Fighting fraud using machines
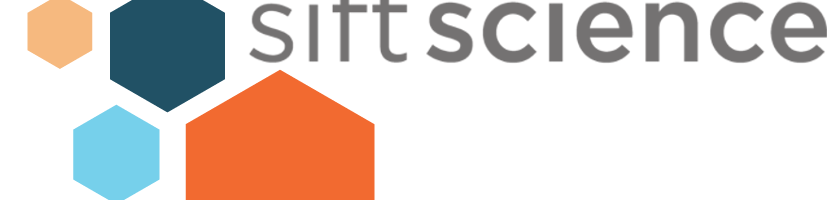
Sift Science uses machine learning and pattern recognition to detect and prevent fraud. The company has strong network effects and has managed to create and capture value by improving its product over time.
In 2011, a group of former Google engineers formed Sift Science. Using algorithms, machine learning, and statistics, they are helping businesses fight online fraud. Sift Science launched through Y-Combinator and has raised a total of $24mn. Its clients range from small websites to high traffic platforms such as Airbnb, Instacart, Twitter, and Zillow. Sift Science’s competitive advantage lies in its ability to use machine learning to recognize fraudulent patterns (more than 1mn identified to date) and proactively address fraud incidents. Its business model is strengthened when new clients join the platform resulting in direct network effects.
The mechanics of Sift Science are based on machine learning and the vast data generated by its clients’ websites. Sift Science monitors thousands of users and signals that occur through combinations of information. Its clients can integrate to the platform using a simple Java snippet. Sift Science collects data from third parties to paint a holistic picture of each user. Typical information includes shipment and billing address, social media posts, IP address, and device fingerprint. The company searches for patterns associated with fraudulent activity, such as chargebacks, account takeovers, and fake referrals. For example, if the billing address of a user is far away from the shipment address, Sift Science may flag the user. Similarly, Sift Science discovered that fraudsters often used Alaska as their billing address because it is the first state on dropdown bars. Customers sort through the profiles of flagged users and provide feedback on Sift Science’s assessments. This process allows: a) Sift Science’s algorithm to learn through new patterns; b) new customers to customize the solution based on the unique needs of their business.
Sift Science creates value for customers by improving the user experience, reducing financial losses, and improving operations. Traditional fraud detection programs rely on fixed rules. For instance, a rule may flag a transaction above a certain amount or related to a geography. These fixed rules result in multiple false positives, which harm the user experience by creating unnecessary screens and transaction cancellations. Sift Science’s algorithm can bring false positives down to 10%, compared to 80% of screens using traditional fixed rules. An important part of the value proposition is financial savings relating to the reduction of fraud. Compared to traditional methods, Sift Science’s adaptive approach is much more effective. HotelTonight, a mobile app featuring last minute hotel deals, reduced chargebacks by 50% using the platform. Opentable reduced its manual reviews of e-gift frauds by 80%. Although Sift Science does not reveal the secret sauce of its algorithms, machine learning explains performance improvements. Fraudsters continuously change patterns, and as a result adaptive models are much more effective than rules in addressing fraud. Machine learning enables the solution to adapt to the unique needs of a business, thus being effective for all customers. Very importantly, strong network effects enable customers to benefit from each other. Using device fingerprinting, for example, Sift Science will ban a fraudulent user from all of its customers’ websites. When one customer identifies a new pattern, all other customers benefit through improved performance. Lastly, Sift Science’s adaptive, dynamic approach is much easier for companies to implement. Because fraud patterns are proactively flagged, customers do not have to go through the painful process of creating rules. The adaptive approach also helps customers’ websites grow in a scalable way.
When the company first launched, it offered its product for free for websites with less than 5,000 users, and charged customers with higher traffic. By being the first company to offer a free product, Sift Science managed to capture users and improve its algorithms during its early days. The company nowadays charges all customers for its products. Its pricing is volume based, by charging a few cents for each API call (devices), account creation, or card payment. Sift Science offers discounts to customers who have high monthly website traffic. So far, the company has been able to successfully capture the value it creates for customers by becoming part of their variable costs. Sift Science’s operating model relies on its innovative culture, the quality of its algorithms, its collaboration with third parties, and customer data. The product is more user-friendly and effective than traditional fraud prevention systems, however, Sift Science is playing in a competitive market. The company’s success will depend on its ability to keep acquiring user data and improving its algorithms.
Sources
http://marketingland.com/why-marketers-should-push-for-machine-learning-based-fraud-detection-152053
In the face of growing e-commerce fraud, many merchants not prepared for holidays
Thanks for the interesting post! Sift Science seems to have a clear value creation and capture strategy. If I was a potential customer, one of my concerns would be whether Sift is likely to be acquired relatively soon. It’s growing, provides great anti-fraud technology, and can be an attractive target for a giant e-commerce company like Amazon. In that case, will the other customers still be provided with the same level of service and privacy protection, or will it serve Amazon’s needs and wean off other customers over time? As a potential customer, I wouldn’t want to grow to rely completely on Sift, as it would be hard to replace afterwards.