What do you get when you cross a lawyer and a computer? A better lawyer!
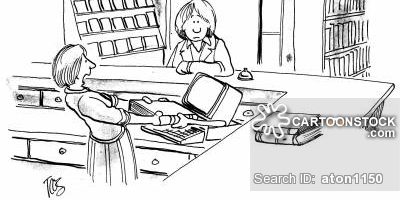
Can computers save the most depressed profession in the country? kCura is building the software to rethink the dreaded document review process.
For decades, it was a rite of passage: sequestered in windowless rooms, surrounded by boxes of documents, and scolded by well-heeled partners, associates at top law firms had to “put in their time” doing document review for litigation discovery. Document review for discovery is a laborious and repetitive task akin to searching for a needle in a haystack. It is initiated in the earliest stages of a litigation proceeding and allows for each side of the dispute to gain access to relevant evidence that isn’t considered privileged communication. The trouble comes in sifting through mountains of irrelevant documentation to find responsive documents.
In the pre-digital era of law, discovery meant reviewing physical documents (external correspondence, internal memoranda, sales contracts, and more). In the digital age, this information has moved from filing cabinets to hard drives. With the move toward digital, though, has come a proliferation of data – International Data Corporation predicts that the digital universe will expand to 5,200 gigabytes of data for every human by 2020[i], the equivalent of over 5,000 truckloads of documents! kCura, the maker of Relativity e-discovery software, has positioned itself to take advantage of the opportunities presented by the digitization challenge that faces the legal industry.
Breaking the Tradeoff
The business model of document review firms has always revolved around the customer promises of speed, cost, and accuracy. Tradeoffs and tension bubbled beneath the value proposition: going faster required larger teams and more layers of management and thus increased cost, accuracy meant hiring smarter people who demanded higher wages and thus increased cost (and even then did not guarantee accuracy[ii]), and increasing accuracy often meant adding QC stages and thus slowed the process. kCura’s approach, though, is to break these tradeoffs with the application of e-discovery technologies for a faster, cheaper, and more accurate review.
Delivering the Unthinkable
The earliest use of technology in the review process amounted to little more than keyword-searches that weeded out large percentages of documents that did not contain relevant keywords. This process, while faster and cheaper than the manual review of yore, had major accuracy problems as responsive, non-privileged documents were ignored simply on account of the absence of certain human-picked keywords, with the FTI Journal noting that “such searches have been shown to produce as little as 20 percent of the relevant documents, along with many that are irrelevant.”[iii]
While e-discovery as an industry has taken off in relation to the increase of ESI (electronically-stored information), kCura’s Relativity technology is leading the way. Driven by proprietary “predictive coding” software, Relativity uses TAR (technology-assisted review), or supervised machine learning, to “show” computers what is responsive instead of “telling” them.
Building the Future
While Relativity has driven forward a major shift in how discovery is conducted, it’s technology is quickly becoming commoditized. Given their first-mover advantage, however, and the strong reputation they have established with key clients, there are several opportunities for them to stay one step ahead of the digitization challenge.
- Expand laterally into new markets – the same consumers (large corporates) could benefit from the same technology (technology-assisted review) in areas like commercial contracts and mergers & acquisitions.
- Expand vertically into higher-value add phases of litigation – while the lions’ share of cost occurs in the review phase, the most profitable categories of work lie farther up the food chain. Relativity could get involved in research, brief-preparation, and litigation prediction.
- Develop more advanced machine learning technology to further deliver on the customer promise of speed, cost, and accuracy – while supervised machine learning represents a major achievement over previous technological and manual forms of review, it is not foolproof. Unsupervised machine learning may further eliminate bias in the development of conceptual maps that lay bare relations previously unknown in the data.
While the e-discovery space will only grow to make the $250 billion market more efficient, Relativity is also at risk of significant regulatory intrusion. Legal services in the US are highly regulated, and the introduction of new technologies and business models has historically brought accusations from trade groups of UPL, or the unauthorized practice of law. Current predictive coding practices, while relatively untested in the courts, have fared well enough to-date. In Rio Tinto Plc v. Vale S.A., for example, the court ruled heartily in favor of the use of Relativity to deliver consistency in the production of documents[iv].
Lawyers need not fear an imminent threat – by freeing them from musky basement offices and papercuts, Relativity allows them to “put their time in” on much higher value add activities… until those activities, too, are better completed by computers.
