Fashion Forward – using deep learning at ASOS to predict customer lifetime value in online fashion retail
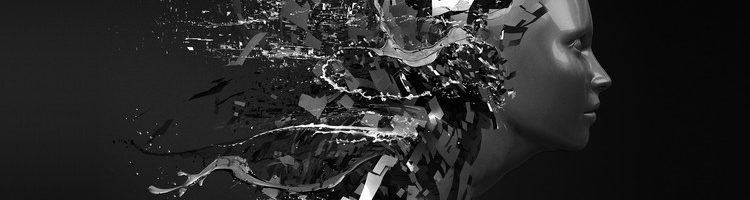
ASOS is using deep learning neural networks to predict customer lifetime value based on online shopping behavior.
I explore the impact that deep learning neural networks have had on the process of forecasting customer lifetime value (CLV) in online fashion retail (retail) using current applications at the online fashion retailer ASOS[1]. CLV is a key marketing metric that assigns future profitability to a customer based on three inputs: marginal value, churn rate, and acquisition cost[2].
In OFR, correctly predicting CLVs is a competitive advantage because of the significant negative impact that unprofitable customers have on a business’ bottom line. There are two ways in which deep learning is improving profitability at OFRs. First, deep learning reduces the quantities of orders from negative value customers by reducing marketing dollars targeted at this customer segment, which increases marginal value. Second, deep learning reduces the churn rate for customers, which increases their average lifetime with the retailer.
The business of online fashion retail is predicated on customers being able to try on clothes at home and return the items that they do not want[3]. For example, in an interview with one ASOS customer, the customer indicated that he had purchased eleven pairs of jeans so that he could find a perfectly fitting pair. However, out of the eleven items, this customer returned eight and kept only three. While this might seem shocking, this is not an atypical purchasing pattern at ASOS. This problem can be exacerbated by free shipping thresholds, which encourage some customers to purchase up to that spend level. Then subsequently return the filler items having received the free shipment discount.

These kinds of customers tend to be negative value customers for ASOS. Customers with negative values are the bane of OFRs because the costs free shipping and returns are borne by the retailer. According to CBInsights, “beyond lost revenue, returns are expensive to process. Each time an item is returned, retailers often pay for its return-shipping, re-sorting, and re-shelving”[4],[5].
At ASOS, deep learning neural networks can identify the behaviors of high value customers, who tend to shop for undiscounted items, in-season items, and more unique pieces. In addition to the items that they look at high-value customers tend to look at different products at different times in a different sequence than low-value customers. Deep learning is then able to assign an intention to purchase and the value of that intention to a given customer given their behavior on the website[6]. This means that ASOS can offer unique promotions to a high-value customer to encourage a purchase.

In addition to predicting intention to purchase and value of the purchase for a given customer deep learning neural networks are also used to predict the likelihood of a customer churning. Prior to application of machine learning, it was challenging to identify customers at-risk of ending their relationship with the OFR prior to them ending the relationship. Now with the implementation of deep learning, a retailer like ASOS can implement retention strategies[7] for high value customers who might be considering taking their business elsewhere. Anecdotally, this is incredibly powerfully because it is generally accepted that it is harder to win back an old customer than to maintain a current customer.
