Can the maker of Post-it notes solve healthcare pricing?
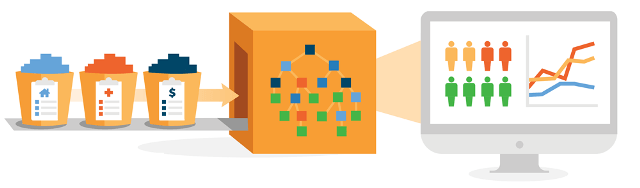
3M and Google’s Verily team up to beat healthcare costs with machine learning
3M in the healthcare space
Of the many divisions in 3M, a multinational conglomerate known the world over for their iconic Post-it sticky notes, there is one in particular on a mission to improve healthcare. For years, 3M’s healthcare division has produced the software that helps hospitals across America “code” their patients’ conditions into an appropriate diagnosis-related grouping (or “DRG”), that tells the hospital what a likely treatment plan and costs to expect, and tells insurers what a reasonable payment will be for treatment.
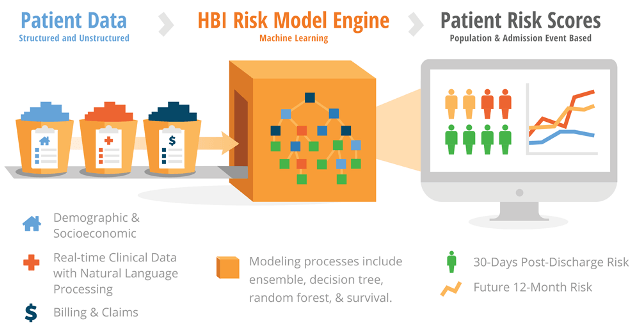
This system of identifying the “product” code that an incoming patient will receive covers every treatment, from hip replacements to “bitten by pig, initial encounter”. Critics have suggested that this system of paying providers based on the procedures they carry out incentivizes providers to exaggerate diagnoses, and perform unnecessary and costly procedures while focusing too little on patient outcomes.
Learning from the data
In the face of this old system, 3M announced in 2016 a partnership with Verily (the healthcare arm of Alphabet), to build the 3M Performance Matrix [1] – a platform that would allow hospitals to synthesize their data from diverse sources such as insurance claims, patient electronic health records, hospital billing platforms, and lab and pharmacy data to compare their operations against larger industry averages, and identify at-risk and underperforming areas of their care delivery cycle.
Instead of merely processing claims, 3M’s new platform would iteratively learn from patients who received similar diagnoses but received different care plans, and begin to suggest to hospitals which care plans were more effective at responding to and preventing complications.
In both the short and longer term, 3M plans to leverage a few growing trends in the healthcare industry to transition from merely recording claims, to helping hospitals reduce and optimize them:
- Population health management algorithms synthesize and combine disparate data sources (EHRs, billing platforms, medical claims, labs and pharmacies), to provide a unified and more accurate picture of a patient’s total lifetime of care and results. 3M uses this unified view to better identify avoidable complications, and correlation between interventions earlier in life and reduced disease later.
- Value-based (also called “accountable care”) reimbursement modules enable payers (insurance companies) to track and pay providers (hospitals) for outcomes instead of procedures by setting and measuring how much acute care was not needed. The performance matrix learns and identifies preventive services that shift hospitals’ focus from costly reactive procedures in order to reduce future needs for hospital visits or chronic care management [2].
- Big data sources are getting better and easier to manage. In addition to internal data (claims data, lab data), publicly derived data (e.g. census data), and device data (e.g. smart watches, smart scales) are now allowing insight into populations at a much more granular level [3].
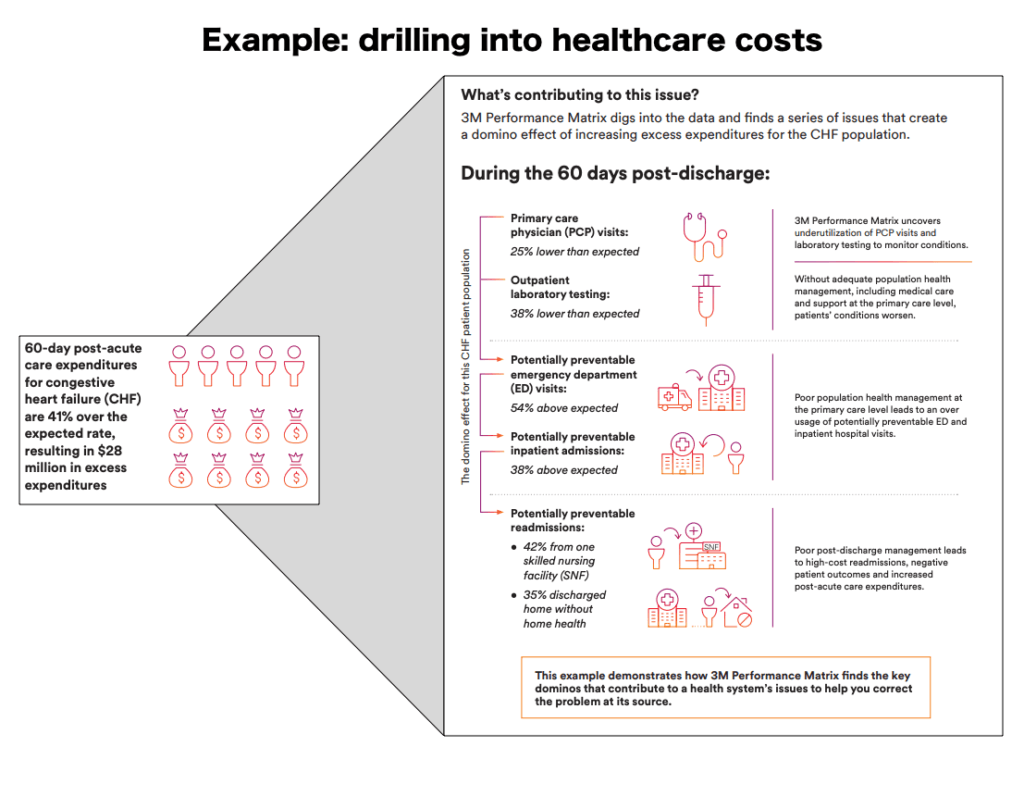
3M brought the data, and Verily brought the machine learning expertise – within a year, the company debuted the Performance Matrix at the Healthcare Information and Management Systems Society conference that was capable of parsing diverse data sources in a hospital to identify unusually long stays, patients whose treatment deviated from expected, and conditions with atypically high readmission rates so that administrators could focus on root causes [4].
