John Deere: Killing weeds precisely with See & Spray technology
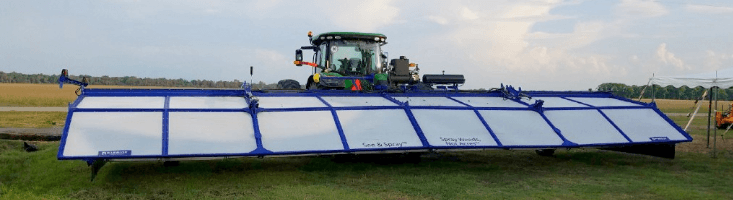
John Deere is trying to change the farming industry by adopting computer vision and machine learning technology.
Agriculture is the oldest and yet most fundamental industry in human history. Especially, the farming industry holds the very bottom of the food-chain and supplies a tremendous amount of food for the ever-growing population of our planet. Even though it is inarguably important for people’s life (we are feeling it even more seriously in this crisis), technology development of farming receives less attention than it should. Productivity and efficiency of farming have been largely driven by decades-old heavy machines and chemicals. Recently, biochemicals have provided significant improvement to the yield, but food safety of those technologies is still a big concern. And now, AI and ML are about to change the game.
John Deere, the 180-year-old manufacturer of farming and industrial machinery, is developing advanced equipment that will reduce 80-90% of herbicide usage by leveraging vision processing and machine learning technology. What this machine does is largely two parts; See & Spray.
(Exhibit A)
(1) See
The machine (shown in Exhibit A) equipped two rows of cameras on its arm. The front cameras use computer vision and machine learning to make on-the-spot judgment calls whether a plant is a weed or not, while the rear cameras calibrate the systems for adjustments on the fly. The machine processes images of plants more than 20 times per second while traveling 12 mph through the field, comparing them to an expanding training library of over 1,000,000 images. After detecting, the machine determines the appropriate treatment for each plant. John Deere has developed intelligent models using computer vision and machine learning that can distinguish subtle differences between cotton plants and weeds of many species and sizes. (shown in Exhibit A) This vision processing and machine learning eliminate human error and save tremendous time for farmers.
(2) Spray
Upon identifying weeds, the machine’s robotic nozzles automatically target and spray herbicide on the weeds. The company says that the machine applies herbicide only to weeds, avoiding chemical application on the cotton crop or on areas without weeds. The precise application allows farmers to reduce chemical usage by an order of magnitude and unlocks the ability to use herbicide alternatives to effectively control weeds that would otherwise be resistant. John Deere also developed a new sprayer nozzle technology designed to respond instantly to changes in the wind to reduce drift by modulating droplet size.
Transitioning into AI and data-focused company
John Deere had a vision of transforming the farming industry with leading-edge technologies such as AI and data analytics early on. In 2013, it unveiled its Farm Forward vision – demonstrating the concept of the “autonomous farm” where machinery would be remotely managed from a central control hub. Along with the effort of digital transformation, John Deere acquired Silicon Valley startup Blue River Technology at $305 million in 2017. Blue River Tech, founded in 2011, was the startup that developed “See & Spray” technology.
Value creation for farmers and beyond
One of many concerns for not only farmers but also environmentalists and people, in general, is the growing usage of the herbicide. Existing weed control methods such as broadcast-spraying of herbicides result in 3 billion pounds of herbicides being used each year costing a total of $25 billion. Over 90% of cotton planted acres in the US have herbicide applied. Moreover, over-reliance on a handful of chemicals is fueling the herbicide resistance of weeds, making traditional broadcast-sprays less effective at weed control.
By adopting new technology, John Deere explained, farmers can reduce herbicide usage by 80-90% while enhancing productivity and yield. This technology will certainly boost farmers’ economics in the long-run and, at the same time, reduce environmental damage.
Challenges ahead
One big challenge is the adoption of technology and machine. While the machine will help farmers save money on herbicides and pesticides, the machine may require a significant upfront investment, including machinery purchase and infrastructure. In addition, the common stereotypical portrayal of a farmer is that they are not tech-savvy and open to innovation. While this stereotype may not be true, John Deere should look for early adopters in the industry and showcase the efficacy and value of its products to the broader customer segment.
The second challenge is the unpredictability of the farming environment. Because agriculture is largely an outdoor application, there’s no enclosed, central onsite facility where one can implement a server and a communications network. To overcome the shortcoming, John Deere implemented edge computing, with processing onboard the machines doing the work. However, edge computing and network have to be customized for each different farming environment, slowing the adoption and scaling. Since data collection on multiple parameters including the image of weeds, weather conditions, soil conditions, not only for the current product but also for future development, John Deere should select representative areas for unique conditions and experiment the product extensively to acquire data. As a result, John Deere would be able to accelerate the customization process and scale faster.
<Sources>
https://www.deere.com/en/index.html
https://www.agweb.com/article/deere-shows-its-tech-side-consumer-electronics-show
https://www.fierceelectronics.com/sensors/ai-meets-farmer-helping-make-each-plant-count
https://www.agprofessional.com/article/blue-river-expands-see-spray-testing-commercial-launch
Thanks for sharing! This was a really interesting blog post–I had no idea that John Deere was leveraging AI to improve farming. You rightfully pointed out that the upfront investment in such expensive machinery could be a blocker from larger adoption. I wonder if John Deere will consider “leasing” the equipment on a monthly or quarterly basis to farmers. While not a direct comparison, I think of the road block Peloton faced with an expensive bike they were trying to sell to consumers. They offered a monthly payment options which made the purchase much more palatable to their target audience. This also ties to the Afterpay case we discussed in class.
Depending on the proximity of farms in a given area and the mobility of the product, there also could be an option of group-buying where a few farms would share costs to own the equipment and take turns using it.