Is Dall-E really improving?

Analyzing evolution from Dall-E Mini to Dall-E 2
I was curious how text-to-image converter AI has improved ever since the release of Dall-E in January 2021. This was followed by Dall-E 2 release in April 2022.
Text: Zebra Unicorn in Space
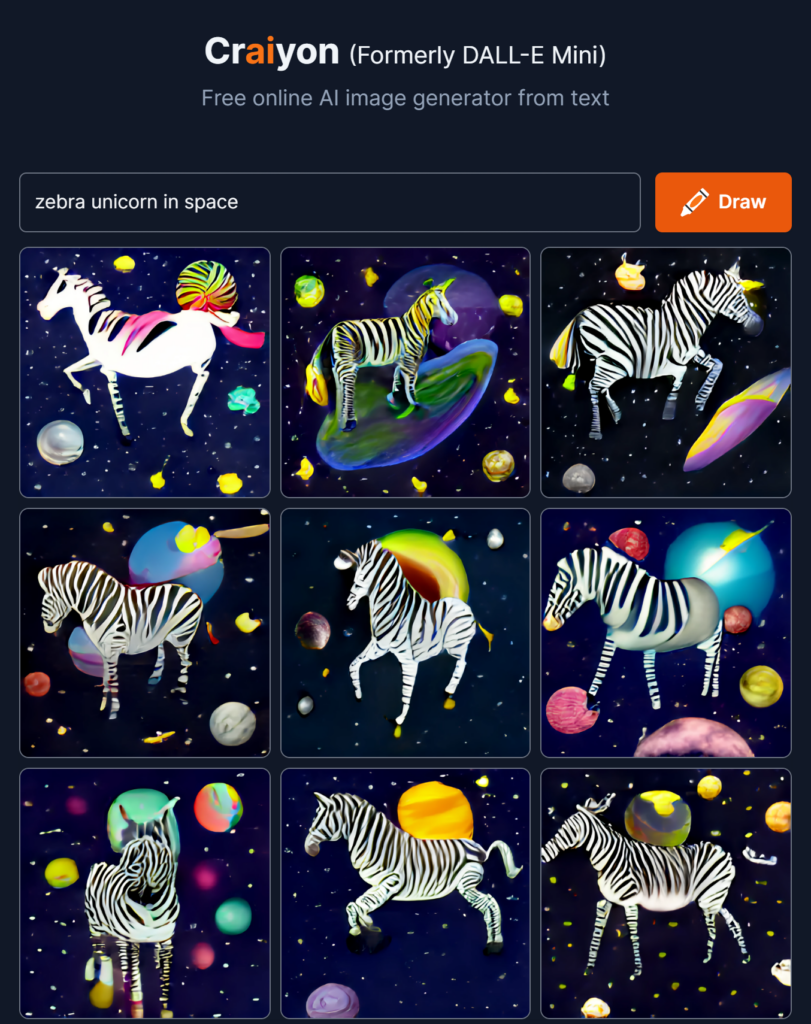
Below’s the one from Dall-E 2
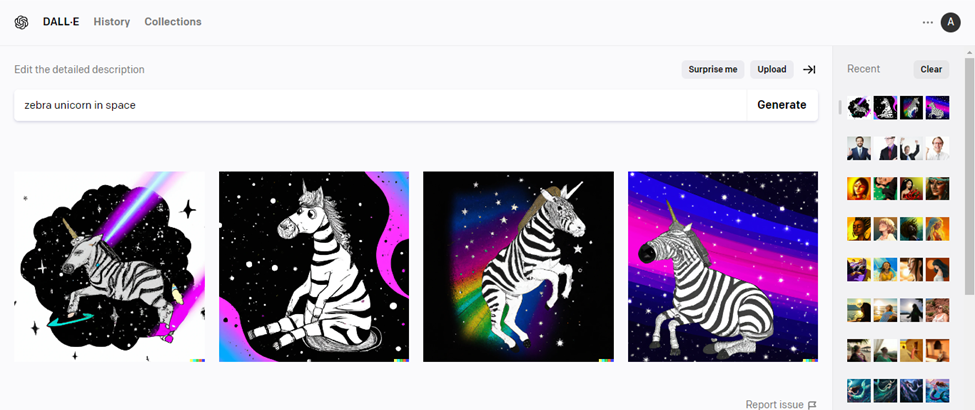
As seen clearly, the Crayon only picks up cues of ‘Zebra’ and ‘Space’ but misses the nuance of ‘Unicorn’. Also, the face is blurred and Zebra is mostly in a running/standing position. Whereas, in Dall-E 2, we have a clear face with the Zebra (and a unicorn) in different positions.
