How Coca Cola is Leveraging Machine Learning in the Hyper-competitive CPG Industry
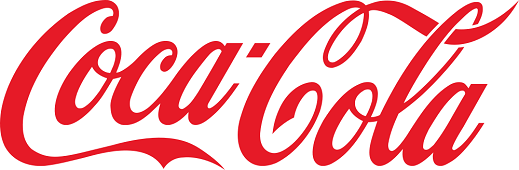
Major shifts are occurring in the food & beverage industry as consumers look beyond legacy CPG brands and towards new, interesting, and perceived healthier alternatives1. As these customer desires continue to intensify, it is critically important for the larger companies to drill-down their focus to the consumer level. Coca Cola is embodying this strategy by utilizing machine learning to personalize its product development and enhance its placement and delivery. It is drastically important for Coca Cola to leverage its power of scale through substantial investments in this megatrend so that it can thrive in the new era of the food and beverage industry.
In the short term, Coca Cola is leveraging machine learning heavily in its product development. One example is the launch of Cherry Sprite2. The company first debuted its interactive soda fountain dispensaries in 2009 where restaurant and retail guests could add a splash of different flavors to their favorite Coca Cola drinks3. The company cleverly collected the data of the vast flavor combinations and leveraged machine learning to identify the most popular versions. Using the analysis, Coca Cola was able to identify a large market for Cherry Sprite and launched the product nationally. Leveraging machine learning, the company was able to bring product development to the consumer level and make decisions based on their individual preferences.
In addition to product development, Coca Cola is also utilizing machine learning in the short term for product delivery. Specifically, it is upgrading consumer interaction with its vending machines4. The company is doing so by finding new patterns in foot traffic and demand to best locate its machines for maximum utility. On top of that, it is also fine tuning each unit. Utilizing machine learning to analyze purchasing behavior and customer data, the vending machines will be able promote appropriate products for that area and offer discounts related to that specific unit. Additionally, the machine will also be able to adjust its “mood” to the setting (e.g., promoting more water if it is in a gym). By leveraging this technology, Coca Cola is further enhancing the personalization of its product placement.
Long term, Coca Cola is looking to use machine learning to improve its placement in brick-and-mortar retail5. The company historically managed a very labor-intensive process for its store-level audits; the procedure used to both understand how its goods are placed on the shelf next to competitors and determine any out-of-stock items that needed to be replenished. To avoid the tedious and costly process, Coca Cola invested in a machine learning technology to analyze images of shelves taken via a mobile phone. By leveraging this new technology, the company could conduct corporate-level marketplace surveys six times a year instead of twice. Looking forward, this machine learning mechanism will only prove more important as competition on the shelf becomes even more fragmented. Coca Cola must be able to react to developments rapidly and this technology will enable the company to be nimbler and better equipped to fend off its competition.
Although Coca Cola has made great strides at leveraging machine learning in several areas so far, there is still more to do to ensure it is maximizing its opportunity. In the short term, the company must continue to focus on product development at the consumer-level. Although Coca Cola has done so in terms of new flavor combinations for existing products, the company should also think beyond line extensions and leverage the machine learning data to also develop new and innovative brands. By doing so, Coca Cola will be better able to compete with new entrants to the food & beverage market that are currently winning by delivering new solutions.
Over the longer term, Coca Cola should continue to streamline its supply chain. The company has made great strides at developing a more cost-effective manner to evaluate its distribution channel. It should now leverage that process and incorporate machine learning further upstream. Specifically, the company should use its learnings at the store level to better manage its production scheduling and raw material purchases. By doing so, it can increase its profitability and drive value to the P&L not only via top-line improvements but also deductions in cost of goods sold. Additionally, by better managing its inventory, Coca Cola will be in a better cash flow position and will have more capital available to invest back into its machine learning project.
Coca Cola has made great progress in utilizing machine learning to personalize its product development and enhance its product placement. However, the food & beverage industry is becoming increasingly competitive each day. With competition rising and industry margins already slim, will Coca Cola continue to allocate adequate investment dollars into machine learning for the long-term?
(word count 782)
Sources:
1 Back, Aaron. “Food Companies Can’t Figure Out What Americans Want to Eat.” The Wall Street Journal, Dow Jones & Company, 5 June 2018, www.wsj.com/articles/food-companies-cant-figure-out-what-americans-want-to-eat-1528200000.
