One Fish, Two Fish: How Machine Learning Can Enhance Fisheries Management
Overfishing has decimated global fish stocks and threatens food security for billions down the line, but machine learning helps regulators meet this urgent challenge with new capabilities and better science.
Abstract
This paper considers the impact of machine learning on fisheries management in the United States to date, how this technology can help regulators combat overfishing in the future, and finally, offers policy recommendations before posing a set of questions.
Introduction
Overfishing threatens the long-term health of the world’s fisheries and the billions of people they feed. The problem isn’t new — each generation has contributed to the steady decline in global fish stocks — but it has never been more severe. Commercial fishing, a $150 billion dollar industry, has reduced the population of large predatory fish by 90% since 1950, and today, 87% of all fish stocks are fully or overexploited.[1] The ever-growing global demand shows no signs of slowing, driven by the 3.2 billion people who rely on fish for their primary source of protein, and the seemingly endless appetite for high quality seafood in upscale markets.[2] If this continues, fish stocks and the fishing economy could collapse. Effective fisheries management, therefore, is a critical issue.
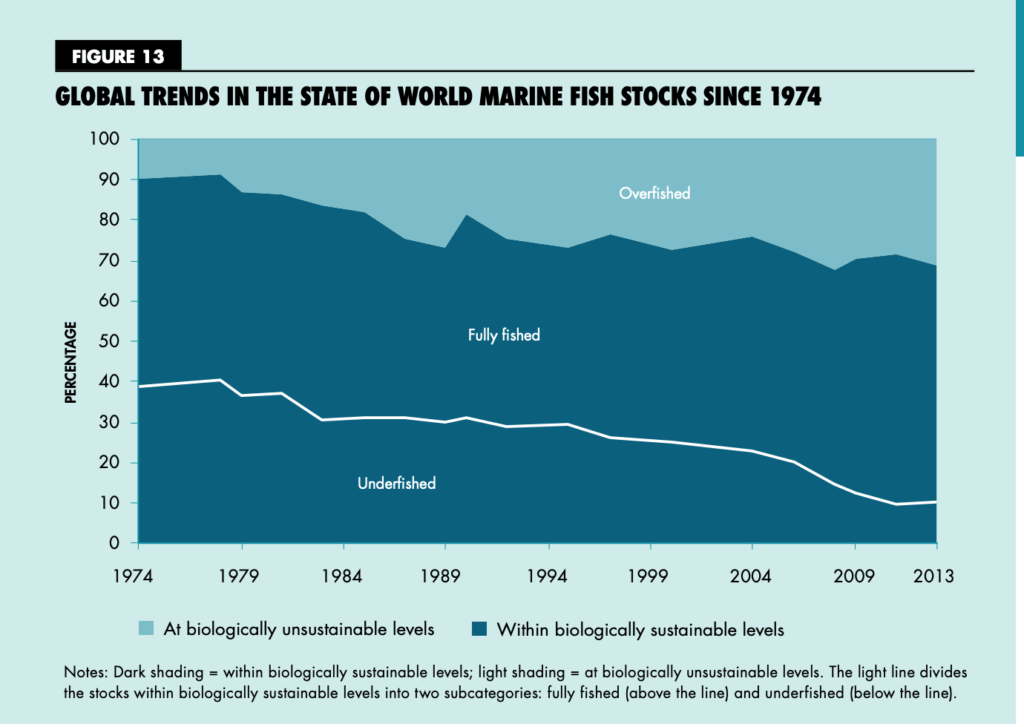
The National Oceanic and Atmospheric Administration (NOAA) manages U.S. fisheries, and a primary obstacle in that effort is a lack of reliable, comprehensive data.[3] This limits their ability to construct models and set sustainable catch limits. Recently, the agency has turned to machine learning (ML) to make sense of otherwise impenetrable data. ML has already demonstrated its utility, and promises to enhance NOAA’s ability to combat overfishing in the future.
