Beat Cancer with Enlitic: A Winner in Medical Diagnostics
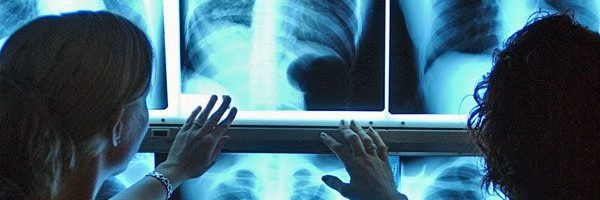
AI has become ubiquitous in our daily lives, influencing online search results to personal styling recommendations. Are we ready to let computers read our X-rays?
Context
A fledgling startup, Enlitic is shaking up the world of disease diagnostics one X-ray at a time. Founded in 2014 with $15MM in funding to date, Enlitic harnesses deep learning, a subset of AI that leverages sophisticated and parallel computational techniques mimicking the human brain, to train computers to screen medical images for cancer and fractures.[1] And with unprecedented access to cheap computing power, sophisticated algorithms, and troves of data, Enlitic is just getting started. After reflecting on its business model and assets, I see Enlitic as a winner, fundamentally changing the healthcare ecosystem as we move to a world where cost-management increasingly matters for providers pressured by healthcare outcomes vs the previous fee-for-service model.
How Enlitic creates value via its operating model:
1) It beats your doctor on accuracy and speed of diagnosis, leading to cost savings and incremental revenue generation for diagnostics. When exposed to a dataset of X-ray images, Enlitic’s algorithm identified instances of lung cancer more accurately than a panel of radiologists – and by 50%.[2] Its algorithm can detect abnormalities that may be overlooked by the human eye, identifying minuscule fractures .01% the size of the entire X-ray.[3] Pitched as a tool to help doctors rather than to replace them (for now), the company further claims to increase diagnostic accuracy by 50-70% and speed by 50,000X.[4]
Why does this matter?
The efficiencies yielded from this technology allow a single doctor to be scaled to conduct more tasks.[5] By allocating routine, time-consuming image tasks to machines, doctors can focus on higher-level tasks, such as treatment recommendations, that require human-level judgment beyond the current capabilities of AI.[6]
One could see Enlitic’s technology driving commoditization in the service of diagnosis itself, thus increasing the value of further downstream services like treatment.
2) With renowned data scientist Jeremy Howard at the helm, Enlitic has the data science expertise to continually hone a diagnostic algorithm that self-learns and gets better with more training data. It may even be trained faster, better, and cheaper than your average med school student. [7] Network effects add further to this feedback loop, allowing for information sharing across previous hospital siloes of information.
3) This could eventually extend diagnostic services to underserved segments, including rural patients or those treated during times where the right doctor is unavailable.
4) The algorithm’s diagnostic process leaves behind an audit trail of how it came to its decision, which serves as an asset in pedagogy and litigation.
How Enlitic captures value:
Through sharing in the profit realized from its clients.[8] This is a risky move for a startup with a limited track record, but one can argue this demonstrates its confidence in its potential. While there’s limited public information on Enlitic’s traction to date, we do know it is being implemented by one of its investors Capitol Health Limited, an Australian healthcare company providing diagnostic imaging services.[9]
While Enlitic exhibits a winning strategy, I’ll be watching to answer some outstanding questions:
- Will we see continued adoption by doctors? There’s lots of hype around machine learning’s predictive potential, but the way it arrives at a given conclusion can be a black box. Adoption of similar AI-powered diagnostic tools like IBM Watson’s partnership with Boston Children’s Hospital suggests there is potential here.[10]
- What effect will adoption have on patient outcomes?
- Regulations on how to use AI in healthcare to follow…
Sources:
[1] https://blogs.nvidia.com/blog/2016/07/29/whats-difference-artificial-intelligence-machine-learning-deep-learning-ai/
[2] http://www.nanalyze.com/2016/02/enlitic-deep-learning-algorithms-for-medical-imaging/
[3] http://www.nanalyze.com/2016/02/enlitic-deep-learning-algorithms-for-medical-imaging/
[4] http://www.nanalyze.com/2016/02/enlitic-deep-learning-algorithms-for-medical-imaging/
[5] http://jamanetwork.com.ezp-prod1.hul.harvard.edu/journals/jama/fullarticle/2588764
[6] http://jamanetwork.com.ezp-prod1.hul.harvard.edu/journals/jama/fullarticle/2588764
[7] http://jamanetwork.com.ezp-prod1.hul.harvard.edu/journals/jama/fullarticle/2588764
[8] http://www.nanalyze.com/2016/02/enlitic-deep-learning-algorithms-for-medical-imaging/
[9] http://www.nanalyze.com/2016/02/enlitic-deep-learning-algorithms-for-medical-imaging/
[10] http://www.mobihealthnews.com/content/roundup-more-dozen-ibm-watson-health-related-partnerships
Great post, really interesting company! The application of AI to automated screening for medical imaging totally makes sense. I believe that a computer algorithm will be able to more accurately and repeatably identify fractures and tumours. I’d be interested in how Enlitic plans to share in their clients’ profits to capture value, will they take a fee for every time their device is used or perhaps try to share in the cost savings or increased revenues. How the value capture model shakes out may also have an implication on legal liability down the line — what happens if the technology produces an incorrect diagnosis and the doctor goes off that recommendation, who is at fault then? Is the company willing to take on these liabilities?
Yep, lots more to be done in understanding how to regulate this. Hence, I think that’s why traction has been slow — the primary use case will be in training rather than in practice until the intricacies are ironed out.