Is FICO outdated? ZestFinance: An Alternative to the FICO Credit Score
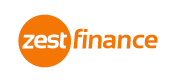
In 2010, roughly 8.3% of US consumers, or 19 million people, were considered ‘unscorable’ by FICO, a credit rating service whose models serve as a key underpinning of the US system for assessing credit worthiness.[1] Included in this contingent of unscorable consumers are those lacking a credit history, read: millennials, who represent an untapped market opportunity for lenders. Also excluded from FICO are the underbanked, those who lack bank accounts and primarily transact in cash. Not only are the underbanked denied access to loans based on typical FICO filters, but they must resort to predatory alternatives, such as payday loans, whose prohibitively high interest rates trap them in a vicious cycle of deep indebtedness that is difficult to dig out of. Has the FICO score become an obsolete filter? And can a lender step in to provide loans to these underserved segments where traditional banks have neglected to do so – and profitably?
In 2010, roughly 8.3% of US consumers, or 19 million people, were considered ‘unscorable’ by FICO, a credit rating service whose models serve as a key underpinning of the US system for assessing credit worthiness.[1] Included in this contingent of unscorable consumers are those lacking a credit history, read: millennials, who represent an untapped market opportunity for lenders. Also excluded from FICO are the underbanked, those who lack bank accounts and primarily transact in cash. Not only are the underbanked denied access to loans based on typical FICO filters, but they must resort to predatory alternatives, such as payday loans, whose prohibitively high interest rates trap them in a vicious cycle of deep indebtedness that is difficult to dig out of. Has the FICO score become an obsolete filter? And can a lender step in to provide loans to these underserved segments where traditional banks have neglected to do so – and profitably?
ZestFinance believes so, and has leveraged machine learning to develop a credit scoring engine for borrowers who lack the available credit history necessary for a suitable FICO score, if they have one at all. By assessing whether an individual should receive a loan and directing the applicant to a bank partner, ZestFinance addresses a market failure where underserved segments couldn’t access needed loans at interest rates they could afford – and that banks could afford to service.
ZestFinance offers two services to address this need. First, it licenses its credit-scoring services to subprime lenders with loans averaging around $600 with an APR of 390% — compare this to an average 521% for payday loans. [2] Second, the company has begun originating its own loans, called Basix, in the near prime space for consumers with FICO scores below 680. These loans aren’t cheap either, with a fixed annual interest rate of 26 to 36% over 3 years for loan sizes ranging from $3,000 to $5,000. [3]
The company achieves this value creation through core assets of 1) an experienced data science team with niche expertise in feature engineering, specifically for credit scoring and 2) a rich dataset of alternative credit assessment variables and loan performance outcomes. The latest algorithmic techniques are applied to a dataset of some 70,000 variables derived in part from 10 alternative credit bureaus that payday lenders report to.[4] Interestingly, a loan applicant’s use of all capital letters on a loan application has been found to correlate with higher risk.[5] ZestFinance’s accumulated dataset of the loans it has underwritten and associated loan performance can then be used to continuously hone and retrain its models.
Just how good are its models? Their subprime loans have achieved a 15% default rate, 50% the average figure for typical payday lenders.[6] The company’s success has attracted the attention of Baidu and JD.com, which have enlisted its talents to develop a credit score for Chinese consumers based on alternate variables such as search history.[7]
ZestFinance captures value through license deals with lenders and loan margin on its near prime loans, but one could see how these revenue streams could erode as the space becomes increasingly crowded and as policy further develops to protect subprime customers from interest rates beyond a certain threshold. Additionally, we have yet to really stress test these loans. It will be interesting to see how loan performance shakes out in the event of a downturn, especially in the case of their Basix loans, which have yet to realize a full 3-year term.
So what’s next?
ZestFinance competes in a crowded space, and key to its long-term defensibility will be its development of a truly proprietary dataset and ancillary services to support its data feedback loop. Joined by Prosper, Elevate Credit, Avant, and LendUp, just to name a few, ZestFinance’s claim to sophisticated human judgment in model building and valuable data are core competencies cited by all competitors. Its access to China’s wider diversity of model inputs positions it to develop a more unique dataset in the short term, but this could erode in the future.
Likely aware of this, ZestFinance has expanded beyond loan underwriting, and is now offering a new service called ZAML, which is a platform lenders can use to build their own credit-scoring models.[8] Clients can input their own data sources, train models, and understand model outputs in economic terms through ZAML.[9]
ZestFinance’s value creation story now has changed to involve value sharing across various players in the ecosystem. Best practices with data sources and modeling techniques can be potentially leveraged across ZAML’s entire client base to improve credit scoring practices – and with significantly less investment from each individual firm. ZAML could also be used to ensure model compliance with federal regulations, such as the CFPB’s mandate that lenders may not discriminate against certain demographics in their loan approval procedures. [10] Value capture now takes the form of recurring SaaS revenue for ZestFinance. Key questions going forward will be whether the company can sustain a recurring need for its ZAML platform – are models built once and rarely retrained—and whether there is an opportunity to horizontally extend its services across industries.
