Walking on the Sidewalk: Building the future of cities in Toronto

Sidewalk Labs, by applying technology like machine learning coupled with innovative urban design, is building the future of cities in downtown Toronto
As I wait for the bus at the stop on Union Station in the heart of Toronto’s Downtown, I reflect on the complexity of urban environments and the potential the appropriate application of technology can have to increase human quality of life. Toronto’s population has increased by 2% per year in that last 10 years and the growth continues to accelerate [1]. Accelerated urban growth in Toronto and many other cities across the world is bringing more than 54% of the world population to live and work in cities, compared to only 36% in the 1960’s. This growth comes with significant challenges, especially with regards to traffic congestion now that 87% of households own at least one car and the average commute time is over 80 minutes per day [2].
Toronto’s rapid growth, like in many other cities, has put pressure on municipal governments to use design and technological solutions like machine learning (ML) and connected devices and sensors (IoT) to optimize their operations and provide better services to their citizens. As an answer to these challenges, Sidewalk Labs, an Alphabet company, is taking an integrated approach to urban innovation that uses technology to complement new city planning paradigms that have the potential to address challenges such as housing affordability, mobility, health and safety. The overarching philosophy of their approach is to use technology as an enabler of effective human-centric urban design, not implementing technology for its own sake.
While studying which ML approaches could be effective to address smart city challenges, the nature of the data produced by cities has been analyzed. Smart city data is generated at a high rate and cannot be entirely reviewed by humans for justification. Additionally, the operating environment in cities changes constantly and has high degree of uncertainty. Given these characteristics, deep neural networks (DNNs) and reinforcement learning (RL) are seen as successful approaches to ML for urban data problems. DNNs are used to be able to add significant levels of abstraction to the massive amounts of raw data cities may generate and make it useful for systems to make real-time decisions. On the other hand, RL methods are used when there is no previous classification of the raw data, but the system is rewarded when the right action happens, maximizing the learning of the DNN on the long term [4].
Transit optimization
Bus and Light Rapid Transit networks are complex time-dependant systems that face significant variability and uncertainty on their demand. By implementing IoT sensors that measure the human traffic flow during different scenarios (e.g. time of day, season, special events in the city) and demand for transit, continuous flow of data can be fed into the DNN which with RL can optimize the bus and rail network. This application will increase the utilization of the transit network, reduce congestion and reduce long term costs of the system
Traffic control
Sidewalk envisions the application of modular street tiles that can change their use depending on demand. Instead of using pavement for roads and concrete for pedestrian side walks that limits the use of areas exclusively to one or the other mobility mode, a single modular material will be used for both areas increasing the flexibility of the space. These smart tiles will change features (colour, heating, texture) to adapt to the use that will be most demanded at a certain point in time, with dynamic changes in features according to ML predictive models of space use.
Sustainable resource use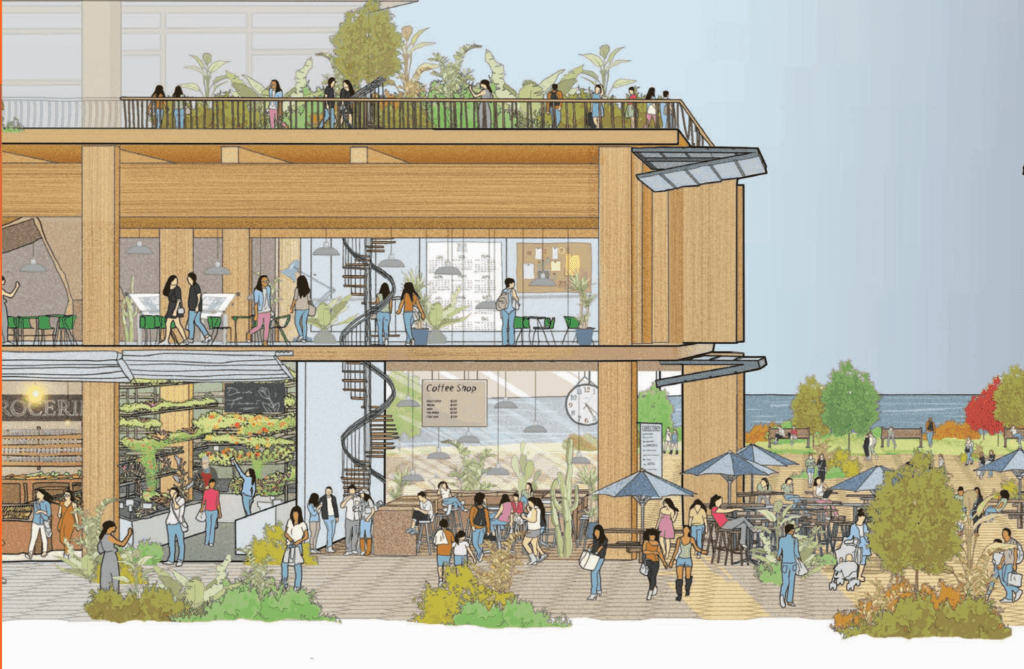
Energy and water consumption are key drivers for resource consumption in cities and their efficient management is essential for sustainable growth. Using big data from weather patterns, city temperature, humidity sensors and smart meters for water and energy use, DMMs can be leverage to automatically adapt living environments to maximize resource efficiency. The system, with enough training, can predict the changes in demand and supply of water and energy to make changes to HVAC configurations in buildings to increase human comfort while reducing cost
Although the potential of ML to solve urban problems is high, critics are concerned with issues related to citizen’s privacy and data collection by private corporations that is a necessary input for ML solutions to derive the appropriate predictions. The method by which Sidewalk Labs, and its Toronto Quayside project, implements technology and navigates policy and privacy concerns will determine the future of ML and IoT applications in urban environment in the future, either stagnating innovation or creating immense opportunities to increase the quality of life of billions of people.
