Plantix: Bringing Crop Science Machine Learning to Millions
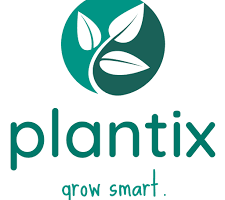
Plantix’s free mobile app helps millions of local farmers identify crop pests and diseases
Over the past decade, venture capital firms have pumped billions into machine-learning startups in the agriculture sector, investing $1.54 billion in 2021 alone. These ventures have promised to leverage machine learning to help agribusiness “control pests, eradicate weeds an increase crop yields” while using “fewer pesticides, fertilizers and other resources, including water.” Given the large and growing size of the global crop production market—estimated at nearly $5 trillion dollars in 2021—the potential payoffs are huge. Yet, while the market includes deep-pocketed multinational players, more than one-third of global food consumed is produced by over 600 million family farms, which have been largely ignored by these new VC-backed startups. Moreover, the UN Food & Agricultural Organization found that among these small farms “20% to 40% of all global crops are lost each year because of plant pests and diseases that aren’t managed properly.”
Origins of Plantix
Plantix was founded in 2015 by Rob Strey and Simone Strey, two crop science PhDs who wanted to develop a tool to help the hundreds of millions of farmers who needed help identifying crop diseases, but who could not afford to hire a professional agronomist. They believed that a machine learning tool could be effective in “high density farming” regions where it could leverage community-provided data to learn to identify local plant diseases over time. Plantix offered a free app that enabled farmers to take a picture with their smartphone, upload it, and receive a diagnosis of plant pests and diseases in real time.

The founders aspired to not only help farmers save their crops, but combat the growing problem of insecticide resistance. Local farmers often used insecticides or other chemicals when they suspected—but did not know—that a crop was impacted by a particular disease. But as with bacteria and antibiotics, insects could develop resistance to insecticides over time, meaning that “recklessly [using] chemicals results in both pollution and resistance.” Tushar Kamble’s experience was instructive. Kamble, a 38-year-old farmer in rural India was experiencing problems his chili plants, which were “smaller than average” and whose leaves were curling. He crowdsourced advice from neighboring farmers and got many different diagnoses, mostly consisting of various diseases. When he downloaded and tried Plantix, however, the app gave him a completely different answer: his chilis simply needed more water. The app also recommended a nutrient spray to purchase. Within weeks, his chili crops were thriving.
Building the Machine Learning Capabilities
Plantix started by focusing on a discrete set of 30 diseases common to the crop-producing parts of India where it intended to launch. The machine learning algorithms for each disease were built on a database of tens of thousands of images of plants. Each image was labeled by professional agronomists. The algorithm, in turn, had to perform various classifications. First, it needed to correctly identify the type of crop. Second, it had to recognize potential damage to the crop. Third, it had to classify any such damage as resulting from a disease (and not, for example, as resulting from inclement weather). Fourth, it had to correctly identify the particular disease. Finally, the app would recommend the best treatment options—not just chemical products, but also natural/biological products (e.g., rapeseed oil) and preventative measures (e.g., weeding and watering). The app also included a community forum feature, where other farmers could help one another diagnose plant diseases when Plantix did not provide a conclusive result.
Plantix leveraged machine learning across the app’s various features—not just the identification of diseases in plant photos. For example, the company trained a neural network to introduce a new feature that rejected poor quality photos and instructed the farmer on how to correctly re-take the photo (e.g., by point the camera closer to or farther from the plant). Another newly introduced feature leveraged machine learning to identify geographic trends in disease spread through photos uploaded by other farmers in the area. Every photo uploaded to the platform was “geo-time-tagged,” which enabled the app to track movements in pests and diseases. The app would then send a push notification to farmers in the area to warn them.

Several unique challenges emerged in classifying the photos uploaded by farmers through the Plantix app. First, poor signal or low battery could cause the phone to inaccurately geotag the image. Second, farmers sometimes took the photos of a crop outside the crop field (e.g., if they took the crop home). To address the former challenge, the Plantix team utilized the data from the Android and iOS operating systems, which both self-report a “confidence radius” when indicating the phone’s location. Photos that exceeded of confidence radius of 50m and which were outside a crop area were discarded. To address the latter challenge, the team developed a neutral network to “detect whether [a] submission came from inside a crop field using high-resolution [satellite] images” and labeled 3,000 such images to train the algorithm.
While it was hard to identify an overall accuracy figure across crop types and diseases, the company estimated that its algorithms correctly identified the disease status and particular disease of a crop upwards of 90% of the time. Some diseases algorithms were easier to develop than others. For instance, diseases with “shorter lifecycles” required as few as 400 pictures to train the algoritihim while other, more complex diseases required as many as 5,000. To date, the company had a database of 30 million images and an estimated 10 million annual users. Plantix’s image database grew at a rate of about 50,000 images daily. By 2022, it tracked about 500 crop diseases and was accessible in 18 languages. And in September 2022, it became the most downloaded app in the “Education” category in the Android Play Store in India.
Value Capture
The founders were committed to keeping the app free, yet by 2022, they had raised nearly $20m in VC funding. Instead, they sought to capture value in various other ways. First, the company “white-labelled” the software for sale to large agriculture and chemical companies. One such partnership was with Germany multinational BASF, which added the disease identification functionality into its “Maglis” crop management platform. Plantix also sold the software directly to large farms, charging fee for every “API call.” The company had further plans to develop predictive analytics capabilities that it could sell to other customers in the crop production ecosystem, including governments, insurers and input suppliers (e.g., seed producers).
Hi Joseph, thank you for your blog. The topic of machine learning and agriculture is novel, but very interesting to me, and it’s really inspiring to see the potential of machine learning that can be applied to the diagnosis of crop diseases. I was wondering about the challenges you mentioned regarding phone signal, location and image accuracy. Do they have a team of agriculture experts to help correct the image data for diagnosis, as we learned in the VideaHealth case before, on the human support side? Or do they rely entirely on algorithms?
What a neat topic! I am glad to see this in a more mature phase from what it was a few years ago. I had seen another example of this (https://blog.google/technology/ai/machine-learning-meets-african-agriculture/) when looking for a topic to do (glad I didn’t pick it and overlap with you!). It is especially interesting to read about the value capture portion. Thank you for posting!