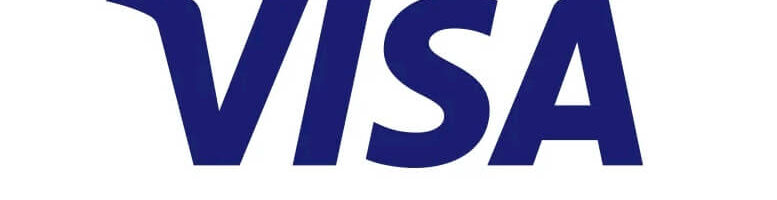
Visa uses machine learning tools and insights from big data to drive value for customers, banks, and merchants.
Visa Using Big Data and AI/ML to Prevent Fraud
Founded in 1958 by Bank of America as the BankAmericard credit card program, Visa, through a variety of mergers and an IPO, has grown to be the second largest digital payment company in the world. Visa does not issue credit cards or extend credit itself. Instead, it sells a variety of Visa branded payment products, such as domestic and international transaction processing and risk management, that financial institutions leverage to provide credit and debit services to customers. In 2021, Visa’s global payment network, VisaNet, processed 232.5 billion transactions, totaling $13 trillion1. For each of these transactions, banks, merchants, and consumers need to trust that the transaction has been made by the legitimate owner of the credit account and that the credit card user’s financial information is secure. This is a significant concern — credit card fraud accounted for 28% of the 1.4 million reports of identity theft in 20202. To meet this need for their customers, Visa collects vast amounts of data on individual consumer purchase habits and analyzes past and current trends to assess the likelihood that any given transaction is fraudulent. Visa’s ability to leverage big data as an asset to quickly and securely process transactions is at the core of how the company provides value to banks, merchants, and consumers, allowing them to grow to a 2022 market capitalization of over $384 billion.
Capturing and Creating Value With Data
Visa’s business has always relied on its ability to add value for customers by detecting and preventing fraud within the millions of transactions processed by VisaNet every day. Visa released real-time electronic authorization in 19833, allowing them to quickly verify canceled, past due, or reported stolen accounts at the point-of-sale. However, there were few other ways of verifying the transaction in real-time beyond that. This started to change in 1993 when Visa began to invest in and leverage early artificial intelligence and machine learning (AI/ML) algorithms to analyze trends in the large amount of consumer transaction data they captured and assess the likelihood of fraud in real-time. Visa has continued to invest in AI/ML throughout the decades, with fraud declining by two-thirds in that time4. Today, Visa uses sophisticated AI/ML software capable of analyzing hundreds of data points per transaction to assess fraud risk and identify potentially fraudulent transactions in milliseconds. With every purchase a consumer makes with their Visa credit card, Visa records data such as merchant location, transaction amount, time of day, length of the transaction, the location of a consumer’s mobile phone, and hundreds of other attributes. This data is compared to past consumer behavior and Visa’s software sends to the consumer’s bank a score indicating the likelihood that the purchase is legitimate. The bank can then leverage the insights provided by Visa to quickly accept or decline the transaction.
These increasingly sophisticated AI/ML models power many of Visa’s product offerings and allow them to add value for customers and consumers in new ways, such as with Smarter Stand-In Processing (STIP). Smarter STIP is designed to jump-in and leverage Visa’s AI/ML models to approve or decline transactions for a bank in the event that a bank’s network is down (e.g. due to natural disaster, bug, etc). In tests, Visa’s deep-learning AI model was 95% accurate5 in mimicking a bank’s decision on whether to accept or decline a transaction. This allows financial institutions to provide to consumers reliable and uninterrupted access to credit, providing value to both. Altogether, Visa reported in 2019 that their AI/ML models have helped banks prevent an estimated $25 billion in fraudulent charges6 annually.
Challenges and Opportunities on the Horizon
In the near term, I can see two major issues for Visa: maintaining their competitive advantage and increasing consumer awareness about data privacy. Both competitors such as MasterCard and tech companies such as Google or Amazon are investing in AI/ML to leverage their consumer data to drive insights and create value for customers. As AI/ML technology becomes more mature, the deep-learning algorithms that Visa has been investing in could become commodified and open them to either disruption or having their margins squeezed by both new entrants in the financial transactions space (such as Stripe) or long-time competitors. The second consideration is around consumers, tech companies (i.e. Apple), and governments becoming increasingly wary about data privacy. Visa’s fraud model depends on capturing large amounts of data about consumers. More stringent government regulations around the types of data companies can collect on consumers could negatively impact Visa’s business model.
References:
- Visa fact sheet. (n.d.). Retrieved October 4, 2022, from https://usa.visa.com/dam/VCOM/global/about-visa/documents/aboutvisafactsheet.pdf
- Mint. (2021, June 21). 25 credit card fraud statistics to know in 2021 + 5 steps for reporting fraud. MintLife Blog. Retrieved October 4, 2022, from https://mint.intuit.com/blog/planning/credit-card-fraud-statistics/
- The Evolution of Payment Security (n.d.). Retrieved October 4, 2022, from https://usa.visa.com/dam/VCOM/regional/na/us/visa-everywhere/documents/visa-security-timeline.pdf
- Rosenbush, S. (2013, March 11). Visa says big data identifies billions of dollars in fraud. The Wall Street Journal. Retrieved October 4, 2022, from https://www.wsj.com/articles/BL-CIOB-1691
- Castellanos, S. (2020, August 26). Visa unveils more powerful AI tool that approves or denies card transactions. The Wall Street Journal. Retrieved October 4, 2022, from https://www.wsj.com/articles/visa-unveils-more-powerful-ai-tool-that-approves-or-denies-card-transactions-11598436001
- Visa prevents approximately $25 billion in fraud using artificial intelligence. Meet Visa. A network working for everyone. Retrieved October 5, 2022, from https://usa.visa.com/about-visa/newsroom/press-releases.releaseId.16421.html
Visa Inc. (2019, June 17).
Thanks for the post, Julien — this was super interesting to learn about! The figures you’ve mentioned in here with regards to cost saving were really impressive themselves but what really surprised me was how early Visa was investing in this technology (1993)! I’d also love to do some personal research around what other data attributes are being taken into consideration by the fraud scoring system; back when I was an auditor, there were some seemingly random tests we had to do at the individual transaction level, which I found intriguing (e.g. a transaction that involves a 5+ figure amount that starts with “1” because a significant number of fraudulent transactions begin with the number “1” based on some psychological theory) — would be funny/interesting to see if Visa employs some of this too.
Thanks for this post Julien! I’ve always wanted to know more about exactly how banks employ AI/ML in such applications — I only had a vague picture in mind earlier.
It seems very impressive that banks are able to save a whopping $25billion in fraudulent charges using AI and that it is able to predict correctly with such high accuracy. While 95% accuracy in itself is impressive, squeezing out the last drop from 95% to 96% or 97% must even add tremendous value to banks at this scale.
I also wonder whether such algorithms are already employed for auditing. For example, do banks employ AI/ML to predict which accounts/ which entries are likely to be fudged up/ which accounts should be examined to increase likelihood of catching errors (to improve upon random checking).
— Aditya Mate