Can Machine Learning Save Us From Being Stuck in Traffic?

Rapid-Flow is solving traffic congestion by offering governments a real-time traffic signal control system powered by machine learning.
Traffic congestion is one of the most critical problems facing modern cities. The Economist estimates that the costs related to traffic congestion in 2017 in the US, Germany and the UK were $461bn [1]. What can governments do to solve this pressing issue?
Pittsburgh based Rapid-Flow Technologies, a spin-off from Carnegie Mellon University’s Robotics Institute, is utilizing machine learning to reduce traffic congestion. The company’s first product, Surtrac, offers a real-time traffic signal control system. Utilizing cameras placed at road intersections and machine learning, Surtrac optimizes the performance of traffic signals for the traffic that is actually on the road, leading to “less waiting, reduced congestion, shorter trips, less pollution, and happier drivers” [2]. Exhibit 1 showcases the details of how Rapid-Flow’s technology works.
Results from Surtrac’s first implementation are promising. From 2012 to 2016, Surtrac was installed at 50 intersections across the city of Pittsburgh (See Exhibit 2 for a map of deployment). Substantial traffic improvements followed this pilot: the system reduced travel times by 26%, the number of stops by 31%, wait times at intersections by 41%, carbon emissions by 21%, and total and fatal crashes by 13%-36%.
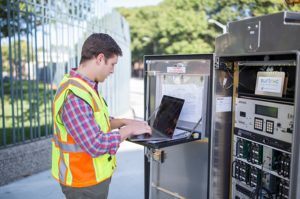
Traffic Signal Management: A Perfect Fit for Machine Learning
Applying Harvard researcher Anastassia Fedyk’s framework [3], traffic signal management is a fantastic area for machine learning to provide value. Firstly, the task involves managing many interconnected variables changing so rapidly over the course of a day that “automated without learning” solutions, which are the systems that most traffic signal networks use today, end up being inefficient. Secondly, the problem involves predicting traffic patterns rather than inferring what causes traffic, and machine learning models perform well in these contexts. Thirdly, the amount of traffic data available is vast, quite complete and can be easily collected by hardware installation. Lastly, new data becomes available constantly, allowing the model to improve with time.
In this context, it’s not surprising that Rapid-Flow is not alone in the space: competitors include Vivacity, a UK based company installing similar sensors and sharing its machine learning generated recommendations with drivers [4]. Additionally, governments are developing their own “AI enabled” smart lights solutions, such as Maryland’s Governor recently announced [5]. Moreover, researchers are working on new machine learning models to manage traffic [6]. In the future, big tech companies such as Google, Uber or Apple, which are developing self driving car technologies and own vast information involving maps and location, could decide to enter the market.
What’s Next for Rapid-Flow?
According to McKinsey, in machine learning “more data translates into more insights and higher accuracy because it exposes algorithms to more examples they can use to identify correct and reject incorrect answers” [7]. This explains why Rapid-Flow is looking to expand fast: the company has recently deployed its technology across the cities of Kane, IL, Portland, ME, Quincy, MA, and Atlanta, GA. Collecting new data from different cities, regions and neighborhoods will allow its machine learning technology to perform better in diverse environments.
Moreover, learning from the pilot, the team has enabled Surtrac to consider all travelers, including pedestrians and cyclists, as it manages a traffic signal system. This is a key improvement, as the initial version of the software was focused on improving car traffic, and was thus biased against pedestrians and cyclists.
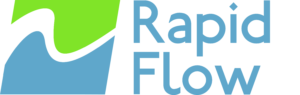
Thinking longer term, some of the key challenges that Rapid-Flow faces are error tolerance and scaling. Regarding error tolerance, Anastassia Fedyk warns us that when using machine learning “mistakes will happen, and they will happen most often in ways that you cannot anticipate”. Tesla and Uber both were involved in self driving car fatal accidents recently [8], and an accident where AI enabled traffic signals cost a life could be brutal for the future of Rapid-Flow. Therefore the company needs to be extremely careful with the safety checks it develops: security should never be compromised in the quest for better performance.
