I, Banker
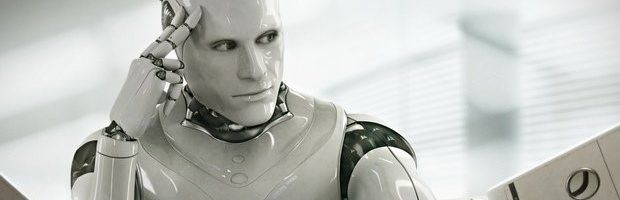
JP Morgan, Machine Learning, and the Future of Finance
Introduction
Isaac Asimov’s 1950 novel I, Robot posits a future shared, uneasily, by humans and sentient robots. As machine learning becomes more ubiquitous, it seems that world may no longer be one of science-fiction. Machine learning programs’ abilities to analyze, collect, and aggregate data have had profound impacts on our world, extending to the banking and financial services industries.[1] Many firms have sought to bolster the security of their online banking platforms by using machine learning to identify data patterns indicative of fraudulent activities.[2] Programs built on machine learning are also much more efficient than human analysts at pulling relevant information from digital financial statements.[3] Machine learning programs have also been employed to automate short-term trading functions, drastically reducing the number of human traders and time required to execute trades.[4] Goldman Sachs has even begun using machine learning to automate certain parts of its IPO process such as compliance assessment, staffing, and organizational book creation that previously took thousands of analyst- and associate-hours to accomplish.[5,6] While many of these applications are yet inchoate, it is already apparent that firms must embrace and adapt to the implications of machine learning to stay competitive.
JPMorgan and Machine Learning
JPMorgan has devoted more time and treasure than any other bulge bracket bank to incorporating machine learning into its banking, asset management, and investment banking operations – over $10 billion a year spent on tech and over 50,000 employed technologists.[7] Notable developments include a “contract intelligence platform (CoIN)” that uses “machine learning to analyze legal documents and extract important data points and clauses”.[8]
Looking at the longer-term, JPMorgan has also instituted mandatory coding training for all the analysts in its asset management division to enable them to better use machine learning programs in their analysis.[9] JPMorgan also established a “center of excellence” in 2016 to “explore and implement a growing number of use cases for machine learning applications across the firm”.[10] Along with an imposing study entitled “Big Data and AI Strategies: Machine Learning and Alternative Data Approach to Investing” in 2017, this group produced one of the first predictive machine learning applications for investment banking, the “Emerging Opportunities Engine” that predicts if a company is positioned to issue additional equity.[11]
Despite this success, it is unclear if JPMorgan will extend coding training to the corporate and investment banking division next year.[12] Investment banking predictive applications for machine learning remain scarce, largely because experts believe utility is limited since current methods are unable to compete with the intuition and judgment of savvy humans over the mid- to long-term.[13]
Recommendations
While it is true that algorithms can approximate but never replicate human intuition and judgment, opportunities still exist to leverage machine learning in investment banking. In terms of process improvement, JPMorgan can emulate Goldman Sachs in automating many of the initial stages of its IPO and merger and acquisition (M&A) processes – utilizing processes already present in their CoIN program. Like CoIN, such a program could save many thousands of hours of human labor and materially reduce process time and costs.
JPMorgan can also continue pursuing applications for ‘Deep Learning’ posited by their “Big Data” study. The study identified that a ‘Deep Learning’ method known as Convolutional Neural Nets (CNNs) could be trained to effectively identify and classify complex technical patterns.[14] When coupled with another ‘Deep Learning’ method known as Reinforcement Learning, already used in the Emerging Opportunities Engine, these CNNs could be of particular use in predicting market conditions in the mid-term to identify the optimal timing for an IPO.[15] The study classifies these methods as “especially promising” because they continually refine and improve themselves against existing data, auguring increased efficacy given expanding and closely-curated data sets.[16]
A final recommendation returns to training. The “Big Data” study identified “employing data scientists who lack specific financial experience or financial intuition” as a significant source of risk in adopting machine learning in finance.[17] JPMorgan has shown a willingness to train its analysts and associates in coding – it may be more beneficial to incorporate instruction on financial principles from the analyst on-boarding program into that used for data scientists. Because of the esoteric nature of machine learning, especially ‘Deep Learning’, the discovery of breakthrough applications will depend not on analysts with an understanding of coding, but on data scientists who understand finance and the data on which those applications must be founded.
Conclusion
Despite their great potential in all areas of finance including investment banking, machine learning programs as we understand them cannot mimic human judgment and expert intuition. Therefore, it remains true that investment bankers need not fear the machines, as in Asimov’s dystopian future. However, do the bankers now have cause to fear financially-savvy data scientists?
