DMs for Dollars
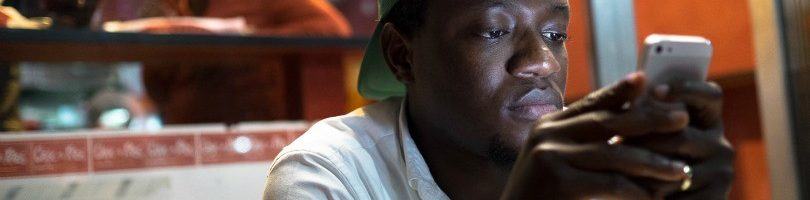
Individuals and businesses in America enjoy access to a mind-boggling array of financial services. Borrowing is ubiquitous – consumers swipe plastic to their heart’s content, smaller firms tap into bank loans, and big corporations issue public bonds. And while societal over-indebtedness can lead to systemic financial calamity, access to banking and credit are core drivers of the virtuous cycle of investment, production, and consumption. Most people in the developing world, however, lack access to the most basic financial services. The World Bank estimates that nearly 60% of adults in Sub Saharan Africa, and nearly 45% in all developing countries, are either unbanked or severely underbanked.1
Lenders are fearful of extending credit given a lack of data and tools to assess the creditworthiness of potential borrowers. The holy grails of borrower risk assessment – evaluating ability and willingness to repay – are challenging in data-scarce environments. Consumers’ financial flows are unpredictable as most lack the luxury of regular paychecks, living payout to payout, which might come from selling farm produce one month and temporary manual labor the next. Workers also struggle with sporadic expenses, including medical emergencies and requests from family who need help.2 Moreover, they have non-existent or fragmented financial records because they earn wages in cash and have little or no formal savings or registered assets.3
Tala, a California-based fintech company, is working to transform financial access by building a lending business grounded in machine learning-driven credit assessment. The company’s Android-based mobile application uses machine learning on a user’s smartphone data to predict her capacity to borrow and her associated repayment/default patterns. The app mines the rich reams of consumer mobile data to build an exhaustive picture of a consumer’s financial life, using “alternative data-points” as reliable proxies for user financial attributes.4
Tala uses a combination of Android and behavioral data to instantly underwrite first-time customers. Behavioral data refers to how a customer interacts with or moves through the app (e.g., which pages she visits and how she arrives there) or whether she read the terms and conditions. Device data examples include device type and ID, operating system vintage, and the apps on the phone.5 The Tala app requests access to certain information, including SMS, call records, and location patterns, collecting over 10,000 unique data points per user.6 These data points can provide valuable insights on a customer – prepaid-minute purchase patterns can indicate a steady or uneven cash flow, and the timing and frequency of calls and text messages can indicate whether she is working a steady job (for example, fewer calls between 9 a.m. and 5 p.m. may indicate that someone is working during those hours).7
Tala has already delivered credit to nearly 1.3 million customers and originated more than $300 million in loans in countries like Kenya, India, and Mexico.8 In the future, the company plans to continue building deeper financial analysis capabilities (e.g., via partnerships with PayPal) and to develop more rigorously data-science focused machine learning techniques trained on historic user data (e.g., recently hired Uber’s Head of Data Science as Chief Data Officer). For repeat customers of Tala, for example, existing repayment behavior will be the most important factor for future lending decisions, leading to a virtuous cycle of more data and more learning that can drive lending of greater amounts and expansion into new types of financial services.9
As the company looks ahead, I would advise them to leverage their data and algorithms to play a more prominent role in the financial well-being of their customers. Tala could build out an offering of non-credit products (e.g., insurance, savings) and enable customers to make good financial decisions (e.g., through the use of targeted alerts).10 The company could spread the mobile lending revolution globally – Africa remains the world’s leader with more than 10% of adults using mobile money accounts.11 Tala could further work with more traditional credit agencies (e.g., Transunion) to complement their existing services to banks and other financial institutions. Tala’s machine learning capabilities could lead to more intelligent credit decisions and lower the cost of sourcing, evaluating, and disbursing loans across a variety of settings.12
Finally, I would challenge Tala to proactively develop a robust risk-mitigation framework for safe-guarding its goldmine of sensitive data. The use of machine learning to analyze alternative data raises serious privacy, ethical and legal concerns. Many people might not feel comfortable with a company having access to such intimate details about their lives. Even if Tala itself behaves ethically, the more data it holds the more that can be stolen by malicious hackers in a data breach. How can Tala work with the rest of the fintech industry to develop and implement data storage and use standards?
