The Hunt for Lost Water
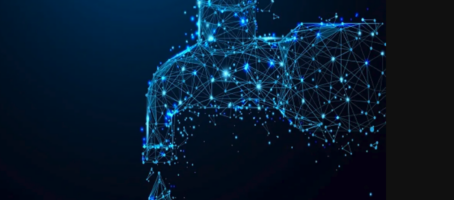
From data pipes to water pipes
One massive market that is realizing immediate gains from AI-based tools is the operations of municipal infrastructure. In the US, the aging networks of utility delivery has suffered from underinvestment and poor maintenance by under-resourced local governments, and this is especially true for the more than 2.2 million miles of underground pipes that comprise the country’s clean water infrastructure1. Non-revenue water (NRW), the volume of clean water that is lost annually due to leaks within the pipe system, is a significant burden on the overall system, accounting for anywhere from 20%2 to 50%3 of the total water produced for consumption.
DC Water, the government agency responsible for drinking water and sewage treatment in the Washington D.C. area4, has struggled with this problem for years, and they’ve recently turned to AI to adopt a more proactive approach towards combating water waste on two fronts
1. Shifting pipe monitoring to computer vision models
2. Predicting where leaks will occur
Computer vision monitoring
The modern approach of pipe monitoring involves a combination of strategically placed CCTV cameras, and using rugged, remote-controlled robots (“crawlers”) to traverse pipes as narrow as 4 inches5. Armed with a variety of sensors and cameras, crawlers can record images to be processed later. However, certified engineers are still required to manually review and tag each frame of the endless hours of grainy and dark pipe footage for potential defects.
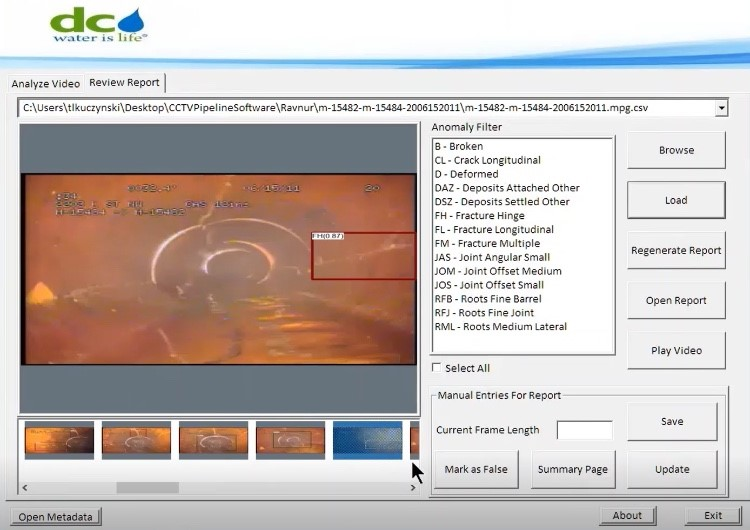
Source: DC Water
To automate this video processing, DC Water developed Pipe Sleuth, an AI-based pipe condition assessment tool. It uses “advanced image processing and deep neural network algorithms to identify, grade and score pipe anomalies based on the NASSCO PACP or UK WRC standards”6. It can handle up to 50 types of anomalies, and outputs interactive reports that are easy to manually review for edge cases. DC Water was able to leverage its extensive library of well labelled footage to quickly develop the initial use cases, and as more data is collected and curated, Pipe Sleuth could iteratively learn and deepen its anomaly detection expertise.
Predicting leaks:
Conventional wisdom states that “you can’t fix what’s not broken”, but that meant that DC Water was always on the back foot when it came to pipe repair, only able to respond after a breakage had occurred. However, AI has now allowed them to “proactively [address] and avoid those incidents, rather than being reactive when a problem occurs”7.
In 2019, DC Water partnered with the Microsoft AI ecosystem to develop a set of “predictive models for [evaluating] water main breaks and sewer flows, as well as pump performance and efficiency”7. By leveraging historical data on rainfall collection, customer complaints, and user consumption (including water-intensive businesses like laundromats), these models could preventatively identify key areas of risk in the pipe network. For example, if heavy rainfall in an area tended to cause 75+ old pipes near hotels to break, when the system detects new rainfall, it can preemptively alert as to which specific pipes are most at risk. And like all models, these tools only improve as they “learn” more about the idiosyncrasies of the DC water system.
Value capture:
The benefits of Pipe Sleuth are relatively straightforward: a dramatic reduction in cost of reviewing pipe footage (up to 75%6) and better accuracy due to the elimination of human error (80+%)6. Combined with the leak prediction tool, these projects enable DC Water to conserve water (~3M gallons/day8), and more effectively deploy resources towards repairing leaks, which increases the durability of the water pipe infrastructure, and more importantly, benefit the people of DC directly through reliable delivery of clean water.
