Netflix knows what you like
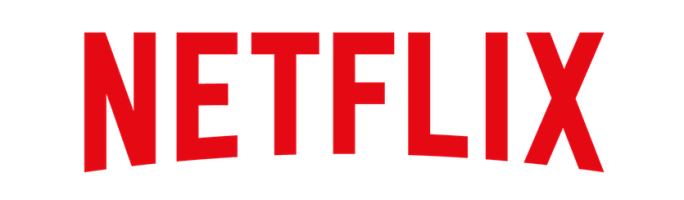
Netflix knows what you like and what you don’t like. Even as Netflix was sending DVDs to households across the U.S., it understood the importance of delivering the content that people would love. This logic has been extended to an extreme to develop an algorithm that recommends the shows you love and hides the rest, i.e. Netflix’s algorithm is boosting engagement and keeping you hooked.
Netflix is the world’s leading subscription video-on-demand streaming company in the world. With over 221 million paid subscriptions in over 190 countries watching millions of hours of content, Netflix generates massive amounts of data.
In the age of the attention economy, large technology companies constantly compete for users’ screen time across devices. This is why the effective distribution of relevant content is extremely relevant for business success at any media company. Large companies like Meta have invested billions in recommender systems designed to keep users engaged. Netflix has not only grown its library of visual content, but it has also developed the best librarian to help consumers find their next favorite show.
Netflix’s recommender system is critical for its success, given that the average user loses interest after 60 – 90 seconds of scrolling through titles, reviewing 10 – 20 titles. This means Netflix’s recommender system has less than a minute and a half to find something its users love, or it risks them abandoning the service. Internet users have numerous other things to do or watch without switching costs.
The recommender algorithms, known as the Personalized Video Ranker (PVR), serve as a key asset and define the Netflix user experience–literally. The homepage users see when opening the app is unique and tailored. The films and series displayed on about 40 rows with 75 videos per row are different every time Netflix is opened. For example, for some users, the Trending Now row might be shown before the Continue Watching row.
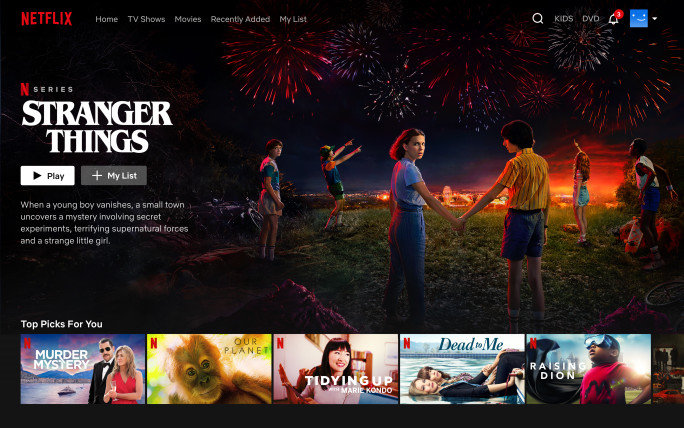
Netflix’s algorithms not only influence content position display. They also change which images are displayed for certain titles depending on the audience.
The algorithm Netflix uses is constantly trained and renewed by you. The algorithms rely on a mix of statistical and machine learning techniques, which can be supervised or unsupervised. This generates strong data-driven network effects that are extremely hard to replicate. The more a user interacts with Netflix, the more accurate the model becomes and the shorter the time to value (watching a film). This can be quantified as a business KPI in which the number of users that stop a session without watching content (churn) is minimized.
