Vetr: Crowdsourcing Stock Ratings
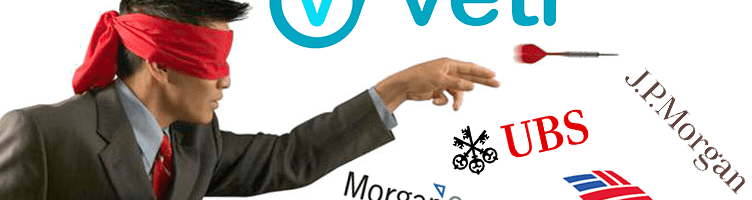
Can crowds do better than sophisticated analysts when it comes to predicting future stock prices?
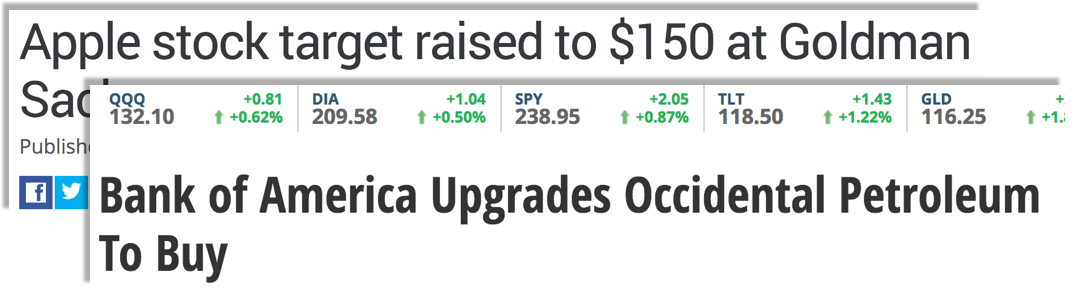
We’ve all seen headlines like this. For retail investors who don’t have access to management teams or sophisticated tools, ratings from professional analysts are often the best source of information as to whether a stock looks like a good bet. But how reliable are those reports, and whose interests do analysts have in mind when publishing ratings? Vetr hopes to offer a better solution by crowdsourcing stock ratings and educating retail investors along the way.
Equity research is famously complicated. Analysts with big name banks spend hundreds of hours poring over company financials, conversing with industry participants, and developing projections to produce what is essentially a ternary output: buy, hold, or sell. Research firms pay top dollar to recruit/retain analysts and sink millions into complex tools and databases (sell-side firms alone spent $15B on research efforts in 2016). Nevertheless, it’s not entirely clear whether that level of sophistication produces differential predictive power. Studies have shown top performing analysts demonstrate persistence in making profitable stock recommendations, but when it comes to target prices, only 24% to 45% of analysts’ forecasts are met. A retail investor deploying a trading strategy based on top analysts’ recommendations isn’t likely to make money. Moreover, the conflicts of interest that beset the sell-side research community are well documented.
Enter Vetr. The company’s stated mission is “to connect the world’s investors to make them more financially effective and successful.” Its key piece of technology is a predictive platform that leverages the insights of a multitude of users to generate ratings for individual stocks. Here’s how it works:
- Users make predictions about the future price of a stock
- Vetr aggregates user predictions then filters them through an algorithm to produce a target price and rating (based on five stars)
- Users’ forecasts are tracked over time, and top performers are rewarded with recognition on the platform
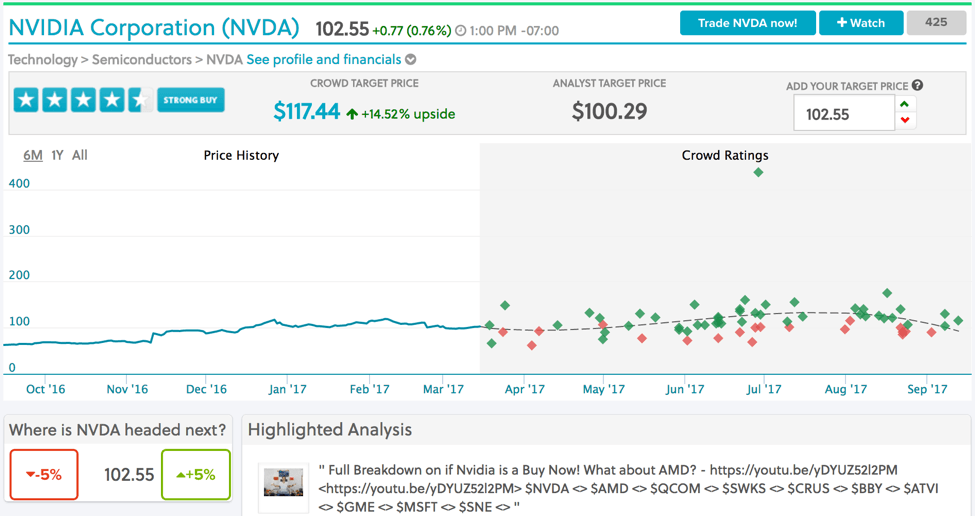
Vetr’s model has some advantages over professional research. It is 100% free, is unencumbered by conflicts of interest, and offers users an opportunity to educate themselves on investment analysis. Furthermore, Vetr’s crowdsourced price predictions could be more accurate than those of Wall Street analysts. According to the firm’s CEO, “our research demonstrates that Vetr’s aggregated predictions are more informative about future stock prices than any individual Wall Street analyst or the target price consensus of industry professionals only.”