This curiosity led me to explore if the advances in OpenAI’s models have also improved upon algorithmic bias, only to end up disappointed!
Text: Successful Professional
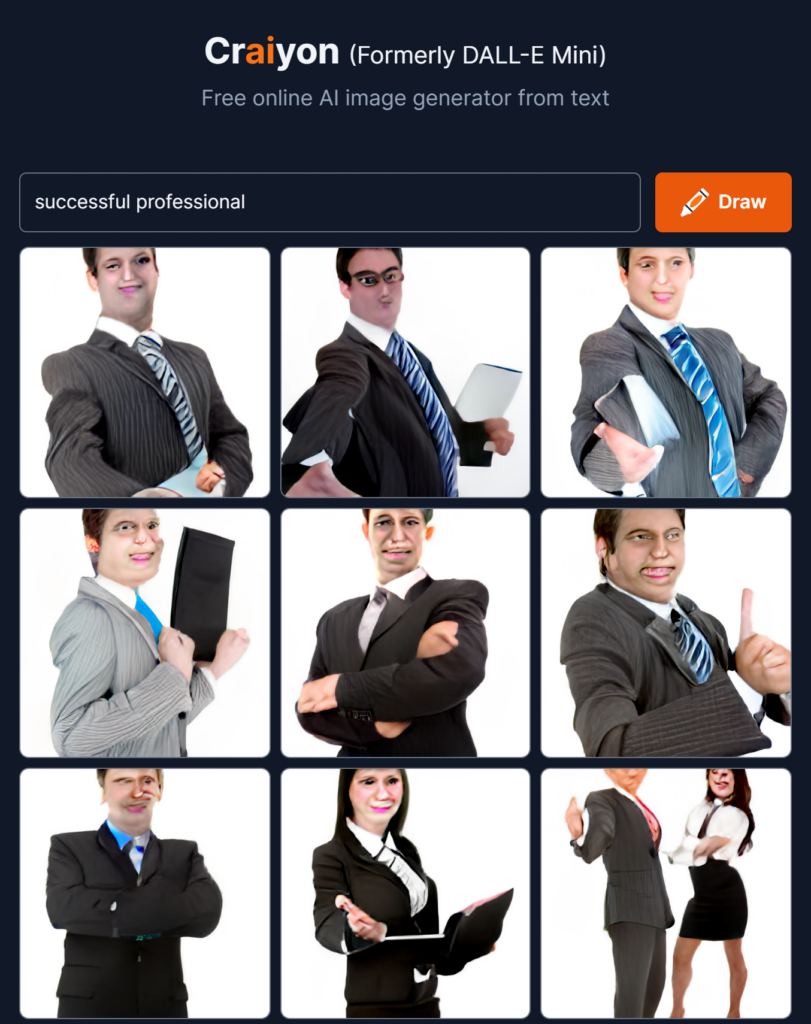
Clearly, all light-skinned people and mostly, males! Dall-E 2 was even worse:

Thank you so much for this post!
It is interesting to see how the AI defines these inputs. It seems like the AI does not have picked up diversity and variety. This might be based on the training set – but I wonder if this can still be changed now or if it is already too late. In that sense the question comes up at what point it is too late to change a bias in an AI or if one has to start from the beginning again.
Thanks Anand! Really interesting how different the Dall-E product is compared to the Craiyon product. I also think it is extremely valuable to point out the biases that are built into these AI programs. Your blog post makes me wonder how we can improve the algorithms to eliminate these biases.
Interesting difference between DALL-E and Craiyon. I’m curious if DALL-E is pulling stock images of humans or if it does just have better data. The difference in human feature recognition is pretty stark between the two programs.
So interesting! I also did a comparison between Dall-E 2 and Craiyon but our observations were slightly different. The data inclusiveness angle is very relevant and thought-provoking
That’s amazing on the quality difference between Craiyon and Dall-E 2 rendering people! My attempt provided quite disappointing human representations (though I give them the benefit of the doubt it being an expensive “free” resource), but Dall-E 2 seems pretty much stock photos to me — I wonder if it’s able to capture other nuance in the statement (like reading papers while drinking coffee) so that it’s not just plugging in a stock image and has to draw something different.
Interesting enough that my result for Craiyon also included women and I would be curious if their dataset is simply more diverse or what’s going on with the potential biases in Dall-E 2 results.