(751)
[1][2]: Statistics Canada, 2016
[3]: Sidewalk Labs Roundtable presentation, May 2018
[4]: Mohammadi et al (2018), “Enabling Cognitive Smart Cities Using Big Data and Machine Learning: Approaches and Challenges”
Very well written — I loved learning about the many ways in which Sidewalk Labs is utilizing data to improve cities! One additional article on Sidewalk Labs’ blog was particularly interesting because it highlighted how useful it can be to use data to impact policy makers. “Models provide the opportunity to create a dispassionate venue in which ideas can be explored and tested by anyone interested in the topic at hand. To achieve this goal we must first build models that resonate with decision-makers and the public as credible, legible, realistic, and compelling. We must then allow anyone the ability to create their own solutions, and investigate and explore solutions created by others” [see source below]. Given the heated political environment, and as you aptly stated, the rapid growth rates of many urban centers, using data-driven models to highlight issues, develop potential solutions, and drive alignment between many stakeholders — from residents to government workers — provides a refreshing alternative to heated political debates.
Source reference: Nick Chim and David Ory, “A key to democratizing urban solutions is building better models,” Sidewalk Labs (blog), April 24, 2017. https://www.sidewalklabs.com/blog/a-key-to-democratizing-urban-solutions-is-building-better-models/, accessed November 2018.
Great article and so relevant to cities that are attracting large levels of population growth (2% is a lot of people considering the size of Toronto). The allocation of resources and efficiencies thereof are critical going forward. It was great to read of a tangible application of ML that is easy to understand and can be comprehended in the near term.
I loved this article. Sidewalk Labs’ redevelopment of Quayside in Toronto is the latest iteration of the “Smart City” a la Epcot and the designs of Lewis Mumford. Many attempts of building the first practical smart city have failed. Why do we think Sidewalk Labs’ effort in Toronto is any different? Alluded to by the author, I think Sidewalk Labs is fundamentally reimagining the urban experience versus trying to create a more modern and techified urban experience. From the design of the modular street tiles to the use of data to limit resource consumption, Sidewalk Labs is focused more on creating a city that is “smart” across all dimensions, not just those that impact the daily experience of the city dweller. Data collection and use (via machine learning) is obviously essential in creating components that can operate automatically and intelligently behind the scenes.
I think the author hit the nail on the head with his or her concerns about data privacy. I would imagine, living in Sidewalk Toronto would require a willingness to give up the right to data privacy (even if it is de-identified). This might be a tough sell to most people and I hope they can create some assurance around data privacy. Machine learning and creating a new urban experience is great, but only if people want to live there. Sidewalk Labs seems to be going into this with its eyes wide open so I am encouraged by this attempt of reimagining the modern city.
An interesting read that sums up how Sidewalk Labs is driving urban innovation. As you mention, machine learning presents opportunities for a city to operate more efficiently and sustainably. The examples you provide of ML to enhance transit and sustainable resource use seem relatively uncontroversial to me. Data on passenger trends and transit usage is already collected by urban transit agencies – and passengers are likely to appreciate the efficiencies that come with better matching supply and demand. Similarly, tracking weather patterns, temperature, and energy usage is an extension of current practices conducted by landlords and weather forecasters. Where ML becomes challenging is in more personal collection of data, as you mention, and the potential to attach user habits to individuals. I’m curious to track how Sidewalk Labs deals with data privacy and surveillance as it moves forward in Toronto.