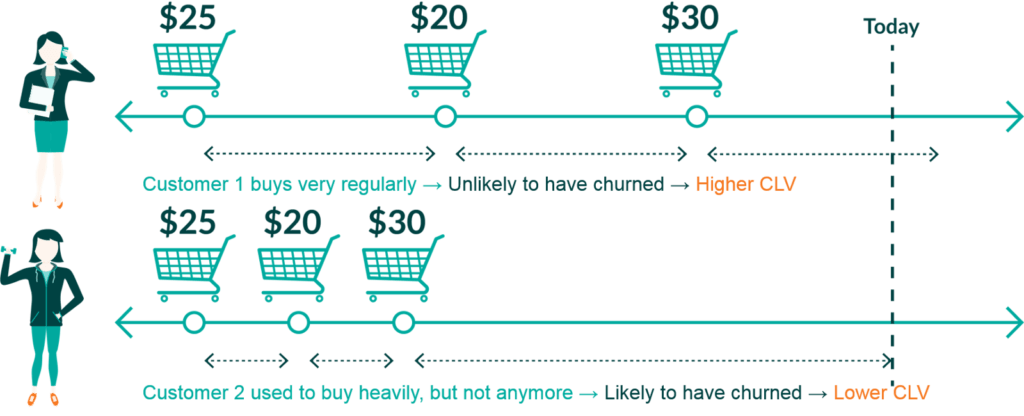
However, going forward, ASOS should consider actively divesting unprofitable customers similar to Net-a-Porter, which “retained the right to refuse returns or close accounts if the level of returns was deemed excessive” [8]. This is important because unprofitable customers redirect valuable resources that should be dedicated towards profitable customers. Should ASOS fire its unprofitable customers and if it does – how should it execute this strategy? More importantly, how should ASOS integrate customer style and size recommendations? Could there be privacy and security concerns and how should the business think about mitigating these concerns?
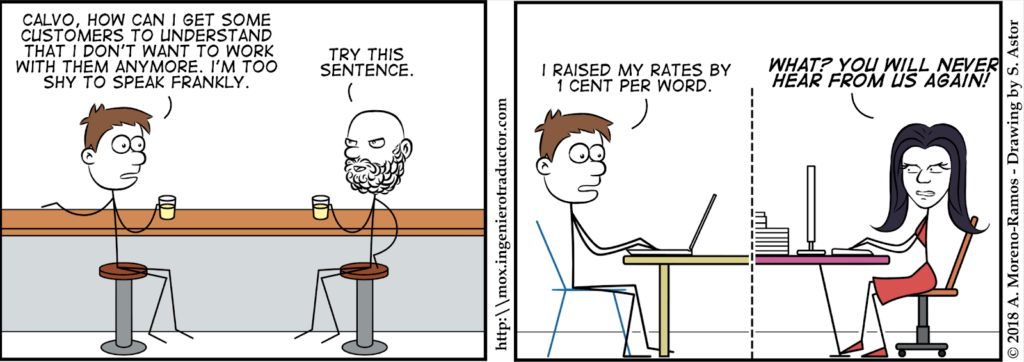
[Word count = 787]
Endnotes
[1] ASOS Plc, together with its subsidiaries, operates as an online fashion retailer in the United Kingdom, the United States, Australia, France, Germany, Spain, Italy, Sweden, the Netherlands, and Russia. The company offers womenswear, menswear, and sportswear products. It sells approximately 85,000 branded and ASOS brand products primarily through its website, asos.com, as well as through social media platforms and magazines. The company is also involved in marketing staff employment and payment processing businesses. ASOS Plc was founded in 2000 and is headquartered in London, the United Kingdom (CapitalIQ).
[2] (Steenburgh & Avery, 2017)
[3] Returns cost retailers $260 billion in 2015, according to the National Retail Federation. And about 30 percent of items bought online end up being returned, versus 9 percent of items bought in stores (Quenqua, 2017).
[4] (CBInsights, 2018)
[5] (Dennis, 2018)
[6] (Chamberlain, Cardoso, Liu, Pagliari, & Deisenroth, 2017)
[7] (Fridrich, 2017)
[8] (Wells & Ellsworth, 2018)
References
Agrawal, A., Gans, J., & Avi, G. (2016, November 17). The Simple Economics of Machine Intelligence. Retrieved from Harvard Business Review: https://hbr.org/2016/11/the-simple-economics-of-machine-intelligence
Andersson, T. (2017, July 21). Deep Learning & Fashion Imagery. Retrieved from LinkedIn: https://www.linkedin.com/pulse/deep-learning-fashion-imagery-thomas-andersson/
Andrew, N. (2017, July 18). A simple way to understand machine learning vs deep learning. Retrieved from Zendesk: https://www.zendesk.com/blog/machine-learning-and-deep-learning/
ASOS Plc. (2018, October 17). 2018 Earnings Call. Retrieved from CapitalIQ: https://www-capitaliq-com.ezp-prod1.hul.harvard.edu/CIQDotNet/Transcripts/Summary.aspx?CompanyId=9237218
ASOS Plc. (2018). Annual Report.