[Word Count: 785 Words]
References
- Jake Kendall, “Fintech Companies Could Give Billions of People More Banking Options,” Harvard Business Review, January 20, 2017. https://hbr.org/2017/01/fintech-companies-could-give-billions-of-people-more-banking-options , accessed November 2018
- “Tala: Using Machine Learning to Provide Access to Credit for the World’s Unbanked,” HBS Digital Initiative, April 9, 2018. https://d3.harvard.edu/platform-digit/submission/tala-using-machine-learning-to-provide-access-to-credit-for-the-worlds-unbanked/
- Tobias Baer, Tony Goland, and Robert Schiff, “New Credit-Risk Models for the Unbanked,” McKinsey & Company, April 2013. https://www.mckinsey.com/business-functions/risk/our-insights/new-credit-risk-models-for-the-unbanked , accessed November 2018
- Ibid
- Tala, “Data Ethics at Tala”, https://tala.co/dataethics/ accessed November 2018
- Jake Kendall, “Fintech…”
- Tobias Baer, “New Credit-Risk…”
- “Tala Raises $65M in New Financing”, FinSMEs, April 19, 2018 http://www.finsmes.com/2018/04/tala-raises-65m-in-new-financing.html
- Tala, “With an Eye Toward Global Expansion, Tala adds Kevin Novak as Chief Data Officer”, https://tala.co/press-releases-1/, accessed November 2018
- Tobias Baer, “New Credit-Risk…”
- Toby Shapshak, “Mobile Drives Financial Inclusion in Africa, Growing 20% in Past Six Years”, Forbes, May 16, 2018. https://www.forbes.com/sites/tobyshapshak/2018/05/16/mobile-drives-financial-inclusion-in-africa-growing-20-in-past-six-years/ accessed November 2018
- Jon Walker, “Artificial Intelligence Applications for Lending and Loan Management”, Tech Emergence, March 27, 2018. https://www.techemergence.com/artificial-intelligence-applications-lending-loan-management/ , accessed November 2018
Great points about the tradeoff between accuracy and security. I would argue the contention lies not in working with other fintech companies but perhaps leveraging best practices in endpoint security from enterprise IT software firms?
Really interesting to see how seemingly disparate data can come together to build a picture of a person’s creditworthiness. On the notion of data security, I agree it’s imperative to take precautions. That said, perhaps a first step might be to reduce the data collected from the get-go. With 10,000 data points per user, would it not take a tremendous historical record of financial performance for the algorithms to then implicitly attribute the probability of repayment to various factors? Is there such thing as too much data?
This is an important topic for a rapidly changing market. I would be interested to know whether Tala is already incorporating Kenyan customers’ M Pesa mobile money account transactions into its machine learning algorithms. Secondly, as an online lender, how does Tala deal with fraud detection? Does it verify information from borrowers manually or depend solely on smartphone data and assume the truthfulness of other information provided by consumers? It seems like their technology platform could be applied also in developed markets, or sold as a SAAS product to other lenders looking to incorporate additional datapoints into their credit underwriting process.
I really enjoyed this piece– you describe an innovative model of affording credit in economies where constraints are broad and deep. I appreciate the lens you took on big data and machine learning, and believe you very eloquently delineated some of the major risks associated with creating this platform (e.g. hackers) as well as possible opportunities for growth (e.g. insurance, savings). This is a fascinating area of growth, it seems, and I wonder what the competitors are doing to mitigate these risks, or embrace these opportunities you site. In the same way that pharmaceutical and medical device companies come under scrutiny when running clinical trials in low-income countries due to the searing history of predatory behavior, I would also question whether this product is too invasive, and whether the regulatory frameworks are in place to prevent predatory behavior and exploitation. Thanks for a great read!
This is a great piece – it also points to what is being done globally to address the level of unbankedness in a lot of developing countries. Like Emma, I wonder about the exit opportunities for this technology. Does it eventually get used by banks in these countries? Does the future of banking reject physical locations for branches? Is this extendable beyond micro-loans? I agree with M.E in worrying about the regulatory and privacy implications of such tech. There are a number of companies using these sort of technologies to create alternate credit scores to guide investment decisions. And undoubtedly, those scores lead to better decision-making by lenders. But whether those technologies will actually lead to lower interest rates or increased access to capital in those markets or a reduced overall cost to lending I believe, remains to be seen.
Very information article. You mention that Tala has already delivered credit to nearly 1.3 million customers and originated more than $300 million in loans in countries like Kenya, India, and Mexico. I would be curious to know how these loans have performed. This would be a fantastic indicator of the robustness and evaluative power of the algorithms. If successful, Tala would be able to deliver much more than loans – the social value of readily available credit for deserving applicants can have the power to change economies and personal journeys.
Thank you for all the work you put into this soghtful article. It is obvious you have a passion for this industry. As I was reading your article, it made me wonder whether or not customers can assume Tala would act “ethically” as you hypothesized. In today’s world of big data, selling macro data is a huge concern amongst society in addition to the added security risk of someone stealing this type of information. Especially in the world of personal finance outside of conventional banking, I wonder how Tala and other fintech companies can build trust when consumers have seen egregiously mismanaged data collection by other big tech firms?
Cool article. Thanks for your insights! I’d be intrigued to learn more about the accuracy of Tala’s algorithm, and how they made the choice to use certain data as predictive of creditworthiness. In particular, data such as “whether a user reads the terms & conditions” seems to me like it would be unrelated to and un-predictive of someone’s ability to repay. So I’d want to learn more on how they chose to use that metric, and what the outcome has been.