The internal-external AI gap: Why the UK’s leading AI healthcare startup refuses to deploy AI internally
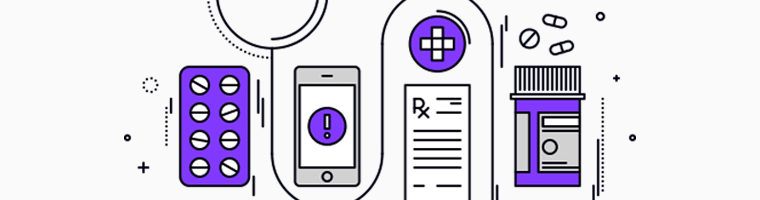
“It will soon be seen as ignorant, negligent and maybe even criminal to diagnose disease without using AI”[1] Ali Parsa, CEO of Babylon Health
Having launched in 2014 as a telemedicine provider, Babylon quickly pivoted into AI diagnostics, growing an in-house AI capability to rival that of Google’s Deepmind (one of the company’s earliest investors). In 2017, Babylon unveiled the UK’s first digital doctor’s practice and rapidly expanded internationally, launching in Africa with the Gates Foundation, the US with Samsung, and Asia with Tencent and Prudential.[2] By mid-2018, Babylon had grown to 3M users globally, 200 corporate customers and around 400 employees – including me.[3]
Whilst the ethical debate surrounding AI-healthcare remains as pervasive as ever, Babylon’s stance on the matter is clear – AI saves lives.[4] In a study co-published by Stanford University, Babylon put its AI head-to-head against human doctors. In true IBM Watson-Jeopardy style, Babylon’s AI outperformed the human – scoring 81% in accuracy of diagnosis (9% higher than the doctor).[5] It is hard to over-emphasise the impact that AI will have on our healthcare system, as Nature explains: ‘AI is poised to revolutionize many aspects of current clinical practice in the foreseeable future.’[6]
The potential of Babylon’s technology to meet the external needs of patients has been proven, but to what extent should they be investing in internal AI deployment? Babylon’s decision not to utilise their own AI capabilities has resulted in an abundance of internal inefficiencies and ballooning costs, with losses of £23.3M reported in 2017[7]. How should startups think about balancing the use of technology to gain operational efficiencies, versus purely as a means to gain new customers?
Why is AI important to Babylon?
Having amassed one of the largest clinical datasets in the world, Babylon uses a data science technique called Probabilistic Graphical Modelling (PGM) to link a patient’s symptoms with a probability-weighted diagnosis. The ‘Babylon Brain’ (Ex.1) summarises the key ingredients to their AI.
Ex.1: The ‘Babylon Brain’[8]
In addition to diagnosis and triage, Babylon provides a back-office service for doctors. We know that doctors spend twice as much time on administration as they do treating patients.[9] By automating common administrative tasks, Babylon offers doctors more time to spend with their patients (see YouTube video)[10]. So why has the company made such strides in improving back-office functionality for doctors, but not for their own employees? In a recent HBR publication, Satya Ramaswamy makes the case for prioritizing back-office AI deployment:
“Start in the back office, not the front office. You might think companies will get the greatest returns on AI in business functions that touch customers every day (like marketing and sales) or by embedding it in the products they sell to customers (e.g. the self-driving car etc.). Our research says otherwise.“[11]
Ex.2: How Companies Around the World Are Using Artificial Intelligence[12]
In focusing all of its efforts on its client-facing AI capabilities, Babylon is missing out on the efficiencies that could be gained from integrating AI across these critical functions.
Recommendations:
- Identify low-hanging fruit. As a first step, focus on integrating AI into functions which achieve the quickest wins (e.g. legal/compliance and workflow automation);
- Create a dedicated Internal AI Team. Create a cross-divisional team of Data Scientists and Operations talent, tasked with using AI to drive through efficiencies across the organization;
- Consider how internal AI capabilities can be commercialized externally. Internal and external AI development can be complimentary.
Open Question:
One critical question that I’d like to end with; when considering technology companies’ business models, should AI ‘Start in the back office, not the front office’?
(780 words)
References:
[1] Gary Finnegan, “Your virtual doctor will see you now: AI app as accurate as doctors in 80% of primary care diseases”, Science Business (February 19, 2018) https://sciencebusiness.net/healthy-measures/news/your-virtual-doctor-will-see-you-now-ai-app-accurate-doctors-80-primary-care
[2] Parmy Olson, “Rise Of The AI-Doc: Insurer Prudential Taps Babylon Health In $100 Million Software Licensing Deal”, Forbes (August 2, 2018) https://www.forbes.com/sites/parmyolson/2018/08/02/rise-of-the-ai-doc-insurer-prudential-taps-babylon-health-in-100-million-software-licensing-deal/#4d492769628f
[3] Mairi Johnson, “Babylon Commercial Presentation”, Babylon Health company documents (August 20, 2018)
[4] Finnegan, “Your virtual doctor will see you now”
[5] Saurabh Johri, “A comparative study of artificial intelligence and human doctors for the purpose of triage and diagnosis”, Babylon Health company website (June 2018)
https://assets.babylonhealth.com/press/BabylonJune2018Paper_Version1.4.2.pdf
[6] Kun-Hsing Yu, Andrew L. Beam and Isaac S. Kohane, “Artificial intelligence in healthcare”, Nature (October 2018), 726
[7] Sabah Meddings, “GP at hand app Babylon bleeds cash”, The Times (October 14, 2018)
[8] Johnson, “Babylon Commercial Presentation”
[9] Danielle Ofri, “The Patients vs. Paperwork Problem for Doctors”,
New York Times (November 14, 2017) https://www.nytimes.com/2017/11/14/well/live/the-patients-vs-paperwork-problem-for-doctors.html
[10] Royal College of GPs, “Babylon AI Event June 27th 2018 Portal Demo”, YouTube, published June 29, 2018,
[https://www.youtube.com/watch?v=AnbYX5qwbdU], accessed November 9, 2018
[11] Satya Ramaswamy, “How companies are already using AI”, Harvard Business Review (April 14, 2017) https://hbr.org/2017/04/how-companies-are-already-using-ai
[12] Ibid.
We have learned that the potency of machine learning algorithms is correlated with the quantity and quality of the data on which a machine is trained. You mentioned that this firm has access to one of the largest clinical data sets in the world. Wouldn’t a tremendous amount of resources be required to collect the data on which to train in order for any internal machine learning benefit to be realized? Is there any evidence to say that the benefits of administrative data collection would outweigh the cost of the time and labor investment required to accomplish such a task?