Data Science and Your Local Utility Company
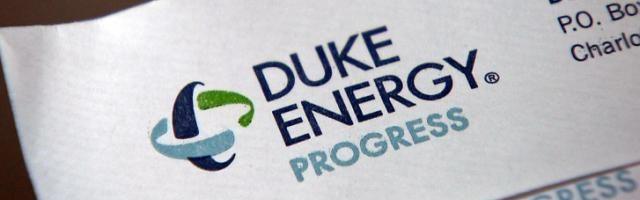
Duke Energy, despite being part of “an inherently slow-moving sector,” is among many utility companies reaping the benefits of data science.
Primer.
Please watch this 2-minute video for an overview: https://www.youtube.com/watch?v=pdrQDOhjF24
Introduction.
Duke Energy is a North Carolina based energy holding company that services 7.2 million customers across the United States and operates more than 250,000+ miles of distribution lines across 104,000 square miles.[i] Despite being part of “an inherently slow-moving sector,” Duke Energy is among many utility companies reaping the benefits of data science.[ii]
Journey.
Since 1904, Duke Energy has operated hydroelectric, nuclear, coal-fired, and natural gas turbine electric plants.[iii] In recent history, the rising cost of maintaining the United States’ aging grid system and the threat of distributed generation and more energy efficient customers has threatened utility company profits, beginning what some experts call the “utility death spiral.” The death spiral theory predicts that as more and more customers move to solar and other forms of distributed power generation, fewer people will be on the grid, which will decrease the number of people that utilities can distribute their costs to, which increases the price of energy to consumers on the grid, which then incentivizes more customers to move off the grid, continuing the downward spiral. As a result, utility companies, like Duke Energy, are looking to cut costs and increase revenue.
It was in this context, that Duke Energy sponsored the Data Modeling and Analytics Initiative (DMAI) in 2012. In this forum , vendors were invited to analyze a sample data set and provide “opportunities and insights” for inclusion in Duke Energy’s “big data analytics strategy.”[iv] Insights from the DMAI included meter and customer analytics, distribution grid analysis, and security, as well as estimated values for reducing operational expenses, increasing revenues, and customer satisfaction (See Exhibit A).[v]
Exhibit A. Summary of Use Cases From DMAI[vi]
After DMAI, Duke Energy identified the following: (1) there was significant value potential in applying data science across its business, (2) internal data needed to be cleaned better, (3) specifications for overall data collection architecture were needed, and (4) Duke needed data science resource and skills. Duke Energy now had the confidence to pursue a “big data” strategy (See Exhibits B and C).
Exhibit B. Data Modeling and Analytics Framework [vii]
Exhibit C. Data Flow Architecture [viii]
Value Creation.
Duke Energy creates value by integrating traditionally siloed datasets and streaming data live from field sensors.[ix] Using a team of data scientists at its Analytics Competency Center, Duke Energy is able to not only look at what had happened or what is happening, but is also able to make predictions about the future. Duke Energy is able to translate insights from meter to distribution to generation into the following improvements (See Exhibit D): [x]
- Reduce power outages
- Reducing power outage time
- Predictive maintenance
- Equipment replacement timing
- Energy utilization modeling
- Risk identification
- Theft identification
- Sever weather impact predictions
- Failing meter identification
- Automated service dispatch
- Improved billing
- Lower call center volumes
- Customer segmentation and profiling
- Improved customer satisfaction
- Improved customer engagement
Exhibit D. Value Creation Example: Improving Power Outage Prediction by Using Additional Variables[xi]
Value Capture.
As discussed above, Duke Energy’s data science initiative creates significant value that is captured in the form of lower costs and new revenue opportunities. Duke Energy shares this value capture with customers in the form of cheaper, more reliable energy.
- Cost reduction. Duke energy has saved millions of dollars using the insights highlighted above. Risk identification and equipment replacement time prediction has improved capital investments and equipment failure prediction has reduced maintenance costs.
- Revenue Opportunities. Identifying energy theft and failing meters and improving billing has allowed Duke Energy to recover millions of dollars in revenue. Duke Energy can also offer new products and services to different customer segments, including “demand-side management programs that reduce electricity use at peak times.” [xii]
Challenges.