Incentivizing widespread participation is difficult given the nature of stock picking. Quality stock analysts may not be willing to share their picks without compensation (e.g., in the form of cash prizes for performing well or some sort of subscription revenue sharing). This is highly problematic, as the idea that crowdsourced predictions can be more accurate than those of professionals depends largely on achieving a critical mass of quality predictors. The social recognition and portfolio building aspects of Vetr just may not be sufficient to attract enough users.
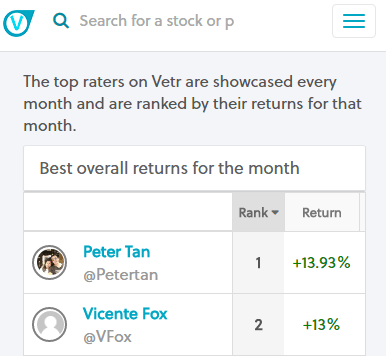
Sell-side analysts typically capture value in the form of one time fees, subscriptions, or “soft money” (i.e., providing research in exchange for brokerage business). Vetr’s aggregate predictions, if proven to be differentially accurate, could be extremely valuable, but capturing that value without destroying the community’s trust or the platform’s transparency would be challenging. Instead, the company is exploring four potential sources of revenue, including shifting to freemium and partnering with brokers to share fees on trades made by users.
Vetr may indeed have value as an education tool, but as a quality source of information for investors looking to build portfolios, it has some serious disadvantages. Most, if not all, of Vetr’s users lack access to management teams. For sell-side analysts, “private communication with management is a more useful input” than is primary research. In The Wisdom of Crowds, James Surowiecki recounts Francis Galton’s famous county fair anecdote: the average of individual guesses as to the weight of an ox was surprisingly accurate. In the case of equity analysis, Vetr’s crowd is competing against a handful of individuals with access to a scale.
This is an interesting new take on an old challenge. I agree with the shortcomings you lay out regarding traditional analysts’ accuracy and reliability, and crowdsourced evaluation of equities seems like an intriguing additional reference point for individual investors. Still, I am skeptical that this model would produce any “differential predictive power.” Stock prices are themselves set by the “crowd” of investors, so why would a subset of less-informed individuals be any more accurate than the overall average? Also, what incentive does an individual investor have to reveal their true view of intrinsic value? Seems to me like conflicts of interest persists even here…
Great post James.
I agree that this model does seem fraught with problems. I’m also very curious what the effects might be of systematic bias from companies. Because almost anyone can create these stock ratings, it would be relatively easy for publicly traded company to pose as analysts and post fake ratings, much the same way Yelp suffers from fake reviews by companies themselves. There may be simply too many misaligned incentives in this space to allow the crowds to work effectively. Combating this type of fake activity might end up being more expensive than simply producing verified stock ratings on a pay-per-rating basis.
Interesting post, James. Could be one step closer towards truly efficient markets. I wonder if the recommendations are truly unbiased though because wouldn’t retail investors who own an underlying stock be incentivized submit very high price targets to encourage more buying? Sort of reminds me of an Australian online forum called hotcopper, where people go to rave about the hottest stocks (which they often already own)
I am fascinated by this concept. Great post, James! One “secret sauce” that investment banks tout (and is probably unwarranted) is that they have direct access to management teams, which make their predictions more valuable. They are able to ask questions about future earnings directly to the company’s leaders on earnings calls. While I agree that the crowd can probably pick stocks better than an individual analyst, this does seem to be a competitive advantage that can’t be replicated. As you say, this model is problematic for several reasons, and I wonder if this additional complexity adds to that list.
Hi James,
Great post. Having worked on the buy-side, sell-side analysts and their estimates are often depended on to reflect the sentiment of the “street” (or the general ownership). I’m not sure that estimates from the crowd will really change this sentiment, thus, I don’t think there really is competition between Vetr and traditional sell-side research. In the best case scenario, Vetr will simply become a source of additional input into the buy side’s process!
Great Post James!! Vetr sounds like an exciting company! When you think about the financial markets in general, they actually are a crowd-sourced pricing model. As the great investor Benjamin Graham said “the market is a voting machine”. This means that the price of a security already reflects the opinion of the crowd – weighted by how much money they have put to use. Given this context, I think the most interesting part about Vetr is their ability to identify top-performers, and give them a higher weight. Vetr could outperform the market by being able to give more weight to historical top-performers when they make a prediction.