Secondly, in order to scale faster, Rapid-Flow should shift its focus mainly to developing its software service, and try to build hardware partnerships. Currently, the company installs its cameras, sensors and controllers in every city it operates in. By partnering with companies that have resources and expertise in deploying large scale hardware systems, such as telcos or utility companies, Rapid-Flow could grow its footprint much faster, and focus entirely on improving its algorithms with the new data and variables collected.
Finally, there are some important questions about Rapid-Flow’s future that remain uncertain. Firstly: should the company also be interacting directly with citizens by accessing their location and providing tailored route recommendations? Or should it continue to work exclusively with governments? Secondly: as the company grows, it will collect data of millions of citizens around the world. How can the company make sure citizen’s data is securely stored? What would the consequences of a hack or data leak be to the company’s future?
(Word Count 798)
Exibit 1: How Does Surtrac Work?
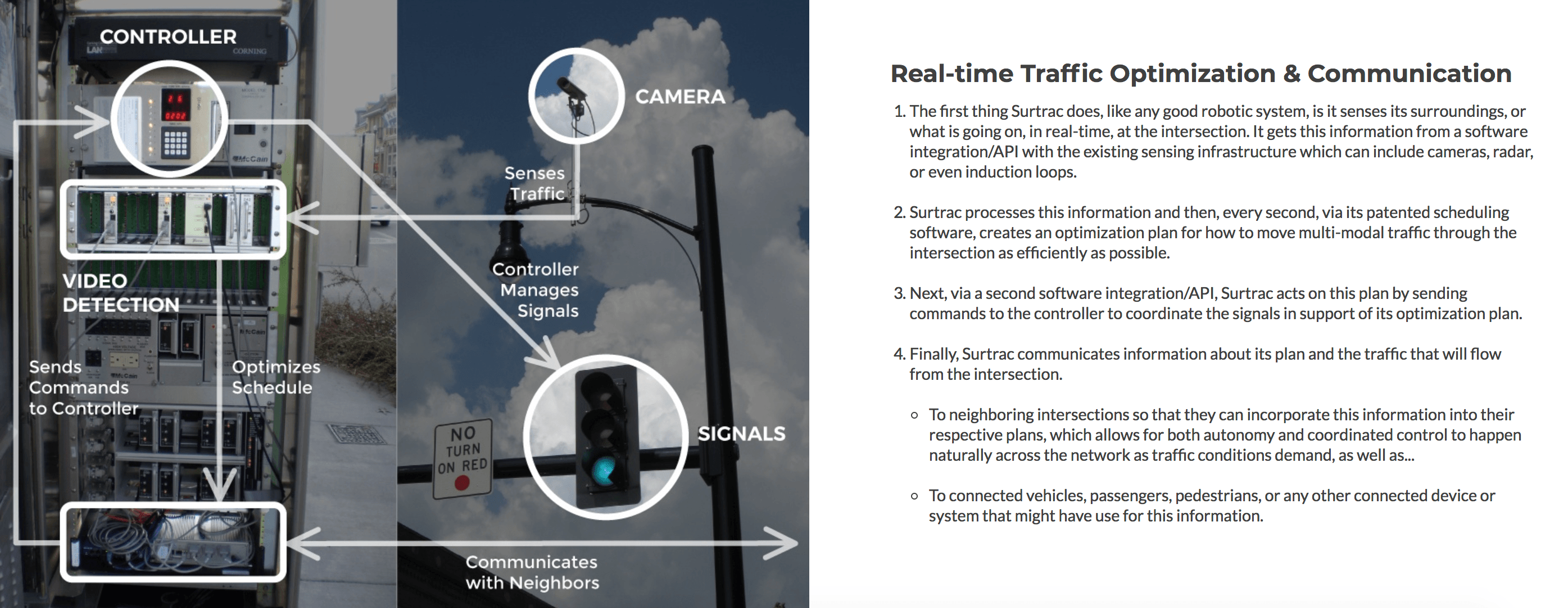
Exhibit 2: Surtrac’s Pittsburgh, PA Deployment Mapped

Exhibit 3: 2017 Estimated Economic Cost of Traffic Congestion
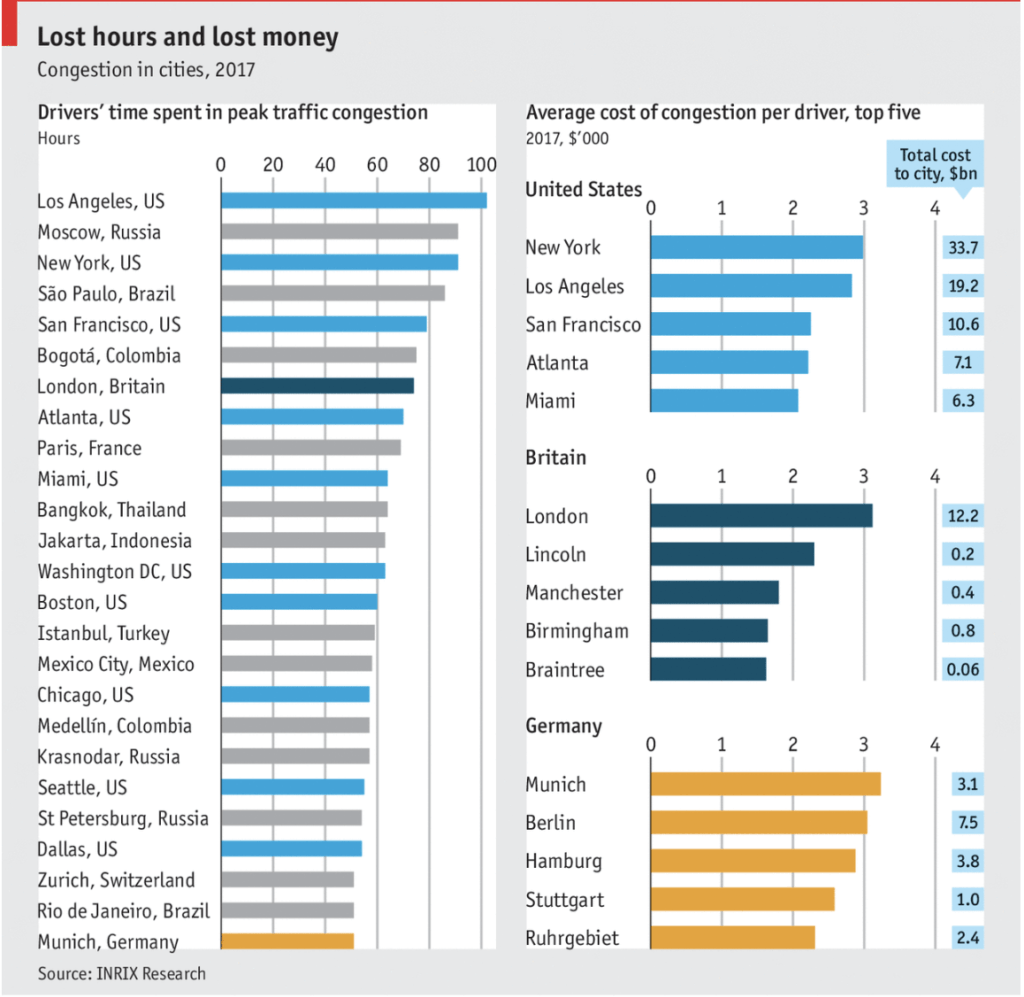
Endnotes
[1] – “The hidden cost of congestion”, The Economist, Febraury 28th 2018, https://www.economist.com/graphic-detail/2018/02/28/the-hidden-cost-of-congestion
[2] – “Surtrac Deployment at Urban Grid Networks in Pittsburgh Neighborhoods”, Rapid Flow Company Website, August 30th 2018, https://www.rapidflowtech.com/blog/surtrac-deployment-at-urban-grid-networks-in-pittsburgh-neighborhoods
[3] – Fedyk, Anastassia, “How to tell if machine learning can solve your business problem”, Harvard Business Review Digital Articles, November 15th 2016.
