Machine Learning in the Shale Patch: A Look at the “Mother Fracker”
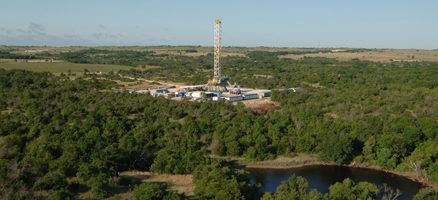
“The energy patch is ripe for opportunities related to things like predictive analytics, machine learning, artificial intelligence projects” – Tim Dove, Pioneer Natural Resources CEO (June 2017) [1]
Reports of U.S. oil supply’s death have been greatly exaggerated. Technological breakthroughs combining horizontal drilling and hydraulic fracturing in the mid-2000s brought about a production boom. Oil production increased from 5 million barrels per day in 2008 to nearly 12 million barrels today [2]. For much of this time, oil prices hovered near $100 per barrel, resulting in healthy economics for shale producers. However, the supply-driven crash beginning in late 2014 caused oil prices to crater below $30 per barrel, leading U.S. shale companies to evaluate new levers to cut costs and survive.
Pioneer Natural Resources, dubbed the “mother fracker” by noted investor David Einhorn given its prominence in the U.S. shale industry, is at the forefront of applying technology in the shale patch to drive efficiencies [3]. Notably, Pioneer cites machine learning as one of its key drivers to hit its publicly-stated goal of producing 1 million equivalent barrels per day in the next decade (vs. slightly over 300,000 equivalent barrels per day currently) [4]. With reams of data produced every moment from its nearly 11,000 producing wells, Pioneer represents a test case for how machine learning can benefit shale producers [5].
Pioneer’s Approach to Date
Pioneer has moved aggressively to capitalize on the potential of applying machine learning compared to its peers. As evidence, Pioneer appointed a Chief Technology Officer (CTO) in May 2017, a rare title for an oil and gas company [6]. The company has taken the approach of partnering with service providers and labs to analyze the large datasets its wells produce, though Pioneer is in the process of building its in-house analytics capabilities.
One area in which Pioneer has found success in applying machine learning is in predictive maintenance. Pioneer operates thousands of wells in often remote areas of Texas. It previously used low tech methods of having workers in the field monitor wells manually but has recently shifted towards using predictive analytics on production data to predict when a well will require maintenance. By doing so, Pioneer “can shut the [well] down and solve what may have been a multi-million-dollar problem thirty minutes before it happens”, according to Pioneer’s CEO [7].
Though predictive maintenance is just the beginning of applying machine learning for Pioneer, it is believed to have great potential across the oil and gas sector. A recent study by Wood Mackenzie, a leading energy consultant, estimates that by placing the U.S. Lower 48’s mature wells on smart production management systems, Lease Operating Expenses (LOE) could lower by 10%, creating $25 billion of value [8]. A McKinsey study analyzing offshore production (note: Pioneer operates onshore) found that a typical offshore platform runs at ~77% of its maximum production potential, resulting in downtime costing ~$200 billion in annual revenue [9].
What Pioneer Needs to Do Going Forward
Though early days, Pioneer has had some mixed success with machine learning results. Research aimed at predicting well performance showed that the model worked well when using data from 1,000 wells, but it ran into challenges when using a subset of 400 wells that tapped into a specific rock layer [10].
One potential way to address this issue is to partner with other shale producers to share data and collaborate on data science efforts. Most shale producers like to safe guard much of their data in-house given potential competition in acquiring adjacent land. However, as the land grab from the early days of U.S. shale has mostly played out, operators may now have less sensitivity to sharing completion and production data with one another. Given the commodity business is not “zero sum”, regional shale operators could benefit from learning best practices from each other.