[1] http://www.tradestreaming.com/2016/04/19/5-surprising-things-you-might-not-know-about-fico/
[2] https://www.washingtonpost.com/business/zestfinance-issues-small-high-rate-loans-uses-big-data-to-weed-out-deadbeats/2014/10/10/e34986b6-4d71-11e4-aa5e-7153e466a02d_story.html?utm_term=.b45ddc768f13
[3] https://www.forbes.com/sites/laurashin/2015/10/06/alternative-lender-zestfinance-raises-150-million-in-funding-from-fortress/#2207479c1f97
[4] https://www.americanbanker.com/news/zestfinance-aims-to-fix-underwriting-for-the-underbanked
[5] https://www.washingtonpost.com/business/zestfinance-issues-small-high-rate-loans-uses-big-data-to-weed-out-deadbeats/2014/10/10/e34986b6-4d71-11e4-aa5e-7153e466a02d_story.html?utm_term=.b45ddc768f13
[6] https://www.washingtonpost.com/business/zestfinance-issues-small-high-rate-loans-uses-big-data-to-weed-out-deadbeats/2014/10/10/e34986b6-4d71-11e4-aa5e-7153e466a02d_story.html?utm_term=.b45ddc768f13
[7] https://techcrunch.com/2016/07/17/baidu-invests-in-zestfinance-to-develop-search-powered-credit-scoring-for-china/
[8] Website
[9] Website
[10] https://www.consumerfinance.gov/fair-lending/
Great post, AC. I also wrote my post about a credit scoring company (Friendly Score), and have a question for you about the institutional context in which these companies operate. In the US, there are strict laws against lending practices that discriminate – whether intentionally or not – along certain demographic criteria (ethnic, gender, etc.). Do you think that what ZestFinance is doing rubs up against these laws in any way?
Great question. ZestFinance closely monitors their models to ensure they aren’t systematically discriminating against certain classes. That said, it’s unclear to me how payday lenders ultimately defend themselves here as the demographics of their accepted applicants are likely quite homogeneous.
AC, thanks for the post! The capital letters thing kinda intuitively makes sense haha. I’m curious as to what some of these other alternative variables are.
I always fill out forms in allcaps because I have terrible handwriting. Not sure how I’ve gotten this far in life.
and what about all the federal forms that tell me to write in allcaps!!?
RIGHT?! Many of the other variables are ones you’d expect: income, zip code, default history, etc.
Nice post Amy. I’m very much interested in what the core alternative credit assessment variables are, and how ZestFinance team interpretate the correlations between them and loan performance outcomes. Also, I wonder if more data collected in the China market will help ZestFinance to predict risks in the US market, i.e. how transferable data and according algorithm is across markets.
Thanks, Yao. I imagine many of the variables are quite transferable, ie income, hours of the day searches are conducted. My understanding is that they cannot take their China data out of China, so that likely limits their analysis in a cross-border context.
So happy you wrote a post about Zest! I think they are a phenomenal team with promising model. Quick update on the business model, they are looking at a significant pivot to B2B with ZAML and I won’t be surprised if they start winding down their balance sheet of Basix loans (yup, they keep it on their balance sheet).
Regarding anti-discriminatory credit compliance, they won’t use variables such as gender, race, etc as direct input but how far they are at making sure the other variables are not significantly correlated with protected class is unknown.
Firms that rely on alternative underwriting keep their models close to their chest – the only way we can perhaps tell is looking at their team’s credentials. Firms that have Palantir teams or backed by Peter Thiel, Jon Lonsdale, Formation 8 or Google are worth a longer look than other in my opinion.
Yep, commented briefly on the ZAML pivot at the end of the post and I agree 100% on the challenges of the existing model — payday lending in general is just not a great place to be in given inevitable regulatory uncertainty. Key will be understanding if ZAML finds a recurring need among its customers.
Interesting story! I looked on the website and still did not figure out what kind of data is used for the mysterious machine learning. Is there any information on this? I do believe in the power of machine learning, but if data input is opaque, I would be more critical of the validity and sustainability of the solution.
They definitely keep their model details close to their chest as that has been defining their competitive advantage to date. Over time, the data they collect on their loan performance will create greater value, but given the recency of their loan issuances, many have yet to complete a full term’s worth of data.
Thanks for writing about ZestFinance!
In many ways, the current credit scoring system is broken, overly relying on previously requiring credit and paying it off rather than looking at the borrower as a financial entity that could be credit worthy while not previously requiring credit. Take, for example, internationals moving to the US in adulthood (i.e. graduate students, work immigrants, etc.) that may have had full fledged financial history in their home countries, may hold considerable assets, but are considered not credit worthy in the US.
Furthermore, the notion that your credit score is mostly based on you requiring credit in the past is weird, what if you were making enough to not need to borrow?
Alternative ways of looking at this issue, especially driven on new data sets and different perspectives are bound to make a huge difference in this space.
Spot on, Gil! The need is significant here, and to your point, I’m especially interested to see how their loans perform in the event of another recession (hopefully not anytime soon). This will show us how effective their models really are.