CircleUp – Identifying the next “Big Thing” using Machine Learning
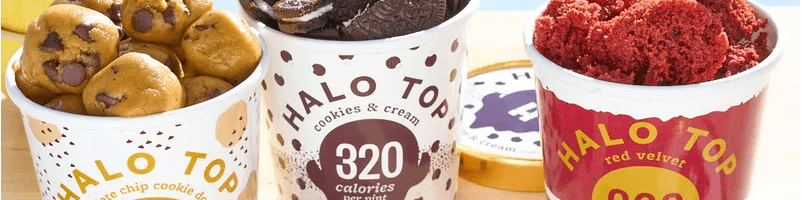
CircleUp is disrupting the consumer VC space using its machine learning platform, Helio, which uses public, partnership and practitioner data to identify small consumer brands with breakout potential.
What if you could use machine learning to predict the next “big thing”?
Trendsetters could use this technology to stay ahead of the pack. Job candidates could use it to identify attractive employers. And investors could use it to make a lot of money.
CircleUp has developed this technology to identify consumer brands with “breakout” potential. Launched in 2012 as an intermediary platform for entrepreneurs to raise “crowd-funded” capital, CircleUp initially used big data to help investors connect to potential investments.[1] Overtime, CircleUp developed a proprietary machine learning platform called “Helio” which powers its Credit fund and its latest initiative launched in 2017: the CircleUp Growth Fund, a $125 million investment fund.[2] CircleUp Growth Fund focuses on early stage consumer companies. Its investments include Halo Top ice cream and HUM beauty.
Source: https://www.foodandwine.com/desserts/halo-top-most-popular-ice-cream-pint-in-us
Machine learning is important to CircleUp’s process improvement for two reasons: (1) it provided the Company with the confidence to evolve from an investment intermediary to a credit fund and equity investment firm and (2) it vastly improves upon existing investing methodologies.
Increasingly, there are a growing number of small consumer brands because of low barriers to entry and changing consumer preferences and a growing number of data points about consumer behavior as a result of improving tracking technologies.[3] In my previous role as an investor in consumer businesses, I spent many hours sifting through databases to identify interesting companies and researching countless data points to assess each company, using everything from financials to social media sentiment and customer reviews.
Source: https://www.flickr.com/photos/wocintechchat/25392378763/
Machine learning provides CircleUp with two primary competitive advantages: (1) the capacity to monitor and analyze millions of companies, which would otherwise be incredibly resource-intensive and (2) the ability to use many disparate consumer data points to identify companies with “breakout” potential while minimizing human bias. Once the investment is made, the algorithms may also help identify potential operational improvements to improve company performance.
The key issue with Helio’s machine learning is the breadth, depth and quality of the data that informs its algorithms. CircleUp tracks 1.4 million brands and pulls from countless data points that fall into three buckets: public, partnership and practitioner data[4]. Per the Company’s website, the “outcome is a knowledge graph of the entire consumer space that maps reviews, labels, social posts, pricing, location, and more to individual products and brands”[4].
In the short term, CircleUp is focused on continuous improvement of its data, given numerous errors associated with the sheer scale and diversity of information being processed, which results in faulty and inconsistent data. CircleUp addresses these errors using high level rules and processes. For example, CircleUp applies natural language processing to filter out companies that are not relevant or do not focus on the US or Canada, where they are currently focused[4].
In the medium term, the Company is focused on improvement of its algorithms and expansion of its data. CircleUp has begun to grow its partnership data sources. For example, it struck a strategic partnership with Nielsen in December 2017, providing Helio with access to Nielsen’s rich retail sales data[5]. The Company is also growing its practitioner data, which includes information from the companies that apply to CircleUp’s crowdfunding function and its conversations with entrepreneurs.
I’d recommend management focus on improving its machine learning algorithm by growing its data sources to stay ahead of its competition in the short term. In particular, I’d focus on partnership and practitioner data which are proprietary and provide a more sustainable competitive advantage.
In the medium term, I’d recommend that the Company test and refine its algorithm as its investments prove out over time. The Company has a critical first mover advantage and should remain focused on using its learnings from existing investments. For example, they could refine the attractiveness of different categories of investments or perhaps build in data points around qualitative features, like characteristics of its management teams or organizational design. Interestingly, the data suggests this data-driven method of investing dramatically improves the diversity of companies getting funded. According to Fast Company, 35% of the companies on the CircleUp platform are women-led, or roughly 17 times more than the industry average[6]. The Company should preserve this unique ability to identify attractive investments based on data, while also exploring its ability to identify potential breakout brands amongst traditionally underfunded companies.
CircleUp’s business model and the disruption to existing private investors raises numerous questions. How sustainable is this competitive advantage? What might this machine learning platform get wrong or where might biases be introduced into the system? Can this machine-based learning be applied to investing in other industries, where customer data may be less prevalent or easy to come by?