What’s missing?
3M and its contemporaries might well be served to defend their turf: competition has arrived in the Population Health management space. IBM and Siemens have partnered to build software for hospitals in the space [5], and recently Amazon announced a partnership with JPMorgan and Berkshire Hathaway to tackle the same problems, starting with their own employee base [6].
In the short term, this competition should encourage 3M to double down on their competitive advantage – enormous historical claims processing databases – and go “deep” in developing machine learning techniques to learn from those data. In the longer term, the Performance Matrix would benefit from diversifying the data sources it can integrate into its analysis – go “wide” as competitors begin to mature, to provide the most holistic view.
Outstanding questions as 3M looks to the future of healthcare
- In the immediate term, a shift from fee-for-service to fee-for-value pricing will cut into short-term profits. How can payers help providers embrace the new paradigm in the face of tightening budgets?
- In the longer term, how will 3M differentiate its product as major technology players enter the space and tap into the same data sources they do?
(Word Count: 757)
Sources
[1] Neil Versel, “3M, Google’s Verily team up on population health management technology”, Oct 12 2016. https://medcitynews.com/2016/10/3m-verily-population-health/, accessed Nov 2018
[2] NEJM Catalyst, “What Is Value-Based Healthcare?”, January 1 2017. https://catalyst.nejm.org/what-is-value-based-healthcare/, accessed Nov 2018.
[3] Dan Addyson, “Population Health Methods: How patient risk scoring works”, March 7 2018. https://towardsdatascience.com/population-health-methods-how-patient-risk-scoring-works-70061ba327d2, accessed Nov 2018.
[4] Susan Morse, “3M, Verily, to unveil performance matrix at HIMSS18”, Feb 23 2018. https://www.healthcareitnews.com/news/3m-verily-unveil-performance-matrix-himss18, accessed Nov 2018.
[5] Neil Versel, “IBM Watson gets ’60 Minutes’ love, then teams with Siemens”, Oct 11 2016. https://medcitynews.com/2016/10/ibm-watson-60-minutes-siemens/, accessed Nov 2018.
[6] Angelica LaVito, Amazon, Berkshire Hathaway, and JPMorgan Chase to partner on US employee health care, Jan 30 2018. https://www.cnbc.com/2018/01/30/amazon-berkshire-hathaway-and-jpmorgan-chase-to-partner-on-us-employee-health-care.html, accessed Nov 2018.
This is a really interesting topic that everyone in the industry – from providers to payers to policymakers – is trying to address. There’s so much data in the healthcare system that it only makes sense to run some analysis on it and gain insights from aggregated data that could help to inform an individual patient’s treatment plan. Given the current organization of the industry, I see several challenges that may arise when trying to analyze patient data from within a hospital or provider system. For example, how do we ensure that data is actually being shared across systems in a way that gives the system a full picture of the patient’s health? Right now the data is incredibly siloed, and patients seem to be the sole owners of their full health history. Furthermore, how might regulations (HIPAA, 42 CFR around mental health data) influence access to data and what can be done with the data? As we look toward the future, I’m also curious how data generated outside of the healthcare system (from social media or wearables) could improve the value of machine learning in healthcare.
I really enjoyed this read and learning about 3M’s initiative to tackle over-spending within the US healthcare system. This initiative, if broadly adopted, could play a transformative role in rationalizing the provision of healthcare services, which has seemed an intractable problem so far. I would be curious to see how providers respond to this solution. How much weightage should a provider give to suggestions made by the 3M system? How would this system adjust for complications or patient-specific idiosyncrasies that, if overlooked, could harm patient outcomes? Providers will want to retain autonomy over clinical decisions, and knowing this, how can providers be held to task for prescribing procedures that seem unnecessary from the 3M model’s perspective? In the absence of any enforcement mechanism, the new system will likely not have the desired impact on provider decision-making. Finally, how do we think of liability-sharing for patient outcomes, in a world where providers receive nudges from machine-learning models and may have to be incentivized to follow such nudges?