Machine Learning & Lending: Banco Santander’s Response to Banking Sector Disruption
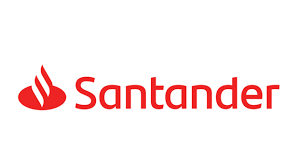
Fintech companies are disrupting the way traditional banks lend money. Banco Santander is too.
The Machine Learning (ML) boom has been a hot topic in the banking sector of late. While it’s already being used by Banco Santander and its competitors for fraud detection and automated customer service [1] [2], companies such as Acorn OakNorth, Lenddo and ZestFinance—tech startups turned darlings of the fintech industry—are contesting the dominance of established banks by applying the technology to a more fundamental element of banking: lending [3] [4] [5].
Applying ML to the lending process offers many potential benefits. ML can increase loan processing speed and decrease administrative costs, by automating time-intensive tasks such as the input of large swaths of document-related data [1]. For example, Acorn OakNorth employs only 14 loan professionals and has lent more than $2.8 billion since 2015; lending this sum would typically require 10x the number of credit assessment staff, according to the company [4]. Kabbage, one of Santander’s fintech investments, can process small business loans in just a few minutes versus several weeks for traditional processes [6].
However, the most salient benefit of ML lies in the technology’s ability to make predictions about credit risk using large unstructured data sets, such as a business or individual’s digital footprint (e.g. mobile phone usage and social media activity) [7]. Incorporating these variables into credit analysis can not only reduce credit losses but can also lead to greater financial inclusion where traditional credit scoring falls short, such as in emerging markets or for low-income borrowers [5].
Banco Santander, recognizing the potential benefits and threats of ML, has taken several steps to incorporate it into its business.
Santander Openbank
In 2016, Santander re-launched its full-service digital bank in Spain—Openbank, relying almost exclusively on ML and Artificial Intelligence (AI) to operate; product offerings, security and fraud detection, and credit risk assessment are all based on these technologies (e.g. mortgage applications and approvals are all done using ML algorithms). The benefits are apparent: Openbank has a million customers, but only one branch and 130 full-time employees. Customers are on average 3x more profitable than Santander’s average retail customers, operating profit at Openbank is higher than at Santander Spain, and the company can provide credit cards to more customers because its algorithm considers more variables impacting creditworthiness. Considering the success of Openbank in Spain and its ML technology, the company is looking to expand the platform to other countries, and incorporate other use cases of the technology, such as robo-advising [2] [8].
Exhibit 1: Openbank vs. Santander Spain Operating Profit, 2017 [2]
Santander InnoVentures
Santander also created a $100 million investment fund called InnoVentures to invest in companies utilizing ML and AI in banking. Several investments offer Santander potential synergies for its core business. SOCURE, a company focused on digital ID verification, uses ML technology to improve online customer authentication [2]. Kabbage, a lender to small businesses in the US, uses online data to provide loans up to $250,000 using a 100% automated system, allowing small businesses to receive loans within minutes [2] [6].
Recommendations
While ML clearly offers many benefits that Santander is already realizing, there are several future opportunities and risks associated with the implementation of the technology that the company should consider.
Expansion into new markets: Santander has already expressed its desire to expand into emerging markets such as Argentina and Chile [2]. However, as internet use becomes more prevalent in frontier markets and banking becomes increasingly borderless, the bank should expand into other lower-income Latin and Central American countries where its ML lending technology can increase financial inclusion.
Test algorithm biases: biases in algorithms are an important source of risk for Santander. One important source of bias relates to the way Santander’s lending algorithms factor in the ethnicity and geographic location of potential borrowers. It is conceivable that as the algorithms learn, they end up discriminating against certain groups of borrowers [5]. To avoid such situations, Santander should continue to maintain an adequate level of human involvement at Openbank to monitor whether these biases become apparent and actively eliminate them to ensure broader financial inclusion and prevent discrimination.
Regulatory collaboration: given the sensitivity around using public and private data of potential borrowers to decide who should receive loan funding, Santander should increase its collaboration with financial sector regulators, to ensure full compliance with privacy laws. As one of the pioneers among the large global banks using ML technology, Santander has an opportunity to play a leadership role in data privacy protection as the technology becomes increasingly utilized for lending decisions.
Going forward, I would be curious to find out whether:
- ML algorithms using multiple online datasets actually result in lower credit losses, and
- How ML lending might differ at different points in the business cycle.
Word Count: 784
Sources:
[1] “Unshackled algorithms: Machine-learning promises to shake up large swathes of finance,” The Economist, May 25, 2017, https://www.economist.com/finance-and-economics/2017/05/25/machine-learning-promises-to-shake-up-large-swathes-of-finance, accessed November 2018.
[2] JP Morgan Cazenove, “Santander: AI, machine learning and robotics transforming the
bank – stay OW,” March 12, 2018, via ABI/ProQuest, accessed November 2018.
[3] Laura Noonan, “AI in Banking: the reality behind the hype,” Financial Times, April 12, 2018, https://www.ft.com/content/b497a134-2d21-11e8-a34a-7e7563b0b0f4, accessed November 2018.
[4] Martin Arnold, “British digital lender valued at $2.3bn after fundraising,” Financial Times, September 5, 2018, https://www.ft.com/content/b8506b2e-b126-11e8-8d14-6f049d06439c, accessed November 2018.
[5] Emily Bary, “How artificial intelligence could replace credit scores and reshape how we get loans,” MarketWatch, October 29, 2018, https://www.marketwatch.com/story/ai-based-credit-scores-will-soon-give-one-billion-people-access-to-banking-services-2018-10-09, accessed November 2018.
[6] Crunchbase, “Kabbage Company Overview,” https://www.crunchbase.com/organization/kabbage#section-overview, accessed November 2018.
[7] Bill Byrnes, Suresh Baral, “Can Machine Learning Improve Consumer Lending? We Think So.,” Protiviti (blog), Protiviti Company Website, August 1, 2018, https://blog.protiviti.com/2018/08/01/can-machine-learning-improve-consumer-lending-think/, accessed November 2018.
[8] Santander, 2017 Annual Report, p. 2, http://www.santanderannualreport.com/2017/sites/default/files/openbank_0.pdf, accessed November 2018.
Excellent piece on Machine Learning in the Financial Services Industry! I agree with your assessment that banks need to ensure that their machine learning algorithms are not violating fair lending laws with any type of implicit discrimination; an additional consideration would be whether to allow machine learning algorithms to “experience” all types of loan outcomes, so as to build better decision making capabilities in the future. In other words, should Santander temporarily accept all loan applications, knowing they would incur higher losses in the immediate future, so that their machine learning capabilities can learn what a good application looks like, potentially improving longer term financial performance? The alternative, is to start by configuring the algorithm according to its current underwriting standards, which may risk incorporating Santander’s own specific biases. Small business lending startups have taken the approach of initially accepting a very broad pool and found success in relatively low loss rates as the algorithms adjust, after a brief period of high losses.
Interesting! I have a tough time thinking about large financial institutions making these types of loans using machine learning without human involvement given their fiduciary obligations as financial institutions. This is why I think startups have had such traction, and why the idea to connect to regulatory bodies makes so much sense. We spoke about the idea of “garbage in, garbage out” in class, so I think multiple data sets would be best. Very interested to see where they continue to expand with this product – doing it in-house or building via acquisitions.