Berzgal, G. (n.d.). Returns in online retail: How to use it as a differentiator in your offering. Retrieved from IMRG (UK’s online retail association): https://www.imrg.org/blog/returns-in-online-retail-differentiator-in-offering/
Bohnhoff, T. (2016). E-commerce: Fashion. Hamburg: Statista.
CBInsights. (2018, November 8). 5 Startups Solving One Of Retail’s Major Pain Points: Returns. Retrieved from CBInsights: https://www.cbinsights.com/research/startup-retailer-returns-expert-intelligence/
CBInsights. (2018, April 3). Retail’s Adapt-Or-Die Moment: How Artificial Intelligence Is Reshaping Commerce. Retrieved from CBInsights: https://www.cbinsights.com/research/artificial-intelligence-reshaping-commerce/
Chamberlain, B. (2017). Deep Learning in Retail & Advertising Summit, London. (A. Cuthbertson, Interviewer)
Chamberlain, B. P., Cardoso, A., Liu, B. C., Pagliari, R., & Deisenroth, M. P. (2017). Customer Lifetime Value Prediction Using Embeddings. Proceedings of the 23rd ACM SIGKDD International Conference on Knowledge Discovery and Data Mining, 1753-1762.
Charmberlain, B. (2017, November 3). Ben Chamberlain, #ASOS- Using deep learning to estimate CLTV in e-commerce #reworkretail. Retrieved from YouTube: https://www.youtube.com/watch?v=UIuiUec6rNI
de Jesus, A. (2018, August 7). Artificial Intelligence for Clothing and Apparel – Current Applications. Retrieved from techemergence: https://www.techemergence.com/artificial-intelligence-for-clothing-and-apparel/
Dennis, S. (2018, February 14). The Ticking Time Bomb Of E-commerce Returns. Retrieved from Forbes: https://www.forbes.com/sites/stevendennis/2018/02/14/the-ticking-time-bomb-of-e-commerce-returns/#3b33f7194c7f
Fedyk, A. (2016). How to tell if machine learning can solve your problem. Boston: Harvard Business Review.
Fridrich, M. (2017). Hyperparameter optimization of artificial neural network in customer churn prediction using genetic algorithm. Faculty of Business Administration, Brno University of Technology, 9-21.
Grossfeld, B. (2017, July 18). A simple way to understand machine learning vs deep learning. Retrieved from Zendesk: https://www.zendesk.com/blog/machine-learning-and-deep-learning/
Kozlowska, H. (2018, August 13). Shoppers are buying clothes just for the Instagram pic, and then returning them. Retrieved from Quartzy: https://qz.com/quartzy/1354651/shoppers-are-buying-clothes-just-for-the-instagram-pic-and-then-return-them/
Mitta, V., Sarkees, M., & Murshed, F. (2008, April). The right way to manage unprofitable customers. Retrieved from Harvard Business Review: https://hbr.org/2008/04/the-right-way-to-manage-unprofitable-customers
Quenqua, D. (2017, December 26). Many Unhappy Returns? Online Holiday Shopping’s Big Hangover. Retrieved from New York Times: https://www.nytimes.com/2017/12/26/style/online-holiday-shopping-returns.html
Ram, A. (2016, January 27). UK retailers count the cost of returns. Retrieved from Financial Times: https://www.ft.com/content/52d26de8-c0e6-11e5-846f-79b0e3d20eaf
Segura, A. (2018, April 30). Artificial Intelligence in Fashion Retail. Retrieved from The Fashion Retailer: https://fashionretail.blog/2018/04/30/artificial-intelligence-in-fashion/
Siff, L. (2012, May 21). Your Unprofitable Customers Are Killing You. Retrieved from Forbes: https://www.forbes.com/sites/lawrencesiff/2012/05/21/your-unprofitable-customers-are-killing-you/#6d3a447b388d
Skinner, R. (2018, February 8). ASOS Uses Machine Learning To Understand Customer Value. Retrieved from Forrester: https://go.forrester.com/blogs/machine-learning-asos-customer-value/
Softweb Solutions. (2018, January 8). How deep learning and AI radically change the way retailers interact with customers. Retrieved from Softweb Solutions: https://www.softwebsolutions.com/resources/deep-learning-and-AI-for-retailers.html
Steenburgh, T., & Avery, J. (2017). Marketing Analysis Toolkit: Customer Lifetime Value Analysis. Boston: Harvard Business School Publishing.