As Duke Energy embarked upon its big data journey, it had to overcome several obstacles, including:
- Too much data, limited storage capacity, and not enough computing power. For example, for every one million smart meters Duke installs, 35 billion usage readings are generated each year. If Duke Energy outfitted every customer with a smart meter, which can also measure “voltage, volt-ampere reaction, meter events, and temperature,” that would be 1.4 trillion data points.[xiii] Duke also did not have a processing architecture that could handle the volume or generate the models needed. As a result, Duke Energy purchased a big data platform, Hadoop, to help configure, store, manage, and model its data. [xiv]
- Duke Energy did not have any data scientists. The company had to create new job positions and descriptions and had to hire and onboard new employees.[xv] Duke Energy could have outsourced this work, but decided to make a long-term investment and build the capability in-house.
- Buy in. The majority of technicians and utility workers have several years of real world experience and typically make a career of the utility industry. At first, they were slow to accept the new technology, but they soon became fans when they discovered it made them better at their jobs. Before the data science initiative, technicians would drive to every transformer on a monthly schedule to check sensors and perform tests. Now the technicians know exactly where to go and what problems to anticipate.[xvi]
In the future, Duke Energy will have to overcome these and other challenges:
- Not all transformers have sensors. Retrofitting these and other pieces of equipment can be costly, but not having data can also be costly. Should Duke Energy retrofit every piece of equipment or wait for replacement?
- The industry does not have a rigorous standard for capturing, storing and managing data.[xvii] Duke Energy is in the process of doing this and will continue to learn over time.
- Distributed Generation. Can data analytics be used to limit the impact of customers lost to solar or capture new value from these lost customers?
Conclusion.
With companies like Duke Energy leading the utilities industry into the data science age, other players have been quick to see the value potential. While individual utility companies are not in direct competition with each other, a fight for value capture has arisen between the different players in the ecosystem, which includes: utility companies, transformer and equipment manufacturers, oil and gas companies, and third-party consulting firms, such as IBM. Who owns the data? Who can generate the best insights? Who will share in the value created?
[i] https://en.wikipedia.org/wiki/Duke_Energy
[ii] https://en.wikipedia.org/wiki/Duke_Energy
[iii] https://www.duke-energy.com/our-company/about-us/our-history
[iv] https://www.elp.com/articles/powergrid_international/print/volume-19/issue-8/features/duke-energy-s-data-modeling-analytics-initiative.html
[v] https://www.elp.com/articles/powergrid_international/print/volume-19/issue-8/features/duke-energy-s-data-modeling-analytics-initiative.html
[vi] http://geospatial.blogs.com/.a/6a00d83476d35153ef01a73d6b4baa970d-popup
[vii] http://geospatial.blogs.com/geospatial/2014/01/distributech-2014-duke-energys-journey-toward-a-big-data-architecture.html
[viii] http://geospatial.blogs.com/geospatial/2014/01/distributech-2014-duke-energys-journey-toward-a-big-data-architecture.html
[ix] https://www.leidos.com/infrastructure/newsroom/article-duke-energys-data-modeling-analytics-initiative
[x] http://www.bain.com/publications/articles/how-utilities-are-deploying-data-analytics-now.aspx
[xi] http://www.bain.com/publications/articles/how-utilities-are-deploying-data-analytics-now.aspx
[xii] http://www.bain.com/publications/articles/how-utilities-are-deploying-data-analytics-now.aspx
[xiii] https://energy-analytics.energycioinsights.com/cxo-insights/coming-to-grips-with-analytics-on-big-data-at-duke-energy-nwid-191.html
[xiv] https://energy-analytics.energycioinsights.com/cxo-insights/coming-to-grips-with-analytics-on-big-data-at-duke-energy-nwid-191.html
[xv] https://energy-analytics.energycioinsights.com/cxo-insights/coming-to-grips-with-analytics-on-big-data-at-duke-energy-nwid-191.html
[xvi] Personal interview with utility transformer technician, July 2017
[xvii] http://www.bain.com/publications/articles/how-utilities-are-deploying-data-analytics-now.aspx