EFL: The psychology behind risk management
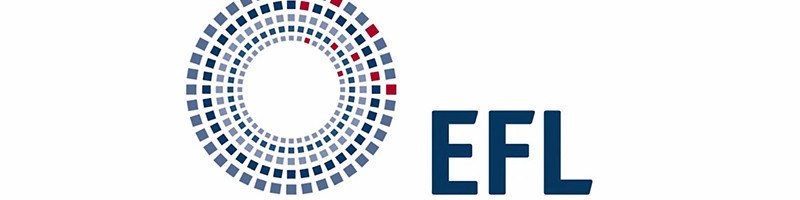
Harvard born startup based in Perú is closing the information gap in order to boost access to credit in developing economies.
Industry Background
The financial services industry has always been one where decisions are rational and backed by evidence and quantitative analyses. When evaluating an acquisition, there’s a model to back up the investment decision. When extending credit to a company, you analyze its financial statements and evaluate their credit history. Nevertheless, when it comes to personal loans or credit cards, it is impossible to conduct a thorough evaluation of every single potential customer applying to credit; that is why banks would traditionally rely on credit scores (which are statistical models designed to predict a client’s default probability by analyzing historical data) to decide whether to approve or deny a new credit application.
This is true for banks around the world. Nevertheless, the reality of each society determines specific barriers that harm the ability of banks to penetrate the market in an efficient way. From my experience in Latin America, I could say that the two greatest obstacles that keep the retail banking industry from growing are: Data scarcity and low quality of the data.
Data Scarcity
Unlike the United States, a large percentage of the population in developing economies has never interacted with a bank, and they don’t want to either. Having a cash based lifestyle, absolutely no credit history or a savings or checking account makes it impossible for traditional credit scores to have a consistent evaluation of these clients. This results in banks not lending to these people, which perpetrates the vicious cycle. Many times (when regulation allows it), banks recur to alternative variables (e.g: demographic variables) to build statistical models of lower predictive value.
Low Quality
Whenever banks have to rely on “alternative variables” (which have to be collected by asking the customer), many times clients lie in their credit applications fearing to not be “good enough” to be deemed worthy in the eyes of the bank. Moreover, some times, sales representatives, incentivized by their sales commissions and empowered by their empiric knowledge of the evaluation models, would game the system by falsifying information in order to get a higher approval rate.
Value Creation & Value Capture
EFL creates value in the industry by providing an alternative statistical model to predict potential customers’ default probability. It collects psychometric information of applicants through a survey that allows banks to create an additional axis to segment a given population. Customers respond to these questions in the banks’ branches during a credit application process, and data input is supervised by a sales representative.
After piloting the predictive power of the model on top of a traditional credit score (Equifax), EFL concluded that its proprietary model could decrease default rates by half while maintaining the same loan volume or increase volume by 140% while maintaining the same default rates. This will automatically translate into higher revenues or lower losses for the client bank, which is shared with EFL in the form of fees.
EFL has managed to successfully build solid relationships with banks and retailers in Perú, and it’s continuing to grow in Latin America and South Asia.
The following graph illustrates how EFL allows for better segmentation:
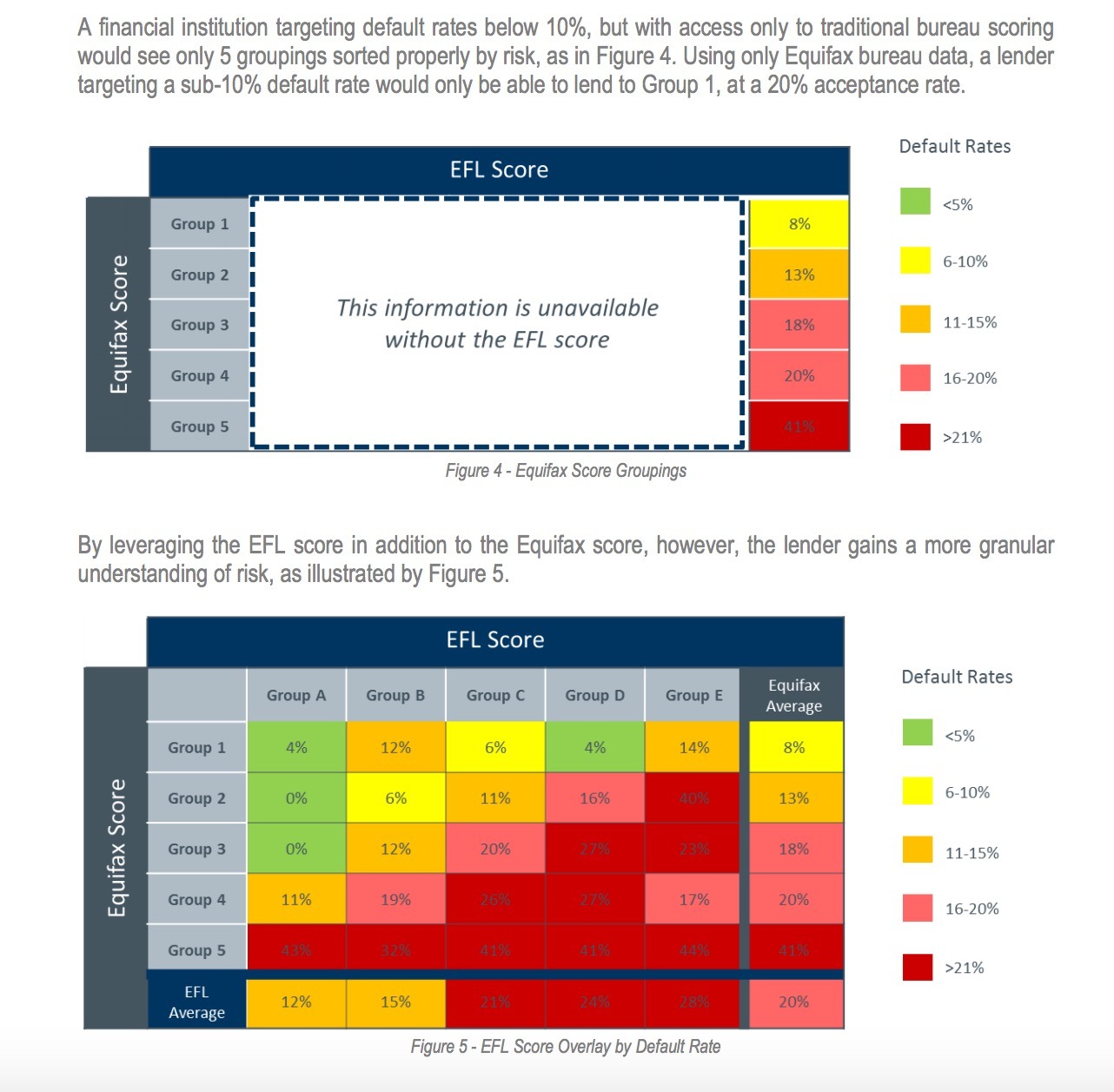
Risks going further