2 Marr, Bernard. “The Amazing Ways Coca Cola Uses Artificial Intelligence And Big Data To Drive Success.” Forbes, 18 Sept. 2017, www.forbes.com/sites/bernardmarr/2017/09/18/the-amazing-ways-coca-cola-uses-artificial-intelligence-ai-and-big-data-to-drive-success/#4e8cd56078d2.
3 Moye, Jay. “Sprite Cherry and Sprite Cherry Zero Are First National Brands Inspired by Coca-Cola Freestyle.” The Coca-Cola Company, 13 Feb. 2017, www.coca-colacompany.com/stories/fountain-favorites-sprite-cherry-and-sprite-cherry-zero-become-first-national-brands-inspired-by-coca-cola-freestyle.
4 “Coca-Cola Is Using AI to Put Some Fizz in Its Vending Machines.” Digital Food & Beverage 2019, WBR Insights, foodandbeverage.wbresearch.com/coca-cola-artificial-intelligence-ai-omnichannel-strategy-ty-u.
5 Cheng, Andria. “CPG Brands Are Leveling The Playing Field By Bringing This Digital Tool Into Brick-And-Mortar Retail.” Forbes, 31 May 2018, www.forbes.com/sites/andriacheng/2018/05/31/one-new-area-cpg-brands-are-leveling-the-playing-field-against-online-retailers/#79293b543abb.
The power of consumer shopping data pre-purchase has been huge for online retailers. Being able to see what shoppers browsed but didn’t purchase, and how they compare various products, etc. Is there a way Coca-Cola can match or emulate this type of data collection for retail? While the mobile pictures collection makes sense, it is still orders of magnitude away from what online retailers collect. Would a combination of facial recognition / customer tracking video software and brick and mortar retail partnership allow Coca-Cola to see which of their products customers consider, but don’t purchase? Given the level of investment this would require, what would have to be true / what would Coca-Cola management need to believe for this to be value accretive?
Great examination of Coca Cola’s ability to integrate machine learning into many levels of its business- product development, distribution, promotion and supply chain management. I did, however, want to raise a couple concerns with the author’s recommendations:
1. I’m not entirely sure that I agree that machine learning can help Coca Cola with product innovation. Certainly, data science and analysis is crucial for Coca Cola to understand consumer preferences and trends. However, to say that it can help with product innovation means that it can identify different tastes that consumers find appealing and correctly assert that they could be combined together. While that is possible, that would rely on a complex understanding of biology, taste science or at least data from other culinary sources or databases. I think, instead, this author is merely asserting that investing in a strong data science platform and team is crucial to Coca Cola’s ability to continue to develop products consumers love. That is something I wholeheartedly agree with.
2. I certainly agree with the author that using machine learning to predict customer behavior and downstream demand for its product could help Coca Cola distribute its product more efficiently, place its vending machines more selectively and even incorporate location-specific advertising in its vending machines. One question I have is what infrastructure is necessary for this to happen. My understanding is that the vast majority of vending machines are not owned by beverage or snack companies, but by vending machine businesses. Would simply adding a few of their own vending machines provide Coca Cola with the scale to achieve their ambitions?
3. Lastly, Coca Cola is an enormous company with many different products in its portfolio. Do you view these innovative machine learning solutions to be something that Coca Cola should implement for all of its products, or simply those with huge scale, like Coke and Sprite?
Best,
Gabe
While Coca Cola has been using machine learning for both innovation and supply chain, I think there is likely more potential for value from improving the efficiency of their supply chain. I was surprised to read that the machine learning technology being used in retailers was actually still quite manual – physical store checks with photos, which would require a lot of resources to scale globally. Given the expansive point of sale information that can be collected from retailers today, I wonder what Coca Cola is doing to leverage and learn from their selling behaviors. Perhaps a middle ground that would not require as many investment dollars would be to leverage POS data and machine learning to better optimize their supply chain management and regionalization of product assortment.
This article presents a great way of how Coca Cola has benefited from machine learning in several aspects of the business, from product development to shelf layout at retail stores. It is clear how the development of the Cherry Sprite was the result of carefully analyzing consumer patterns at fountain dispensaries, however the author goes as far as to say that machine learning could potentially help Coca Cola create new and innovative brands. It is not clear to me how they could develop new brands using the current information available as I believe the value of the information collected at fountain dispensaries has limited value.