[2] Kaggle: Building deep communities for deep learning
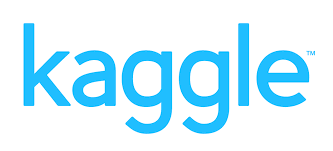
Kaggle is a competition platform that leverages crowdsourcing to find solutions for deep learning problems. This blog summarizes how Kaggle managed to create value and its challenge to stay relevant
What is the common denominator of grading high school essays and improving the search for Higgs Boson at CERN? The answer is deep learning! Being one of the most promising areas of research, deep learning has attracted several successful commercial players, Kaggle being one of them.
Founded in 2010 by Anthony Goldbloom and Ben Hamner, Kaggle is one of the leading platforms hosting data science competitions focused on deep learning solutions. The platform is a perfect case example to the democratization of innovation through crowdsourcing that multibillion dollar companies are leveraging today. While the company has several competitors like TopCoder and HackerRank, it has managed to stay ahead thanks to its focus on deep learning. Since its inception, Kaggle turned from just being a competition platform to a “hub for applied machine learning research”.
How does Kaggle create and capture value?
Kaggle’s biggest asset is its community consisting of 880,000+ data science members spanning more than 200 countries. Kaggle creates value both for its community of data scientists and the companies/organizations that host competitions on the website. As for the community, the primary value is created through monetary prizes offered in competitions, which range from as low as few hundred dollars to millions of dollars.
Besides immediate monetary benefits, Kaggle posts job opportunities for its community members. The platform has become an important resource for its job-seeking members who have landed jobs in companies such as Walmart and DeepMind. The platform also offers intrinsic motivations to its community such as the flexibility for participants to self-select, creativity and impact potential of competitions, which are at least as important as the extrinsic monetary motivations. Moreover, Kaggle offers immense learning opportunities to its community members. Through Kaggle Scripts (which were renamed to Kernels in 2016), Kaggle encourages users to publicly share their code on the platform. Also in 2016, Kaggle released the Datasets product, which made key datasets public on the platform.
As for the companies working with Kaggle, the solutions developed usually offer big advantages and cost savings. An example of a successful project was the development of computer-aided medicine, aiming to leverage deep learning to detect symptoms of vision loss due to diabetes. Around 80% of diabetes patients develop retinal damage that lead to vision loss, which is often preventable if detected early. California HealthCare Foundation (CHCF) used Kaggle participants to develop an algorithm that would accurately identify diabetes-related retinal damage 85% of the time for a cash prize of $100,000. Such a solution created big advantages for CHCF allowing for fast diagnosis as opposed to having to wait for laboratory results and obviating the need to have trained doctors.
In terms of value capture, Kaggle earns money by charging companies for the algorithms developed in competitions. Companies are given access to algorithms for 6 months for a flat fee, after 6 months companies are charged a monthly license fee.
Google acquisition and the path forward
While the crowdsourcing approach for deep learning innovations has worked well so far, the key challenge for Kaggle is to stay relevant in this low entry barrier-business. Except for its large community, the company’s business model can easily be replicated and many companies are building in-house solutions to crowdsourcing. Internally, Kaggle is also faced with the challenge of keeping its community motivated and retaining high level of participation. Since the size of monetary awards for contests does not depend on the number of submissions, it is crucial for Kaggle to attract participants by offering problems that are both interesting and approachable (for example they shouldn’t require supercomputers to solve problems!). Kaggle seems to be able to offer a wide range of problems that attract 3,500 competition submissions per day. On the other hand, the intelligence of trained models in deep learning increases in proportion to the size of data and computation power. Therefore, users are likely to require more computing power which is most commonly provided by cloud computing products. Currently, Amazon Web Services (AWS) is the undisputed market leader in cloud computing.
Just as the importance of cloud computing is increasing, an important turn of events happened to Kaggle recently. On March 8th, 2017 Kaggle CEO Anthony Goldbloom has announced that Kaggle will join Google Cloud and is reported that the company will continue to “operate as a distinct brand”. While there is excitement around the unlocking of potential from the combination of Kaggle’s large data science community with Google’s cloud business, there is also significant concern about losing the independence of the platform and losing the high level of participation. Building the ‘deep’ community was Kaggle’s differentiating play and Google is rumored to have acquired the company in order to tap into its vast pool of talent. Therefore, it is crucial for Kaggle to sustain the rate of growth in its community participation, otherwise the company can quickly become obsolete.
[1] https://techcrunch.com/2017/03/07/google-is-acquiring-data-science-community-kaggle/
[2] https://techcrunch.com/2017/01/12/kaggle-hosting-1m-competition-to-improve-lung-cancer-detection-with-machine-learning/
[3] http://www.economist.com/blogs/babbage/2011/04/incentive_prizes
[4] http://www.economist.com/news/science-and-technology/21664943-computers-can-recognise-complication-diabetes-can-lead-blindness-now
[5] http://blog.kaggle.com/2017/03/08/kaggle-joins-google-cloud/
[6] https://en.wikipedia.org/wiki/Kaggle
[7] http://blog.udacity.com/2016/07/companies-kaggle-machine-learning-talent.html
[8] http://www.datamation.com/cloud-computing/slideshows/top-10-cloud-computing-companies.html
[9] http://venturebeat.com/2017/03/15/what-the-kaggle-acquisition-by-google-means-for-crowdsourcing/
[10] http://www.inc.com/magazine201403/darren-dahl/big-data-crowdsourcing-kaggle.html
Great post Oyku! I had participated in a Kaggle competition last semester during a Bigdata course and it immensely helped me. However, I somehow felt it did not give me the complete flavor of identifying the problem, data mining, cleaning the dataset and coming up with valuable insights given it’s pre-packaged problem format where the data is anonymized or even changed at times. I know Kaggle is still far ahead of its game but considering the low barriers-to-entry in this industry and increasing sophistication around data analytics, do you think and if yes, how Kaggle should consider evolving their business model?