[4] – Williams, Henry, “Artificial Intelligence May Make Traffic Congestion a Thing of the Past”, The Wall Street Journal, June 16th 2018, https://www.wsj.com/articles/artificial-intelligence-may-make-traffic-congestion-a-thing-of-the-past-1530043151
[5] – Shaver, Katherine, “‘Smart’ traffic signals soon will change themselves in Maryland”, Washington Post, October 25th 2017, https://www.washingtonpost.com/local/trafficandcommuting/smart-traffic-signals-soon-will-change-themselves-in-maryland/2017/10/25/d4f57058-b9bf-11e7-9e58-e6288544af98_story.html?utm_term=.c805dd4fa25c
[6] Liu, Ying & Liu, Lei & Chen, Wei-Peng. “Intelligent traffic light control using distributed multi-agent Q learning”, 2017 IEEE 20th International Conference on Intelligent Transportation Systems (ITSC), 2017.
[7] – Bughin, Jacques et al., “Artificial intelligence: The next digital frontier”, McKinsey Global Institute, June 2017, https://www.mckinsey.com/~/media/mckinsey/industries/advanced%20electronics/our%20insights/how%20artificial%20intelligence%20can%20deliver%20real%20value%20to%20companies/mgi-artificial-intelligence-discussion-paper.ashx
[8] – Wakabayashi, Daisuke, “Self-Driving Uber Car Kills Pedestrian in Arizona, Where Robots Roam”, The New York Times, March 19th 2018, https://www.nytimes.com/2018/03/19/technology/uber-driverless-fatality.html
Traffic Jam Picture: By NOMAD – https://www.flickr.com/photos/lingaraj/2415084235/sizes/l/, CC BY 2.0, https://commons.wikimedia.org/w/index.php?curid=4783150
This seems like a perfect application for machine learning – I think your point about the completeness of the data is really important. Data cleanliness seems to be a critical issue for a lot of applications that use data about public infrastructure or other projects. And I hope it means we all spend less time in traffic in the future!
Thanks Amina! I wanted to do a public sector example and there isn’t much published out there yet, this was one of the only neat examples I could find.
Super interesting application of Machine Learning. Exhibit 3 with estimated costs due to congestion is a great way to look at the magnitude of this problem. I agree with Amina – data sanctity is really important here. Regarding the option of either working with the government or individually with consumers – I believe working with government would help them scale faster and get access to data quickly. Working with consumers could also mean a slower pace of growth like any other digital company and also tough competition from similar apps such as Google/Uber like you mentioned who can decide to enter the market.
I find this topic very intriguing! I have personally sat through too many hours of traffic and agree with you that an algorithm-driven solution to this problem will contribute so much more than just reducing hours spent on the road (a friend of mine came up with his remote doctor start-up idea as doctors in large cities nowadays waste too much time while stuck in the traffic).
To address your question on software vs. hardware, I definitely think that focusing more on the software will allow them to develop a more advanced solution that takes into account and learn from multiple variables. I think there is even a potential for the data collected through Rapid-Flow to help plan road constructions to minimize traffic jam in developing countries as well.
One thing I am not 100% clear on is that if Rapid-Flow decides to interact directly with the customers, would they serve the same purpose as Google Maps and Waze? I can see a scenario where the company becomes a price taker if they work exclusively with governments, but am unsure if they can remain competitive as they directly approach end-customers.
Great Article. This post is definitely something I can relate to. Citizens of Mexico City can spend on average more than 3 hour commuting to work. This is a great application of Machine Learning to improve the quality of life of millions around the world. I get the impression that this solution could become mainstream in the short term while we wait for autonomous vehicles. However, as you mention, companies implementing this type of technologies need to be careful on how they scale. One accident could putt the technology at the scrutiny of the public, causing mayor drawbacks to scale.
Thanks Sebastian, this was a really interesting read. One question that comes to my mind is the long term impact that can be created using this data such as construction of new flyovers, number of lanes needed etc. Hoping a brighter future for this amazing application of machine learning.
Interesting application of machine learning and hopefully with autonomous cars being developed in the same time, one day we can get rid of all traffic jams! You raise a good point about data privacy and storage – in my mind a very important question is who owns the data? Is it the government or the company?
A very interesting article that I read about Waze a while ago also pointed out the issues of data ownership – should we allow private companies to be involved in designing traffic flow in cities? Waze already diverts traffic based on economics e.g. it will direct you to a gas station that pays higher commission to Waze even if it is further away vs a nearby station. Should we allow companies to “buy” which roads we take?