Using machine learning to bend the healthcare cost curve
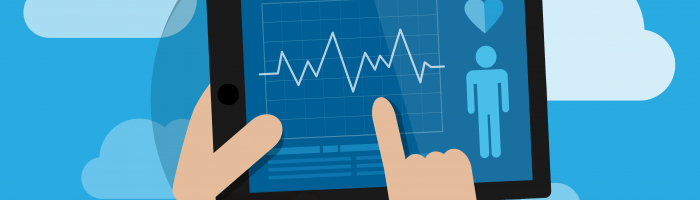
EHR and billing services provider athenahealth uses machine learning to automate administrative tasks – how can they use their unique market position to add more value for their clients?
Academics and politicians commonly decry the “health care cost crisis” in the United States. While spending more per capita on healthcare than any other country in the world, the United States has an average life expectancy in the middle of the pack among developed nations.[1] Though much of the cost burden in the United States is driven by high prices for treatments, as much of 20% of healthcare spending is the result of clinical waste and administrative complexity.[2] athenahealth, as a provider of electronic practice administration tools to small-medium-sized healthcare practices, has an important role to play in helping their clients reduce this wasted healthcare spending.
Machine learning is a crucial tool for this task, due to the volume and structure of healthcare data. Hospitals generate a breathtaking amount of data. Everything from a patient’s heartrate to the tone of a doctor’s clinical notes could conceivably be used to improve patient outcomes or reduce the amount of time a doctor needs to spend on a given patient (thus improving the cost effectiveness of healthcare).[3] However, this data is typically not structured in a way that is amenable to simple regression analysis or other less sophisticated methods of data analysis. Machine learning, then represents a huge opportunity for a company like athena to help their clients wade through the mountains of data that they generate.
athenahealth has several machine learning pilots that they are testing in parallel. athenahealth has thus far avoided the extremely complex field of computer-aided diagnosis – opting to start with lower hanging fruit. As Girish Venkatachaliah, vice president of data strategy, analytics and machine learning at athenahealth put it, “in the future, AI will benefit patients. But today it can help doctors.”[4]
athena’s most prominent machine learning program deals with faxes. Due to regulatory requirements, faxes remain the most common mode of healthcare communication. athenahealth’s 100,000 providers receive 120 million faxes every year.[5] athenahealth is using machine learning to automate some of the processes of importing faxed data into a hospital’s electronic medical record. Using machine learning to recognize and automatically transcribe common fields in faxed forms, athenahealth has been able to reduce the average time a clinic spends reading and importing a fax from over two minutes to one minute and 11 seconds.[6] These time savings add up and represent cost savings for clinics and for the healthcare system more broadly.
athenahealth has also begun using machine learning to automate the processing of prescription refill requests. A plugin for athenahealth’s software can evaluate a refill request and determine whether it meets treatment guidelines for a given patient.[7] The company claims that it can process up to 60% of prescription refills without involving a physician – again generating substantial time savings for clinics.
Saving time for clinics is a worthy goal, but for athenahealth to truly help bend the healthcare cost curve they will need to delve into improving the way that physicians administer care. Through its athenainsight service, athena is already providing descriptive data to its clinics about how other clinics treat a given condition. However, there is a huge opportunity to build on this service and begin offering proactive treatment recommendations. Some of the most innovative hospital systems are beginning to apply machine learning to understand the likelihood of success and/or complications for a specific procedure given everything they know about the patient’s health profile.[8]
Services like this are a natural next step for athena – and would be a huge value-add for their customer base. athenahealth’s user base consists of over 100,000 physician practices who treat 55 million patients per year.[9] athenahealth typically serves clinics on the smaller end of the size spectrum – many of which are under-resourced and/or located outside of major cities.[10] This massive user base represents an opportunity to democratize access to some of the most impactful machine learning tools used by top national hospital systems.
Obviously this sort of service comes with major ethical, legal, and logistical challenges. athenahealth would need to ensure that they implement safeguards on the system to preserve patient safety and patient privacy.
As machine learning is increasingly used as a decision support and/or automation tool in healthcare, several open questions remain:
- How can we maintain human safeguards in the treatment process while still realizing time/cost savings?
- How can we provide transparency into why a machine learning algorithm is making a recommendation?
(726 words)
[1] OECD, “OECD Health Statistics 2018,” http://www.oecd.org/els/health-systems/health-data.htm, accessed November 2018.
[2] Berwick DM, Hackbarth AD. Eliminating Waste in US Health Care. JAMA. 2012;307(14):1513–1516. doi:10.1001/jama.2012.362
[3] Brian Kalis et al., “10 Promising AI Applications in Health Care,” Harvard Business Review (May 2018): accessed November 2018.
[4] Gale Pryor, “AI poised to transform healthcare, one fax at a time,” athenahealth blog (March 2018): accessed November 2018 (https://www.athenahealth.com/insight/ai-poised-transform-healthcare-one-fax-time).
[5] Jonathan Bush, “How AI Is Taking the Scut Work Out of Health Care,” Harvard Business Review (March 2018): accessed November 2018
[6] Gale Pryor, “AI poised to transform healthcare, one fax at a time,” athenahealth blog (March 2018): accessed November 2018 (https://www.athenahealth.com/insight/ai-poised-transform-healthcare-one-fax-time).
[7] Rod Moore, “The next frontier for AI: Prescription refills,” athenahealth blog (April 2018): accessed November 2018 (https://www.athenahealth.com/insight/ai-poised-transform-healthcare-one-fax-time).
[8] Bill Siwicki, “Machine learning helps UI Health Care reduce surgical site infection by 74%, save $1.2 million,” Healthcare IT News, (September 2018): accessed November 2018, https://www.healthcareitnews.com/news/machine-learning-helps-ui-health-care-reduce-surgical-site-infection-74-save-12-million
[9] athenainsight, “About the Data” (2018): accessed November 2018 (https://www.athenahealth.com/insight/about/data).
[10] Dana Quattrochi, “Presentation to 2018 Investor Summit” athenahealth (February 2018),
Curious to understand what this means at the health clinic level. Does less time spent on importing information mean more time with patients, or is it simply cost savings in terms of fewer positions. Agree that the ability to have this information in a database type platform opens a lot of possibilities in terms of tracking and reporting on diagnosises based on conditions – but there’s probably a fair bit of data merging required for that which introduces some intricacies.
Thanks for sharing, this is really great work. Your question, “How can we provide transparency into why a machine learning algorithm is making a recommendation?” is particularly noteworthy because machine learning does offer the opportunity for more flexible modeling that may better approximate the true function that maps complex clinical inputs into meaningful insights. However, in order for machine learning to be deployed widely, there need to be real-time checks and balances on the algorithms developed in healthcare and as such providing both predictions and explanations for providers and patients will be crucial.
Thanks for this great read. Machine learning has many potential applications within healthcare, and as you alluded to, this is especially true when considering clinical decision making. A key way to maintain human safeguards is to use the algorithms as support tools – many of which are being rolled out in practices around the country. An important concern whenever creating a support tool to help make clinical decisions, is that each patient’s perspective on health value can be different. Therefore it is extremely important to ensure that there is no inherent bias encoded into these algorithms. Interestingly, just as a tool can be biased, so too can a physician. How can we ensure that this becomes an unbiased tool to improve informed consent, rather than an additional layer of bias through which a patient receives health information?