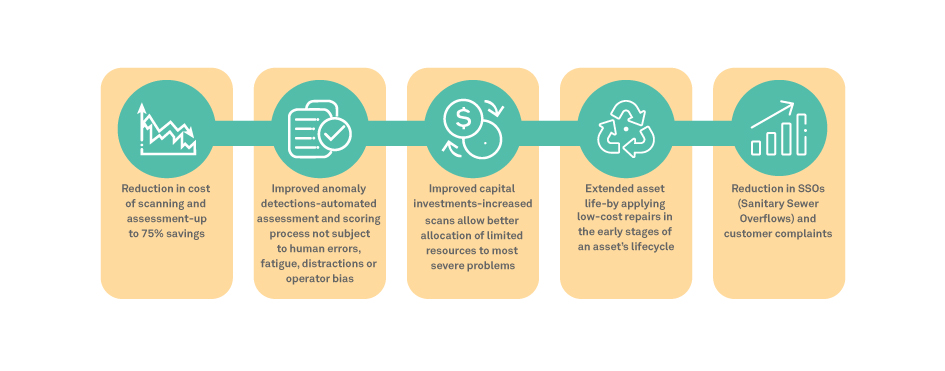
Source: Wipro
DC Water’s value capture is unique in two ways. First, unlike a typical for-profit venture, the end goal is not to maximize users or ads since they do not profit from customers directly. Instead, as a government agency, their mandate is to serve their community well, and the value of these AI tools is captured through higher constituency satisfaction, increased public health, and (tangentially) lower tax bills.
Secondly, rather than hoarding this innovation, DC Water has been actively working with partners to license out this technology so that other municipalities can adopt and benefit from these tools. In a cutthroat AI world where most firms are fighting to protect and commercialize their models, DC Water is trying to publicize and democratize theirs, proactively sharing their work to protect a key component of the national infrastructure, and empowering others to capture more value from DC Water’s work.
Organizational changes:
For a government agency, DC Water has done an admirable job in developing and integrating these AI tools into its culture.
To develop Pipe Sleuth, DC Water worked with Wipro, a tech consulting vendor, to create a proprietary solution over the course of ~2 years. The “predictive leaks” AI models were developed in close collaboration with a team at Microsoft’s Redmond headquarters. In both instances, rather than building the tech in-house with limited software engineering resources, DC Water leveraged more experienced technical partners, and instead focused on providing their water management expertise. This incredibly smart decision helped them get prototypes off the ground quickly, and built tremendous public goodwill for their partners.
Internally, DC Water integrated these AI tools by creating robust data pipes between the ML models and their internal management software systems (SCADA and PCS), customer systems, and GIS platform (to enable real-time spatial analysis)8. While not a small effort, DC Water believed it was important to use this data from day 1, so that the end products don’t sit siloed and unused.
Finally, DC Water made a concentrated effort to train their personnel to become more sophisticated in making use of these models. They started with simple exercises around automated usage meter tracking, before slowly incorporating more advanced data and models into the workflow. As Tom Kuczynski, DC Water’s CIO, admits, ramping up the team is not a single step, but a long-term investment, but he has noted that the team seems very receptive once they better understood the value that these new tools bring8.
Appendix:
- Drinking Water, American Society of Civil Engineers, 2021. https://infrastructurereportcard.org/cat-item/drinking-water-infrastructure/#:~:text=Overview,water%20to%20millions%20of%20people.
- Non-Revenue Water, Sensus. https://sensus.com/solutions/non-revenue-water/
- Up to 50% of Water Lost to Leaks in US – Here’s How We Stop It, Technology Networks. February 28, 2020 https://www.technologynetworks.com/applied-sciences/news/up-to-50-of-water-lost-to-leaks-in-us-heres-how-we-stop-it-331459
- District of Columbia Water and Sewer Authority, Wikiepdia. https://en.wikipedia.org/wiki/District_of_Columbia_Water_and_Sewer_Authority
- Robotic Crawlers, FiberScope. https://www.fiberscope.net/pipe-inspection-robot/#:~:text=crawlers%20or%20robots%3F-,Pipe%20inspection%20robots%2C%20also%20known%20as%20robotic%20pipe%20inspection%20cameras,to%20perform%20visual%20condition%20assessments.
- Pipe Sleuth, Wipro. https://www.wipro.com/engineering/pipe-sleuth/
- Goldstein, Phil. DC Water Taps AI and Cloud to Save Costs, Improve Infrastructure, State Tech. July 24, 2019. https://statetechmagazine.com/article/2019/07/dc-water-taps-ai-and-cloud-save-costs-improve-infrastructure#:~:text=DC%20Water%20has%20partnered%20with,sewer%20overflows%2C%20according%20to%20Kuczynski.
- Olavsrud, Thor. DC Water hunts lost water with analytics, CIO. Apr 19, 2021. https://www.cio.com/article/191612/dc-water-hunts-lost-water-with-analytics.html
Thanks David – this is a fascinating post! The question I have for you is whether you think this will become the standard, given the difficulties of working with governments. This could save tax payers billions but is government ready to join the AI revolution?
It’s a good question, and I think that resource constraints are actually going to be the best driver of AI adoption. As municipal government budgets get stretched, many of them will have no choice but to adopt tech as a way to do their jobs well without materially spending more.
This is a pragmatic and a cost effective approach to an already existing infrastructure where the future of this is IoT but implementing sensors and infrastructure is an expensive exercise and requires considerable investment in this space.The indirect benefits and the impact it has on an effective functioning of a society is quite glaring but with them investing in additional models I wonder how sustainable will this be ?
So if I understand your question correctly Melissa, many of the sensors, cameras, and robotics being used are already in place. What DC Water is using AI for here is simply the processing of the data that those cameras already collected. I do agree that IoT will become a powerful tool in scaling up infrastructure support, but I imagine it will be a slow and steady process, and likely largely motivated by shrinking budgets (which will require government to replace more expensive human labor with tech).