Wells, J. R., & Ellsworth, G. (2018). ASOS Plc. Boston: Harvard Business Review.
This definitely resonated with me as a long-time (and hopefully high-value!) ASOS shopper. It was interesting to learn how they combine churn modeling with LTV to come to customer lifetime value, and I’m curious to hear more about how their marketing and retention strategies deviate for different customer value segments, and what retention strategies are effective in reducing churn for each of those segments (e.g. coupons, search advertising, email campaigns etc.) and how they test the efficacy of these strategies. The idea of having a company fire their lowest value customers is interesting as well, particularly as customers can also move up and down the CLV chain, and because this type of decision can lead to negative press or brand risk.
This brings up some interesting debates about how a retailer should consider “customer loyalty” as opposed to customer value. For example, how should ASOS evaluate a customer that is a loyal buyer but also more likely to try and return items? They provide value in terms of consistent sales, but if half the items in each purchase get returned, the net value to ASOS could be quite mixed. Does this behavior call for a different application of machine learning? Or does showing a customer a webpage with only the items the customer is likely not to return lessen the impact or engagement that customer has with the brand experience?
As a long-time ASOS customer I appreciate this post! ASOS has been an industry leader in innovation around size and style predictions. This adds value to customers who are able to order their optimal size and get recommendations for products they may never have purchased. In addition, this also serves to reduce fulfillment costs for ASOS as customers are a) less likely to return an item if it fits and b) less likely to order multiple sizes if they are confident in the size prediction model. Given that items are frequently two day air shipped from the UK around the world, these cost savings could be significant for the company.
I enjoyed reading this article. I think that they way that ASOS has been integrating machine learning is a useful application, and seems that it would generally yield positive results. However, I do find merit in the comment made by @Greg Andrews above regarding possible unseen impact of “firing” customers that may in fact be loyal to the ASOS brand. A related concern that I have with this is the ability (or likely inability) for the machine learning system to be able to capture intangible benefits of customers that are in fact “negative value” from a dollar perspective, but may be net “positive value” if benefits such as positive word-of-mouth, brand championing, and referrals exist with that same customer. Do you think this is something ASOS should be worried about?
You’ve made an interesting point in the article about winning back a customer being harder than maintaining a current customer – which leads to identifying patterns when a valuable customer could drop off.
This also makes me wonder whether there is a way to make an unprofitable customer profitable by using ML/AI and predicting better sizing and styling for customers. It would eventually reduce the number of returns if the customer experience is improved via personalisation.
I agree that there is lots of room for AI in the customer retention space. The idea of having a central brain that analyzes customer inputs (potential to churn, predicted value, style, price point, geography, age, etc.) and then decides how to best retain that customer (reactivation code? highlight a new product? up communication frequency?), and through what channels at what time is very appealing. I agree the challenge here is primarily privacy. How do I map a customer across devices when cookies are being cleared, or are not allowed, or a person purchases a new device, etc.? Without that, how do I build an accurate model?
In addition, I wonder how the strategy employed by ASOS (using AI to improve ROI through customer retention) could be translated to smaller businesses. The ASOS approach only works if you have access to millions of customers and billions of site visits/interactions. I am not proposing that ASOS needs to figure out this solution for smaller businesses, but I am interested to see how this technology becomes more accessible over time as predictive models improve.