Lenddo: using data analytics to boost financial inclusion
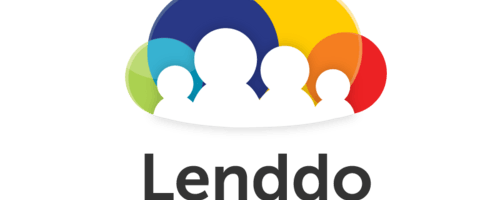
Can social data and online behavior predict an individual’s propensity to default on a loan?
The challenge
Access to financial services is a key element for reducing poverty and boosting economic prosperity. There are approximately 2 billion people and 200 million small and micro enterprises in emerging economies that lack access to basic financial services such as savings and credit services. Even in developing nations with high degrees of financial inclusion such as Malaysia where 92% of adults have bank accounts, there is a mismatch between the financing needs of the population and the financial services available to them. The result is that individuals and SMEs often end up paying extremely high fees for services that do not adequately address their needs.
Even though there has been some progress over the past few years, millions of lower and middle-class citizens in emerging economies are affected given the chicken and egg dynamic of the traditional financial system. Individuals have the steady income to take on a loan but they lack the credit history to get approval from financial institutions. Structural issues such as lack of infrastructure and information, weak credit score bureaus, inefficient institutions, among others complicate the situation further.
Lenddo
The traditional credit assessment process relies on evaluating the borrower’s capacity to pay. This is burdensome and costly for both the lender and the borrower, especially in the case of smaller loan sizes. Lenddo is taking a different approach by collecting both traditional and non-traditional data and using artificial intelligence to predict an individual’s likelihood to pay.
Lenddo does not actually provide financial services directly to individuals. It enables financial institutions to do predictive analytics to service new client segments in a profitable and scalable manner.
How does the process work?
- Applicants submit their applications – the application consists of a simple form with basic personal information (name, date of birth, email address, mobile number, employer and education), a psychometric test and granting data access permission to Lenddo.
- Data collection – Lenddo collects applicant data from traditional sources such as credit bureau data and financial transactions (if available) and non-traditional data from the following sources:
- Psychometric tests
- Telecoms
- Browser history
- Mobile data (i.e. geolocation)
- Social networks
- E-commerce transactions
- Data Analysis – Using advanced machine learning techniques, Lenddo’s predictive algorithms look at 12,000 variables and come up with a credit score. The credit score (called the LenddoScore) ranges from 0 to 1000, with higher scores representing a lower likelihood of default.
- Decision-making – Financial institutions can use the LenddoScore to help them differentiate good from bad borrowers and approve or deny loans. Financial institutions using LenddoScore have, on average, increased their approval rates by 50% and decreased their default rates by 12%.
Value Creation
First, Lenddo is allowing its clients to target a segment of the population they were previously unable to serve because the loan economics do not work under the traditional operating model. Credit assessment accuracy and efficiency have dramatically increased as it only takes the algorithms 3 minutes to come up with a credit score and the process requires no human intervention, resulting in high operating leverage.
Second, Lenddo can update its credit assessment for an individual in real-time. If specific events occur in an individual’s life that affect the likelihood of default, banks can proactively take action and manage the risk/return profile of the loan portfolio
Third, Lenddo allow banks to gain insights into the consumer’s financial needs by analyzing their online behavior. Banks can take advantage of these tools to adequately match financial services to individuals and innovate on their product offerings.
Finally, individuals get access to the financial services they actually need without having a credit history. Additionally, a virtuous cycle is created because there is a better match between the loan characteristics and the individual’s financial needs making them less likely to default, which in turn improves their credit worthiness.
Risks
While the advent of data analytics and technology seem to be a promising solution to the challenge of financial inclusion, it comes with considerable risks. These include issues such as fraud, data privacy and security, discrimination and insolvency (the latter is mostly applicable to startups). Regulatory agencies must play a significant role to ensure that individuals are protected from predatory practices while at the same time not hampering much needed innovation in the space.
Sources
- The Global Findex Database 2014: Measuring Financial Inclusion around the World, World Bank – Asli Demirguc-Kunt, Leora Klapper, Dorothe Singer, and Peter Van Oudheusden. April 2015.
- Revolutionizing Microfinance: Insights from the 2017 Global Symposium on Microfinance. World Bank Group. May 22nd, 2017.
- https://www.lenddo.com/products.html#creditscore
- https://www.lenddo.com/about.html
Interesting post, SAG! I am curious about the sustainability of Lenddo’s value creation. Financial institutions are rapidly digitizing and getting their hands dirty in data. Similarly, many online lending companies are gaining traction that use similar metrics to measure someone’s creditworthiness. What is to prevent the institutions Lenddo is serving from building their own capabilities or subscribing to an online lending platform?
Thanks for the post SAG. I am really fascinated by these models particularly in the context of emerging markets where many people are unbanked and have no formal credit histories. I am however, curious to understand the consumer’s perspective. While Lenddo definitely provides access to much-needed financial services, it also seems incredibly intrusive. In this age of data breaches and privacy concerns, how comfortable are consumers knowing that you are dynamically scouring through their ecommerce transactions, browsing history and social media? Further, how is that information being used? Given the dynamism of the decisions, I would worry how posts I am making on Facebook for example, are affecting my credit score so I wonder how Lenddo is educating consumers about this to manage their potential skepticism (if they are anything like me of course).