(778 words)
[1] “Backed With $1.5M, Circleup Aims To Be The Angellist For Consumer And Retail Startups”. 2018. Techcrunch. https://techcrunch.com/2012/04/18/circleup/
[2] “Circleup Announced $125 Million Venture Fund”. 2018. Techcrunch. https://techcrunch.com/2017/10/31/circleup-announced-125-million-venture-fund/.
[3] Consumer Brands Seeking Innovation Reach Out to Emerging Companies. Garland, Russ. The Private Equity Analyst; New York (Dec 2014).
[4] “Circleup”. 2018. Circleup. https://circleup.com/helio/.
[5] “Circleup And Nielsen Collaboration Fuels Growth Of Early-Stage Consumer Products”. 2018. Prnewswire.Com. https://www.prnewswire.com/news-releases/circleup-and-nielsen-collaboration-fuels-growth-of-early-stage-consumer-products-300570909.html.
[6] “This Investment Platform Funds More Diverse Companies By Focusing On Data, Not Founders”. 2018. Fast Company. https://www.fastcompany.com/40527386/this-investment-platform-funds-more-diverse-companies-by-focusing-on-data-not-founders.
Cool article, and very inspiring to see that machine learning applied in this context hews towards improving diversity of target companies, whereas in other machine learning contexts (Amazon’s recruiting software) the opposite is true at the individual level. The biggest question for me is why CircleUp is seeking to disrupt VC, and not PE? It seems that if the underlying thesis is that algorithms trained on quality data-sets could be more effective at identifying investment opportunities than humans, then it would be easier to prove out in a landscape where data is more prevalent. My thought would be that established companies (classic PE targets) would have a significantly larger amount of data that Helio could crunch on vs. early stage companies (classic VC targets).
Came for the picture of ice cream, stayed for the great article.
In your article you recommend CircleUp stays ahead of its competitors with a first mover advantage. This seems to hint at that speed and more data is the advantage. Would you agree that should CircleUp focus on building robust algorithms, watch guarding for biases in the data, and observing the current investments’ success to update the pool of data influencing their company selection algorithm?
In your post you suggest that “they could refine the attractiveness of different categories of investments or perhaps build in data points around qualitative features, like characteristics of its management teams or organizational design.” I’m skeptical that these data points or metrics can be effectively incorporated into an investing algorithm. The premise of Helio seems to be that early sales data, social media mentions etc. are leading indicators of success for small consumer brands and can be tracked to move quickly on up-and-coming brands. Those metrics seem to have a pretty direct link to potential for success across all companies. With qualitative features, however, there are numerous models for success. As we’ve learned in LEAD, no single management style or organization design is a leading indicator of future growth or success.
Interesting post. How does the Helio technology account for “breakout” brands for concentrated market segments? For example, how would one discover and capitalize on a brand that serves a niche consumer segment, such as an effective conditioner for curly hair? I imagine the technology would focus on mass trends.
Thank you Chris Li for this great piece of knowledge. I was not aware of the work CircleUp was doing before reading your post. As I read through your post, what struck me as the most interesting is the ability the company has had to improve the quality of the data it feeds into the algorithm, e.g. partnering with Nielsen. At the same time, I find it hard to wrap my head around the fact that investment decisions are being made based purely on data, especially when we are talking about early stage companies that are still developing i) their business model, and ii) their leadership team. This leads me to question how will CircleUp stand the proof of time (or proof of return vs. other benchmark) when so much of the factors that influence the success of an early stage company cannot be measured objectively?
I am very interested with the question about sustainability. Although their information is proprietary, I wonder how easily replicable their AI/ML platform would be? If competitors are able to come close to processing and determining the same outcomes with their own platform, then I see their advantages to significantly decrease. I imagine that their platform is difficult to replicate, and will grow in complexity as their data sets become richer and their AI becomes more advance. But as companies rely heavily on AI/ML, they should be aware of their competitive advantages and seek opportunities to minimize competition in the space.
Really interesting article! I agree with your recommendation for CircleUp to invest in proprietary data partnerships to build a sustainable competitive advantage. That said, I am curious about accuracy of the outputs from the machine learning system and wonder whether identifying good investments is something that can truly be automated. Anecdotally, I have heard that the founding team is at least as important to investors as the product. On that vein, I wonder whether this system can assess founder potential well enough to be effective as as sourcing tool. Perhaps this is something the company can build into their data set, maybe by partnering with LinkedIn to assess the founders’ skill sets and credibility as part of output.
I think that what CircleUp is doing is super cool, especially for the point you raised around female investors and the potential elimination of biases pervasive throughout the fundraising process. My only concern is about the dependencies upon partners and data sources in general – if you don’t own and generate all of your own data inputs, how can you be confident that they’re not going to dry up? What happens if Nielsen decides they want a piece of the pie and pulls the plug on the partnership? What if some of your data providers themselves lose funding (as happened to me when one of my main sources of K12 school enrollment data used lost funding from the federal government last year)? Data risks certainly aren’t a reason to shy away from making data-driven decisions, but if I were investing in a fund that was making investment decisions largely on the basis of Helio recommendations, I would want to know that they have a plan to keep deploying capital even if Helio breaks down.