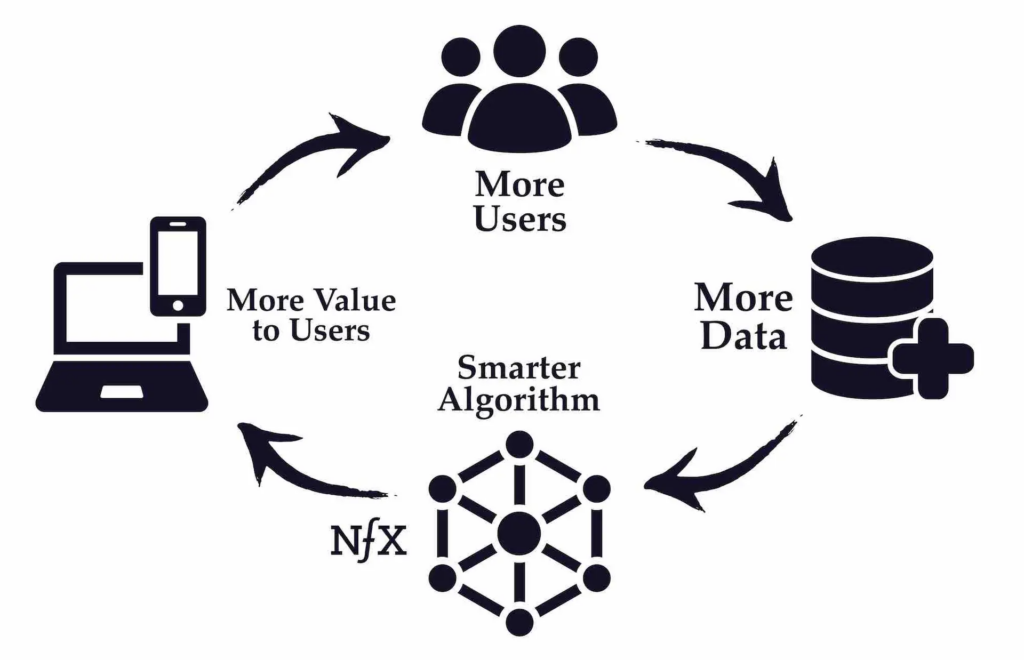
Furthermore, Netflix’s recommender system has a direct effect on media inventory. The ability to recommend and effectively distribute content to an audience who would have otherwise not been aware of is worth billions of dollars. The Effective Catalog Size (ECS) describes how distributed viewing is across a show catalogue. If all the viewing comes from one show, e.g. Stranger Things the number is 1, hence the lower the number the more distributed the viewers are across the content platform. The difference in ECS between a non-personalized recommendation and a personalized recommendation is 4x. In essence, Netflix gets a better investment return for distributing its content (recommender system) than for creating it and posting it on the platform.
Netflix had to overcome large challenges to create a recommender system, and in many ways, they are still just getting started. Even though a whopping 80% of content viewed is facilitated by the recommender system (20% search), customers often find the content they don’t like or nothing to watch at all. This highlights how difficult it is to create the perfect recommender system for all users. On the bright side, this is also a strong MOAT against newcomers like Disney Plus who don’t have the years’ worth of data necessary to create a comparable solution.
A recurring problem Netflix’s recommender system faces is recommendations for new users. The cold start problem starts when new subscribers start using Netflix for the first time, and the algorithm has no data to make inferences and develop relevant recommendations. Netflix tries to minimize the risk of poor first impressions/recommendations by asking users to select a few titles they like and literary jumpstart the recommender system.
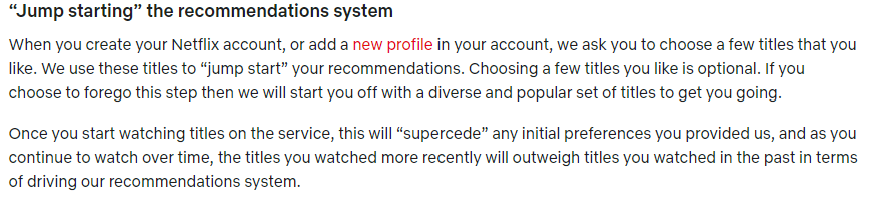
In the last months, Netflix headlines have been plagued by doom and gloom due to subscriber loss and well-funded competition from Amazon and Disney. The so-called content wars are threatening to make the whole sector unprofitable. This is a clear opportunity for Netflix’s recommender system, as it is difficult to replicate a MOAT that is 4x more important than the amount of content a platform has.
Finally, what does your Netflix home page look like? Post it in the comments.
Sources:
Very interesting, Daniel! I didn’t know Netflix would even change the display pictures for the same movies recommended, how detailed! I wonder what KPIs they use to measure the effectiveness of recommendations and reinforce/reward the recommendation mechanism (in addition to churn?). Also, would they design anything in the recommendation system to prevent user addiction/too long watch time?
Daniel, thank you for sharing this interesting post!
I can totally see the work Netflix has put in the last few years to collect more data about the user and manage to provide better content recommendations. A few years ago I considered cancelling my subscription because it took me so much time finding something I liked, while nowadays I have so many movies waiting in my library for me to watch during the weekend. Even though you can say Netflix today is wiser in terms of providing a better analysis about the customers’ preferences, Netflix is dealing with new challenges regarding the number of competitors that see a big opportunity in this space to capture more viewers. I wonder if Netflix will be able to maintain profitability and growth in the future years, maybe their secret formula is not a secret anymore and other companies such as Disney Plus will double down on efforts to be the top choice of consumers, only time will tell…