(799 words)
Endnotes
[1] H.J. Wilson, A. Alter, and S. Sachdev, “How Companies are Using Machine Learning to Get Faster and
More Efficient,” Harvard Business Review, May 3, 2016, https://hbr.org/2016/05/how-companies-are-using-machine-learning-to-get-faster-and-more-efficient, accessed November 2018.
[2] Tom Groenfeldt, “Citi Ventures Deploys Machine Learning and Artificial Intelligence with People,”
Forbes, October 31, 2016, https://www.forbes.com/sites/tomgroenfeldt/2016/10/31/citi-ventures-deploys-machine-learning-and-artificial-intelligence-with-people/#6df26ce65268, accessed November 2018.
[3] Laura Noonan, “JPMorgan’s requirement for new staff: coding lessons,” Financial Times, October 7,
2018, https://www.ft.com/content/4c17d6ce-c8b2-11e8-ba8f-ee390057b8c9, accessed November 2018.
[4] Nanette Byrnes, “As Goldman Embraces Automation, Even the Masters of the Universe are
Threatened,” MIT Technology Review, February 7, 2017, https://www.technologyreview.com/s/603431/as-goldman-embraces-automation-even-the-masters-of-the-universe-are-threatened/, accessed November 2018.
[5] Dakin Campbell, “Goldman Set Out to Automate IPOs and It Has Come Far, Really Fast,” Bloomberg
Digital Article, June 13, 2017, https://www.bloomberg.com/news/articles/2017-06-13/goldman-set-out-to-automate-ipos-and-it-s-come-far-really-fast, accessed November 2018.
[6] Raul Hernandez, “Goldman Sachs found a way to automate dealmaking tasks usually managed by
investment bankers,” Business Insider Digital Articles, June 13, 2017, http://www.businessinsider.com/goldman-sachs-found-a-way-to-automate-dealmaking-tasks-usually-managed-by-investment-bankers-2017-6, accessed November 2018.
[7] Noonan, “JPMorgan’s requirement for new staff: coding lessons”.
[8] JPMorgan Chase & Co, 2016 Annual Report, https://www.jpmorganchase.com/corporate/annual-report/2016/ar-ceo-letter-matt-zames.htm, accessed November 2018.
[9] Noonan, “JPMorgan’s requirement for new staff: coding lessons”.
[10] JPMorgan Chase & Co, 2016 Annual Report
[11] Ibid.
[12] Noonan, “JPMorgan’s requirement for new staff: coding lessons”.
[13] Marko Kolanovich and Rajesh T. Krishnamachari, “Big Data and AI Strategies: Machine Learning and
Alternative Data Approach to Investing,” JPMorgan & Chase Co., May 18, 2017, p. 8.
[14] Ibid., p. 112.
[15] Ibid., p. 114.
[16] Ibid.
[17] Ibid.
It will definitely be interesting to see whether JP Morgan extends their coding training in the coming years. The trend of increasing analytics training for more ‘traditional’ hires is something we see in the consulting industry as well, so it’s not surprising that it’s happening in banking as well. Yet I do wonder what the benefits are of providing traditional banking hires with coding training, as opposed to hiring data scientists specifically. I imagine that it would be very difficult to train a banker up to the level of a data scientist and vice versa, so I’m curious as to whether they continue to cross-train both, or if they find a more synergistic way to have those two different functions work more collaboratively together.
“Thousands of analyst- and associate-hours to accomplish”… that one definitely struck home. You’ve done a fantastic job drilling down to the roots of what financial transactions entail and how machine learning can disintermediate many of the roles within the traditional chain-of-command in banking. However, I have to disagree on the intrinsic value of expert intuition. From my experience in the banking, tech and entertainment worlds, ‘intuition’ is frequently just subconscious pattern recognition, which is notoriously unreliable, especially as future outcomes diverge further and further away from past trends. Ultimately, most of the higher-level banking positions (i.e. managing directors) essentially spend their days relationship-building (i.e. wining and dining clients) to win business. In other words, not adding value but ingratiating themselves with corporate decision makers. Expanding on your thesis, I do believe a two-person shop (one with finance theory expertise, one with data science expertise) combined with powerful ML could prove to be the next, and superior, iteration of what financial advisory looks like tomorrow.
Definitely agree with you that the best next iteration will be a blend of human expertise and appropriately-crafted ML. I may have chosen the wrong word in “intuition” to describe what humans have that ML programs lack to date. It seems that one of the biggest limitations of ML, for now, is it cannot – to use an in-vogue section phrase – “see around corners” (predicting the effects of regime changes, political trends, zeitgeists, thorny hypotheticals, etc). Humans still hold the upper hand here with regard to long-term predictions, but can still greatly benefit from the partnership with ML.