Mapping the Global Fleet
Earlier this year, Global Fishing Watch (GFW), a partnership between Google and two non-profit organizations, produced the first ever real-time map of the global fishing fleet. The program relies on two distinct types of machine learning — feature engineering and convolutional neural networks — to process over 22 billion data points (AIS satellite transmissions provided by NOAA).[4] The algorithm can identify ships, their location and speed, and distinguish between different fishing practices.[5] Without ML, processing so much data would have taken a full-time worker 100 years.[6]
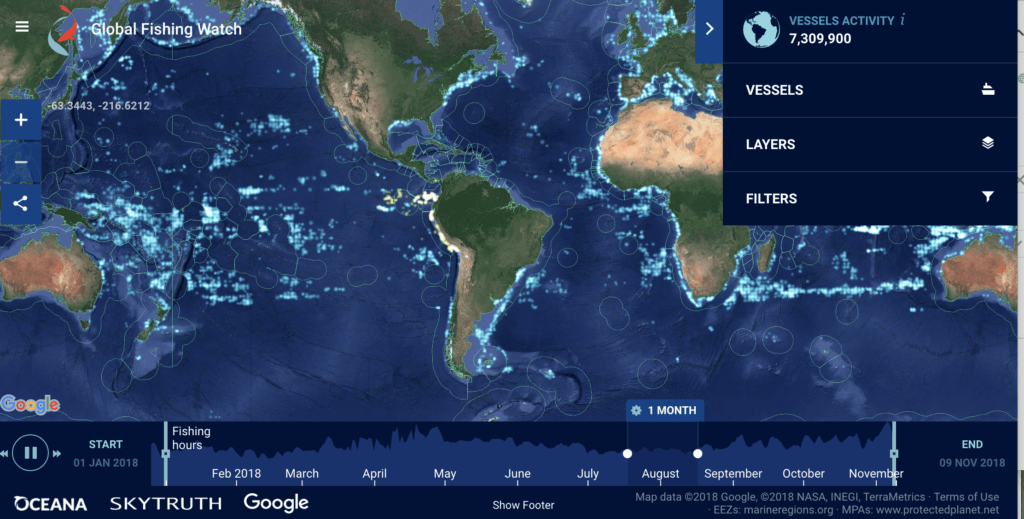
Ultimately, an algorithm is only as good as its data set, and GFW’s is not perfect or complete.[7] For example, the map relies on AIS tracking data, but smaller inland ships do often not have AIS on board, and others turn off or mask their signals to circumvent government regulators.[8] Even if the map is not perfect or entirely comprehensive, it provides NOAA with an entirely new monitoring capability, and demonstrates the transformational potential of ML for fisheries management.
Counting and Identifying Species
No one knows how many fish are in the sea, as they are quite hard to count.[9] Since it began estimating population sizes in 1960, NOAA has relied on methods which require taking fish out of the water in order to count and identify them.[10] This is outdated, inefficient, inaccurate and expensive, but still widely used. [11] New technologies, however, offer a better alternative. Recently, NOAA has deployed new electronic monitoring devices (cameras, acoustic and electronic imaging tools) in pilot programs across the country. The biggest cost associated with each is not hardware, but rather the labor required to analyze the data, so NOAA turned again to ML for help.[12] In 2016, they released data collected from a pilot program in New England, which replaced human observers with cameras.[13] They then co-sponsored an online competition offering a $50,000 cash prize, which generated ML algorithms that counted and identified fish with 100% and 75% accuracy, respectively.[14] Other ML projects have had similar success in identifying species using different visual data inputs.[15]
As digital observation methods become more widespread and the dataset on catch yields expands, ML can help NOAA answer fundamental questions about the state of the ocean and more effectively manage threatened species. Ultimately, however, though this technology holds promise, it is not yet sophisticated enough to provide a true representation of ecological health due to the complexity and overwhelming number of variables at work in any environmental system.[16] To be sure, there is much work to be done before ML is robust enough to accomplish that task.[17]
Recommendations
To harness the power of machine learning to combat overfishing and promote sustainability, NOAA must collect more data and share it widely. To the extent its budget allows, NOAA should (1) deploy new monitoring systems whenever possible, (2) begin crowdsourcing digital images to expand their dataset, (3) promote increased information sharing between agencies, governments and the public, and (4) continue partnering with groups that can provide innovative ML technology. All this will enhance NOAA’s understanding of our ocean and how to protect its natural resources.
Questions
- To what extent might bad actors use more science to further exploit fisheries?
- What can individuals do to combat overfishing?
Word count: 790
Footnotes
[1] [1]United Nation Food and Agriculture Organization, Fisheries and Aquaculture Department, “The State of the World Fisheries and Aquaculture,” (2012). http://www.fao.org/docrep/016/i2727e/i2727e00.htm,
Andrew Revkin, “Commercial Fleets Reduced Big Fish by 90%, Study Finds,” The New York Times, (2003). https://www.nytimes.com/2003/05/15/world/commercial-fleets-reduced-big-fish-by-90-study-says.html
[2] The World Bank, “Brief: Oceans, Fisheries and Coastal Economies,” (2018), http://www.worldbank.org/en/topic/environment/brief/oceans
[3] Adam Allington, “Plenty of Fish in the Sea for Big Data, AI to Tackle,” Bloomberg News, (August, 2018). https://www.bna.com/plenty-fish-sea-n73014481426/
[4] Global Fishing Watch, Research, “What We Do,” (2018). https://globalfishingwatch.org/research/
[5] Ibid.
