Where is Alpha? – Machine Learning at D. E. Shaw
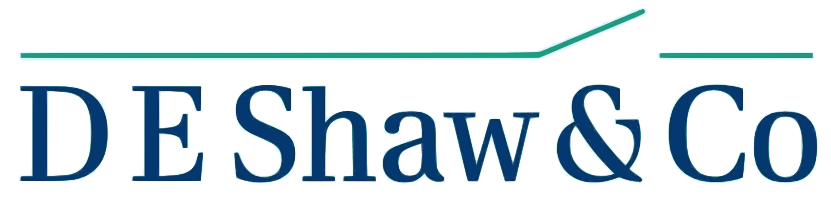
Can machines outsmart humans in the market? D. E. Shaw believes they can.
Does Machine Learning Help You Become Richer?
There has been a huge debate on whether machines can outsmart humans in the financial market. Over years, hedge funds with quantitative (“quant”) investment strategy have been increasingly incorporating machine learning to predict the future market movements. The D. E. Shaw Group (“D. E. Shaw”), one of the largest quant hedge funds in the world, is among many such hedge funds which have been promoting machine learning to boost investment returns.
The Importance of Machine Learning at Quant Hedge Funds
Quant hedge funds approach investing using quantitative methods to analyze the past financial market patterns, in the hope that it will help them predict the future market directions. Traditionally, renowned scientists, mathematicians, and physicists led such quantitative analysis at those funds. D. E. Shaw, established in 1988 by its namesake Mr. Shaw, has had successful investment track record, and manages almost $50 billion as of January 2018. [1] Because superior investment returns attract more capital from investors, quant hedge funds have tirelessly tried to improve their algorithms day after day. Machine learning is one of the latest trends at quant hedge funds, because many investors believe that its statistical approach can predict patterns and associations within financial market data, and can achieve higher investment returns.
Can the Smartest Humans Outsmart the Market?
To stay competitive in the short-run, more than 250 developers and engineers at D. E. Shaw are constantly analyzing and improving its trading algorithms based on machine learning. [2]
Also, as a medium term strategy, in August 2018, D. E. Shaw announced that they formed a new machine learning group led by an esteemed University of Washington Professor of Computer Science Pedro Domingos. [3] Dr. Domingos, an expert on machine learning, will lead the new team to analyze the market data from the latest machine learning perspective. According to a job description of one of the open Machine Learning Researcher positions, their responsibilities include “designing, implementing, testing experimentally and scaling up new models for forecasting asset prices, developing new tradeable signals, and other financial applications.” [4]
However, D. E. Shaw is not the only quant hedge fund which utilizes machine learning and artificial intelligence. In fact, 2018 Hedge Fund Sentiment Survey by BarclayHedge revealed that more than half of respondents (managers of hedge funds and commodity trading advisor funds) use machine learning and artificial intelligence to inform their investment decisions. [5] In fact, many of D. E. Shaw’s competitors have been aggressively poaching machine learning experts from tech companies and universities. Earlier this year, J.P. Morgan hired Dr. Manuela Veloso from Carnegie Mellon University to lead the firm’s Artificial Intelligence Research. Goldman Sachs lured machine learning expert Charles Elkan from Amazon. [6]
Recommendations – Integration and Black Swan
Although D. E. Shaw seems to have taken the right steps to stay ahead of the competition, I believe there are a few remaining issues that need to be addressed. In the short term, the firm needs to integrate the new machine learning team to their existent investment teams. As the new team comes up with new machine learning algorithms, other trading teams need to adopt the new algorithms appropriately and swiftly. It is imperative that the company will establish sophisticated communication and integration channels among the machine learning team and other teams, because fast and accurate algorithm adoption is a key to success in the rapidly-changing market environment.
