Re-imagining AR Management and Debt Collections
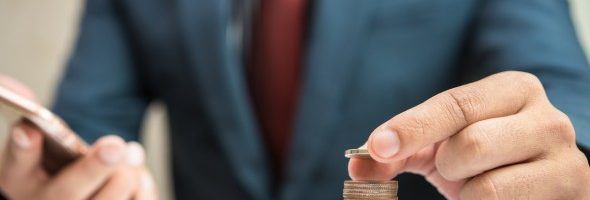
CollectAI and TrueAccord are only two examples of a novel business model: digitized debt collections. Once a labor-intensive, and very inefficient industry Accounts Receivable Management and Debt Collections have been transformed into a data-driven, fully automated service, as digital players are changing the game.
During the last few years, digital innovation applications have reached the services sector. New technologies are no longer limited to improve manufacturing and back-office processes, or to develop better products, but now they can also help companies with customer-facing activities to transform their operations while improving client interactions and delivering more efficient results. This is especially true for the finance functions at most of the companies, where research has found that “currently demonstrated technologies can fully automate 42% of finance activities and mostly automate a further 19%”.[1]
Transforming operations: from labor-intensive functions to digital assets
Traditional debt collections operations have been performed either by finance departments at operating companies (those which offer their products and/or services with some kind of credit terms), or by outsourced third-party experts – the so-called Debt Collections Agencies (DCAs). It is important to mention that the traditional operating model is highly labor-intensive, with wages accounting for 42.9% of total U.S. DCA industry revenue in 2017[2]. Moreover, in developing countries where even basic technologies are less ubiquitous, labor costs could add up to 65% of total revenues (!). Such a cost structure is explained basically because the traditional model was reliant on large teams of agents both analyzing the debt portfolio information and contacting debtors to negotiate debt terms and to collect payments. The two main functions performed by Collection Depts and DCAs were related to (i) exploit historical debt and payment data of current debt portfolio, in order to segment it and work on strategies to recover the outstanding debts; and (ii) serve as a contact center to engage with debtors, negotiate payment terms, and ultimately collect payments.
In contrast, disruptive digital players are offering an integrated, smart, fully automated, end-to-end service to handle their customers’ accounts receivables. For instance, in Europe, CollectAI is offering a cloud-based integrated solution that goes from e-invoicing services to machine learning powered debt collection services[3]. Similarly, in the U.S. market, TrueAccord is offering a “decision-making engine that uses machine learning to create personalized, digital first, consumer experiences (…), that can also predict the consumer’s reaction to communication frequency, timing, channel, and content”.[4]
The potential behind these solutions is that they can both increase the recovery rates and reduce the associated collection expenses.
Advantages of a digitized business model
– Labor Cost Savings. As per mentioned before, DCAs operate large analysts’ teams, that are very intensive in labor. By applying currently proved technology (RPA) to repetitive tasks such as segmentation, skip-tracing, queue prioritization, conciliation, and reporting, collection agencies could save between 20% and 30% of labor expenses[5]. If the same principle is applied for the contact centers, DCAs could not only gain FTEs efficiencies by automating customer contact with a rule-based omnichannel approach but also would mean lower costs related to staff hiring, training and especially turnover, which in the case of contact centers can be as much as 2x the U.S average.
– Improved Collection Rates. Besides freeing up huge loads in human resources, applying data-science capabilities to the segmentation and contact of debt portfolios can deliver great results. Currently, the most used methodologies to segment are driven by basic demographics variables and historical payment behaviors, but both tactics add limited value. Cutting-edge players are now using trained algorithms to learn about collection, pulling knowledge from previous successful cases and applying those insights to decide how to best approach the situations at hand. For example, TrueAccord’s autonomous machine-learning decision engine has already worked with (and learned from) more than 3 million debtors, offering them convenient payment plans, customized schedules, and easy documentation. There is no collection agent capable to handle that amount of information to make real-time decisions. In fact, data from real business cases show that machine learning capabilities allow TrueAccord to pull far ahead as data and decisions aggregate, outperforming traditional collection agencies by at least 30% and by as much as 500%, depending on asset class and customer segment (as shown in Figure 1).
Figure 1
– Better Customer Service. Automating the contact with customers ultimately lead to better customer reviews. Virtual agents (such as Google Duplex) are capable to connect seamlessly through different platforms (phone, email, text). They can also serve clients at unprecedented speeds without the need for waiting lines and with zero fatigue signals at the end of each day. Finally, by setting strict rules, companies can rest assured that all virtual contacts will comply 100% with current laws. These enhanced features not only make operations more efficient but also help optimize customer retention with higher satisfaction rates.[7]
– Enhanced Pricing Assessment. Another yet to be explored benefit from applying technology to collection processes is that companies driving higher predicting power on outstanding debt payments could then logically build an edge on pricing debt portfolios more accurately. Although the companies I have researched in this post have not openly admitted that they are active in the business of acquiring impaired debt portfolios, it is plausible to think that better pricing models would lead to a competitive advantage when bidding for delinquent debt portfolios. If true, this could represent a possibility to vertically integrate and become a ‘debt owner’, which would give companies an alternative source of revenue.
Most likely losers
In the long-run, my view is that traditional players in the collection industry will have to adapt or die. Current market leaders such as Encore Capital and Alorica would no longer be able to sustain their cost advantage when compared to non-labor-intensive players with enhanced technology skills. Currently, traditional DCAs managing large debt portfolios rely on economies of scale to serve customers at lower recovery prices. Many of them can do that by offering other contact center services and business processes outsourcing (BPO), while others are part of the debt portfolio acquisition business. Both the scale advantage from larger players and the cost advantage from ‘debt owners’ are at risk when compared to a smart, technology-oriented service such as the ones described above.
The other evident losers will be the collection agents, since at least their current services will no longer be required at the same magnitude levels. Contact center agents will probably lose their jobs to virtual agents for most of the interactions with clients, though some tough negotiations are expected to be still performed by human agents and supported by the data gathered by ML engines. Data analysts would be partially replaced for same ML algorithms; however, their experience will be critical in the machine training process.
[1] Frank Plaschke, Ishaan Seth, and Rob Whiteman. “Bots, algorithms, and the future of the finance function”, McKinsey & Co., January 2018.
[2] IBISWorld Industry Report – 56144 Debt Collection Agencies in the US, as of December 2017.
[3] “CollectAI – Our Solutions” – https://www.collect.ai/#solutions
[4] “True Accord – Product” – https://www.trueaccord.com/product
[5] Capitalizing on Robotics – Driving savings with digital labor, KPMG 2017.
[6] TrueAccord Performance Case Study. February 2018.
[7] CollectAI – Hanseatic Bank Business Case – https://www.collect.ai/press-release/collectai-improves-hanseatic-banks-receivables-management-with-a-24-percent-higher-collection-rate/
Gregorio, thanks for this insightful piece. I was impressed how much AI has been able to achieve in a field that was traditionally thought of as high-tough and therefore hard to automate. From reading your piece, I got the impression that the current solutions are using data analysis to figure out which borrowers are the “low hanging fruits” – debtors that are easier to deal with. I would assume there is a segment of borrowers with a low willingness to pay and thus require more human interaction. Perhaps there is an area where human workers are still needed? Could there be a model in which AI analyzes the borrowers and tells humans which borrowers to talk to and let humans handle the talking part? Taking it a step further, there could even be a scenario in which AI is advanced enough to come up with rough talking points or negotiation strategy. Human just need to add a slight human touch and deliver the talk.