[i] https://www.emc.com/collateral/analyst-reports/idc-the-digital-universe-in-2020.pdf
[ii] http://www.nytimes.com/2011/03/05/science/05legal.html
[iii] http://ftijournal.com/article/taking-predictive-coding-out-of-the-black-box-deleted
[iv] http://blog.kcura.com/relativity/blog/more-da-silva-3-takeaways-from-judge-pecks-rio-tinto-opinion
It was interesting to think about technology disrupting such an old profession like lawyers. The fact that a process as laborious as unenjoyable as document review can be partially outsourced to technology is monumental. I wonder how much of an impact that change will have in a law firm’s ecosystem. Especially as technology advances beyond just collecting relevant documents from a pool of documents, no longer will the rookie associates have to engage in laborious jobs. This increase in productivity of the labor force might mean that law firms might not need as many associates, making the prestigious firms even more competitive to get into.
As machine learning and natural language processing technology develop further, I think we will see gigantic improvements in products like kCura. Just like any machine learning process, discovering relevant documents will only get better and easier as the technology engages in more discovery and more feedback loop. At the same time, when lawyers start to rely too much on the technology for document discovery, I think it may be easy to fall into trap of confirmation bias. Unless humans vet the unidentified documents periodically, machines might pick up more and more similar documents and become blind to the relevant documents that the algorithm had trouble finding.
Dave, thanks for the interesting story on kCura. I looked up their website and was surprised by how much they segment their product offering. They break down Relativity into 8 different services, and also offer RelativityOne. Along with their service suite, they also offer different operating platforms, including one that is managed locally on the client’s hardware (and though costlier to use, is presumably more secure) and another that runs on AWS Cloud (cheaper, but potentially less secure). See: https://www.kcura.com/relativity/ediscovery-products/overview/ & https://www.kcura.com/relativity/how-to-buy/premises
I was surprised by how much differentiation kCura offers, and I wonder if it is too complex. Typically, SaaS companies strive to present simple pricing and product plans to consumers, especially if they are in a nascent business or are a first-mover. The fact that kCura breaks down the individual services worries me, as I feel like they may be nickel-and-diming customers, leaving the door open for competitors to take advantage. There are also substantially more competitors in this space than I would have imagined – found at least 20 on this one list: https://www.g2crowd.com/products/relativity/competitors/alternatives.
I strongly agree with your point on utilizing more unsupervised machine learning. I fear that their TAR program may do a solid job of automating the low-hanging fruit of document review, and would transition associates’ work to more complex or less common document review. However, as you pointed out, the goal of Relativity and other software products should be to complete document review in entirety, in order to allow associates to put their freshly minted JD degrees to higher-order use. At the same time, as Brittany pointed out, humans should always be involved in the process, even if as a checkpoint, since confirmation bias could creep in and lead to poorly performing automated document reviews.
Interesting and thought-provoking overview. I wonder a few things —
1) In this case, as so many attorneys end up be legislators, I wonder how much trust you have that the regulatory process will result in restrictions that protect the integrity of the system vs. simply protecting the industry’s position as a whole from disruption?
2) What does the advent of this technology and application in the legal space mean for the purchaser of legal services? Given that we are substituting high up-front, low run rate costs from technology in the place of expensive human hours, will we simply see stagnant prices and improving margins? Or, by leveling the playing field for some analytical tasks, is there going to be price pressure exerted on the industry and prices fall as a result? Or is there some other outcome?
Thanks for sharing this article! I had the following thoughts to offer:
1. Competition: In looking into this space, I found several established firms that offered document review services (and outsourced legal processing services in general). These firms are investing in their proprietary software solutions to automate document review and other services. They have existing relationships with law firms globally and could leverage those relationships to upsell their new SaaS offerings, providing competition to the likes of kCura. See http://cobralegalsolutions.com/pdf/cobraTop10.pdf
2. Obsolescence: Technology majors are investing heavily in AI and text-interpretation. These companies have significant cash reserves and human capital to develop new technologies, and pose a high level of obsolescence risk to kCura. By way of example, see the Language Processing research unit at Google http://research.google.com/pubs/NaturalLanguageProcessing.html
DA, thanks for the post.
This is a great example of a situation in which technology works together with humans instead of replacing them. By automating the document discovery process, humans now have more time available to perform more value added tasks.
I am curious how the software actually works and what ideas/concepts were applied for its creation. In TOM class we discussed IBM’s Watson and to me both technologies seem similar. I wonder whether both are driven by the same type of algorithms or if they are fundamentally different.