As with any data analytics business, the most important element is the data that is inputted in the model. EFL might provide a better tool to discriminate risk when traditional models don’t work, but if the data is not true, the whole model is in danger. Customers, or even sales people could still figure out how to manipulate inputs to get better approval rates and ruin the statistical significance of the product. Since the data collection process is decentralized, EFL should ensure appropriate and effective controls are set up to prevent this from happening.
On the other hand, EFL is building and improving its algorithm through data from its clients’ history. When deploying the model with new client, some biases could be transferred from one competitor to the other. In order to avoid this, EFL must ensure that its partners are not using arbitrary evaluation policies on top of the psychometric tool, or again, the data will lose statistical significance.
Thank you for your post, Andrea. I think what EFL is doing is laudable both for creating a potentially profitable business model and for potentially increasing credit access for underserved populations. I also agree that data integrity is a key concern. This could manifest not only due to the data collectors, but also because borrowers may figure out how to “game” the system. The nice thing about traditional credit scores is that they tend to be based on more objective criteria such as default histories and income. While far from perfect, they are harder to fake. Psychometric analyses, on the other hand, are subjective by nature – they have optimal answers that potential borrowers can learn. As such, the value of any given set of questions will likely depreciate and the questions that replace them may not have the same predictive power. Thus, I worry that what starts out as a promising tool may devolve into a non-value-added questionnaire.
If it is somehow possible to ensure that the answers to psychometric questions can’t be gamed, I wonder if there would be any regulatory outcry. After all, if a person cannot game the system, that must mean the questions judge something intrinsic about the person. Insofar as these intrinsic characteristics correspond to easily identifiable features (i.e. race, religion, hometown, language, etc.), the tool could be accused of discrimination. Obviously, every type of credit score is discriminatory in some sense, but society is more likely to accept discrimination based on default history than more intrinsic characteristics.
I completely agree with the concerns you raise. Comparing behavioral variables to psychometric or demographic variables will always result in a better discrimination power from the first ones because they measure objective observable actions. Nevertheless, those preferable risk models are unavailable for populations of unbanked individuals and micro businesses, specially in developing economies. EFL’s model adds predictive power on top of traditional scores. Which means that banks who implement EFL’s score in their evaluation process will not stop using Equifax all together. While its susceptibility to “gaming” is significantly higher than a traditional score EFL has taken measures to counteract the risk of faulty data. I asked the Director of Innovation about this and he explained how sometimes it’s now “what” the individual answers, but “how” (e.g. how long does he take to reply, how many times does he change his answer).
About the regulatory outcry, I can totally see that happen in the U.S. In Latin America, on the other hand, we can even use demographic variables to find correlations with default rates. This, in the U.S. is discrimination and is forbidden. I understand how both these policies makes sense in the markets where they’re applied; having a less mature market where high quality behavioral data is scarce, many times you need to use all resources available regardless of how precise they are.