In the medium term, D. E. Shaw should also control its risks and hedge against “black swan” events, which are high risk fat tail events that only occasionally happen with catastrophic results in the market. The dissolution of the legendary quant hedge fund Long Term Capital Management L.P. (“LTCM”) in 2000 occurred because the smart Nobel-laureates behind the company significantly underestimated the likelihood of an apocalyptic market downturn, which materialized in 1997 and 1998. Given some overlapping investment strategies between D. E. Shaw and LTCM, it may not be a coincidence that D. E. Shaw’s investment performance also stumbled in the same period. Apart from avoiding excessive leverage, which led LTCM to the notorious bankruptcy, D. E. Shaw’s machine learning team should carefully assess the potential magnitude and implications of catastrophic market downturns.
Where is Alpha?
Given the ever-changing financial market environment, can machine learning beat the market in the long run?[7] It should be helpful to think whether machines can predict the future market movements that most often occur independently from past market patterns. Also, in order not to repeat the LTCM fiasco, how can D. E. Shaw prepare for the next black swan event? (789 words)
Exhibit 1 Performance Comparison between AI Hedge Funds and the Broad Market
Endnotes
[1] D. E. Shaw website. (2018). Assets Under Management. [online] Available at: https://www.deshaw.com/what-we-do [Accessed on 11 Nov. 2018].
[2] D. E. Shaw website. (2018). What We Do. [online] Available at: https://www.deshaw.com/what-we-do#investment-approach [Accessed on 12 Nov. 2018].
[3] D. E. Shaw Press Release. (2018). D. E. Shaw Group Forms New Machine Learning Research Group |[online] Available at: https://www.deshaw.com/assets/articles/MLV_Release.pdf [Accessed on 10 Nov. 2018].
[4] D. E. Shaw website. (2018). D. E. Shaw Group Forms New Machine Learning Research Group |[online] Available at: https://www.deshaw.com/careers/3693 [Accessed on 11 Nov. 2018].
[5] BarclayHedge. (2018). Majority of Hedge Fund Pros Use AI/Machine Learning in Investment Strategies |[online] Available at: https://www.barclayhedge.com/majority-of-hedge-fund-pros-use-ai-machine-learning-in-investment-strategies/ [Accessed on 11 Nov. 2018].
[6] Medium. (2018). Pedro Domingos Will Lead New D.E. Shaw Machine Learning Group |[online] Available at: https://medium.com/syncedreview/pedro-domingo-will-lead-new-d-e-shaw-machine-learning-group-3c722e41aafc [Accessed on 11 Nov. 2018].
[7] Will Knight. (2016). Will AI-Powered Hedge Funds Outsmart the Market? |MIT Technology Review[online] Available at: https://www.technologyreview.com/s/600695/will-ai-powered-hedge-funds-outsmart-the-market/ [Accessed on 12 Nov. 2018].
[8] (Featured Image). D. E. Shaw Logo |[online] Available at: http://www.luminatellc.com/wp-content/uploads/2018/05/DEShaw-logo.png [Accessed on 12 Nov. 2018].
[9] (Exhibit 1). Nishant Kumar. (2017). How AI Will Invade Every Corner Of Wall Street|Bloomberg [online] Available at: https://www.bloomberg.com/news/features/2017-12-05/how-ai-will-invade-every-corner-of-wall-street [Accessed on 12 Nov. 2018].
The markets are one of the most complex man-made systems in existence. However, they are based on real relationships and rules, and thus eventually I think machine learning will exceed the best human judgment. As we learned in Finance though, alpha will disappear as soon as “everyone” has these algorithms, leading potentially to a market machine learning arms race not unlike the chess-playing computer arms race that still exists today.
Very interesting piece. Agree that markets function based on relationships between many different variables and market constituents will move markets based on pattern recognition. Based on this, I believe machine learning will continue to be highly valuable in public market investing. Would be interested to see how this relates to other areas of investing where human to human relationships matter more, like building relationships with managers in private equity. Separately, in public market investing, i believe quant based trading and a machine learning based approach have become more prevalent given the shift to passive investing (via increase of mutual funds and ETFs) – what would happen to accuracy of machine learning if the pendulum shifts back to active investing (i.e. individual stock picking based on fundamentals vs. technicals)?