A second recommendation is to work with third-party data analytics firms to speed up the process while Pioneer is building out an internal team. Analytics firms have identified oil and gas as a space where machine learning can be very effective due to significant data generation. One such firm, C3 IoT, believes that IoT analytics have the potential in the oil and gas sector to “unlock billions of dollars in improved operating revenues and reduced costs per year” [11].
Considerations for the Shale Industry
- Are shale companies overstating the potential of machine learning in the oil patch? Do the improvements in well uptime amount to “singles and doubles” or can they differentiate producers operating in a commodity market?
- Should shale companies “double down” on this trend by investing capital in data analytics and hiring data scientists? Should they instead outsource to third-party data analytics providers to maintain lower headcount?
(759 Words)
References
[1]. Dove, T. (2017). Pioneer Natural Resources Third Quarter 2017 Earnings Call.
[2]. “Weekly U.S. Field Production Of Crude Oil (Thousand Barrels Per Day)”. 2018. Eia.Gov. https://www.eia.gov/dnav/pet/hist/LeafHandler.ashx?n=pet&s=wcrfpus2&f=w.
[3]. “Why David Einhorn Is Shorting The ‘Mother Fracker’ And Other Oil Companies”. 2015. Fortune. http://fortune.com/2015/05/04/2015-sohn-conference-einhorn-shorting-mother-fracker-oil-companies/.
[4]. “Pioneer Natural Resources Company Reports Third Quarter 2018 Financial And Operating Results | Pioneer Natural Resources Company”. 2018. Pioneer Natural Resources Company. http://investors.pxd.com/news-releases/news-release-details/pioneer-natural-resources-company-reports-third-quarter-2018.
[5]. Pioneer Natural Resources. Form 10-K, 2018. Page 43
[6]. Pioneer Natural Resources. Form 10-K, 2018. Page 45
[7]. “Why The CEO Of Pioneer Natural Resources Is Mining His Own Business”. 2017. Bizjournals.Com. https://www.bizjournals.com/dallas/news/2017/10/23/why-the-ceo-of-pioneer-natural-resources-is-mining.html.
[8]. “Digitalization Can Save Upstream Sector $75B Each Year”. 2018. Rigzone.Com. https://www.rigzone.com/news/digitalization_can_save_upstream_sector_75b_each_year-12-nov-2018-157454-article/.
[9]. “Why Oil And Gas Companies Must Act On Analytics”. 2017. Mckinsey & Company. https://www.mckinsey.com/industries/oil-and-gas/our-insights/why-oil-and-gas-companies-must-act-on-analytics.
[10]. “Pioneer’s Analytics Project Reveals The Good And Bad Of Machine Learning”. 2018. Spe.Org. https://www.spe.org/en/jpt/jpt-article-detail/?art=4439.
[11]. “From Digital Disruption To Digital Transformation | C3”. 2018. C3. https://c3.ai/industries/#industry-oil-gas.
This article provides a thoughtful examination of an industry that is ripe with recent technological advances. Making money in the oil industry was a heck of a lot easier when oil prices are $100/barrel. These prices led enough new players into the space to create a surplus of production. With the drastic price drop in 2014, oil producers were forced to focus on efficiency improvements and cost cutting. As horizontal drilling and fracking technologies continue to improve, the lowest cost provider will have a serious competitive advantage.
It will be interesting to see just how big of a role machine learning can play in this lower priced environment. As Mark suggested, perhaps the larger players with the most data will be the companies that have the most to gain from machine learning. Pioneer seems to be one such company that could see large benefits from the technology.
As I read this article, I began to see even how the application of this technology can be extended from maintenance into production optimization. Rather than rely on humans to observe well performance on a day-to-day basis, and decide on whether to make operational changes like beaning up/down wells or increasing gas-lift rates if applicable, the same mathematical models used by operators, production technologists and reservoir engineers can be coded into the machine learning algorithm such that, on a real-time basis, the algorithm can ‘decide’ on the right course of action and execute by sending appropriate control signals to the wells. This will free up time for humans to focus on more critical or strategic tasks.