[6] Ashley Marranzino, “How Machine Learning Reveals the True Costs of High-Seas Fishing,” Massive Science, (2018), https://massivesci.com/articles/international-fishing-management-practices-tracked/
[7] Yeomans. “What every manager should know about machine learning,”. Harvard Business Review Digital Articles (July 7, 2015).
[8] Nicolas Longépé, et al. “Completing fishing monitoring with spaceborne Vessel Detection System (VDS) and Automatic Identification System (AIS) to assess illegal fishing in Indonesia”
Marine Pollution Bulletin, Volume 131, Part B, 2018, pp. 33-39
[9] Nicole Twilley, et al., “How Many Fish Are in the Sea?”, The Atlantic, (October 5, 2016), https://www.theatlantic.com/science/archive/2016/10/how-many-fish-are-in-the-sea/502937/
[10] Ibid.
[11] National Oceanic and Atmospheric Administration, Department of Fisheries, “Developing Machine Vision to Collect More Timely Fisheries Data,” (May, 2018).
Adam Allington, “Plenty of Fish in the Sea for Big Data, AI to Tackle,” Bloomberg News, (August, 2018). https://www.bna.com/plenty-fish-sea-n73014481426/
[12] Meg Wilcox, “The Future of Fishing is Big Data and Artificial Intelligence,” PRI, (May, 2018). https://www.pri.org/stories/2018-05-10/future-fishing-big-data-and-artificial-intelligence
[13] Ibid
[14] Ibid.
[15] Ibid.
[16] Simone Marini, et al, “Tracking Fish Abundance by Underwater Image Recognition,” Nature, (September, 2018).
[17] Ibid.
Given that the current algorithms seem to be able to identify species accurately, I wonder if machine learning could also help scientists identify how fish migration patterns are changing as the oceans warm.
To your point about GFW’s dataset being incomplete – the algorithm is only as effective as the data that it is fed, so if ships continue to refuse to cooperate in certain areas of the world, it could skew the efficacy of the algorithm.
This research is so important and it needs to be thorough and complete so regulatory bodies can act quickly. Fishing populations are being decimated and farming techniques can lead to unhealthy fish stocks that can have an effect on entire ecosystems. I believe consumers can have the biggest impact on this market and they should demand transparency on where their protein was sourced and asked in what manner it was caught. Only by changing the demand dynamics of this equation can we combat “big fish” and try to begin to replenish the global fisheries. From a machine learning aspect, it makes me hopeful that scientists and researchers are using advanced technology to diagnose this problem before it crosses the point where it may be too late to make incremental changes and a large scale, global effort, would be necessary to preserve the fisheries.
The numbers behind the declines in the worldwide fish populations are astounding and do not seem to be slowing down anytime soon. One positive is the increase in the number of fish farms being installed globally. These fish farms also have challenges including pollution from their waste, the spread of diseases from farmed to wild fish, feed requirements (feed generally includes wild fish), and extensive work required to ensure optimal tank conditions. That being said, machine learning is also being used in this field and each year increased funding goes into new innovation. One super interesting company you should check out is SalMar who just installed a farm off the coast of Norway that can hold 1.5m salmon and uses machine learning to measure the real-time activity of the fish (https://www.economist.com/technology-quarterly/2018/03/10/net-gains).
This is a great analysis on the value of machine learning in protecting our fish populations. As you mentioned in your recommendation section, the work that NOAA is doing could also benefit significantly from open innovation. There seems to be a lot of crossover with what Gavin wrote about in his post about Zooniverse. If you have a large group of people who are passionate about this topic and willing to support the agency then opening it up to a large group to help process the digital images could be very powerful. While many of us looked at the major trends in silos, you have identified a topic where machine learning and open innovation can be used simultaneously to magnify the benefit.
Very interesting piece. First, it is clearly a problem that requires the application of ML, I was impressed by your figures of how much time would take a worker to process the data. Definitely, the world cannot wait that long. Second, I really appreciate the fact of how you connected you article to another mega trend: open innovation. Seems like NOAA could also benefit greatly from this cheaper and faster model to innovate.
This is a very interesting topic. On the subject of bad actors, I can see machine learning algorithms directing fishermen to predicted areas of concentration, potentially conflicting with conservation efforts. The use of AIS data to identify the movements of fishing vessels is clever, but as you mentioned AIS is not typically used by small fishing vessels and can easily be turned off. The information provided by this algorithm/monitoring will need to be enforced, however that is costly and potentially impossible in international waters.
Aside from general education, our best chance to help this situation as individuals is through our purchasing and consumption habits. While certain areas of the world require fish in their daily diet, consumption in the West seems more driven by luxury markets and health fads. If we truly value this as a society, we need to start voting with dollars.