Can artificial intelligence help address the complexities of non-artificial mind?

Can an app powered by machine learning be better and faster at diagnosing mental illness than humans?
Mental health: The challenge
Mental health is a behemoth of an issue; one that not only millions around the world continuously grapple with (in fact, depression will be the #1 most common chronic condition for Americans by 2050 [1]), but that the healthcare system has been struggling to provide adequate care for. The critical steps in treating this condition are diagnosis and timely intervention, which can be a challenge in today’s world where specialist appointments can take weeks to get and episodes of the illness can land patients in the Emergency Room, for lack of alternate timely intervention options.
Whereas traditional mental health diagnostic tools rely on extensive self-assessed and self-reported mood metrics at discrete points in time, Ginger.io processes a continuous stream of data gathered from a patient’s smartphone through machine learning to identify the potential onset of a mental health episode and the need for intervention. This data includes phone movement data, screen usage data, text and other communication data, and self-reported mood data [2]. The algorithm learns the typical patterns within these data for each patient and uses machine learning to identify abnormalities which signal a likelihood of mood disorder. The algorithm was built by contrasting data from these sensors with responses to 1.4 million mental health assessment questionnaires, and continues to learn as users continue to complete these assessments in-app [3]. This app has been proven to identify changes in mood and signs of depression before a patient even realizes it has occurred; in several cases, up to 2 days before [4]. In response, the app can help virtually engage a coach with targeted therapy before outward symptoms even manifest.
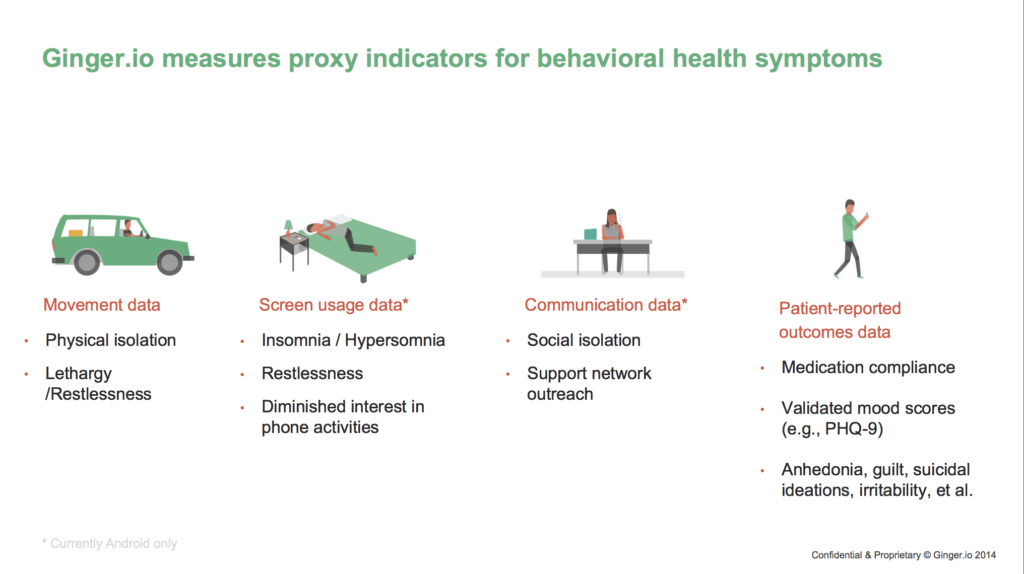
This approach is a very clever application of machine learning. The input data comes in several forms and combines several datasets containing different variables to identify patterns. Deviation from historical data and behavior, while entirely possible that it is not a sign of mental health illness, can be a very good indicator of overall mood in this case. Because the prediction of mood is not absolute, but rather suggestive to initiate outreach of a coach, this problem lends itself very well to the benefits of machine learning.
Strategic recommendations for the future
The company began by offering its technology to hospitals, but quickly pivoted to scaling their impact by selling their service directly to consumers and integrating into platforms of medium and large self-insured employers as well as United Healthcare’s insurance plans [5]. In the longer term, the company is working to offer better access, expanding its suite of therapists and coaches on staff to offer a fuller stack of service and improving training of its coaches and accuracy of its algorithm through continuous in-app feedback.
My recommendations are two-fold: 1) On the algorithm side, to integrate additional datasets, including language processing and social media data and 2) On the strategic side, to sign up more integrated delivery systems and ACOs.
On the algorithm, we input near infinite amounts of data into our phone in the form of language, from the words we use to text to the keyword searches we look up. The app could implement additional natural language processing and sentiment analysis to better predict mood. In addition, there have been several studies about the impact of social media and mental health; for example, one study found that increased use of blue and gray-toned colors in a person’s Instagram posts is correlated to depression [6]. For this reason, the algorithm could evaluate and analyze social media use and content further to distinguish additional nuances in mood.
On the strategy, the company could take even greater advantage of their value proposition to reduce healthcare costs by marketing towards payers that are taking on risk for the cost of care of populations: for example, integrated delivery systems (such as Kaiser Permenante), ACOs (such as Chen Medical), concierge medicine programs (such as One Medical), and even Medicare or Medicaid programs.
Open questions
Although ginger.io shows promise as a potent treatment for non-serious mental illness and has proven hospitalization avoidance [4] and clinically significant improvement in depression [5], an open question remains in my mind. How much can we rely on machine learning to identify when a patient with mental illness needs help? Will an app like this make others, such as the medical care team or the personal support network of a patient, abdicate responsibility and assume that an app will alert them if and when someone may need intervention? To what degree will this impact interpersonal relationships and community as we know them today? With all the hullabaloo about technology ruining society as we know it, will this app just take us another step in that direction?
(Word count: 770 words)
References:
[1] 1. Heo M, Murphy C, Fontaine K, Bruce M, Alexopoulos G. Population projection of US adults with lifetime experience of depressive disorder by age and sex from year 2005 to 2050. Int J Geriatr Psychiatry. 2008;23(12):1266-1270. doi:10.1002/gps.2061
[2] Hari Prasad, “Behavioral Analytics for Healthcare”, 2014. http://www.caltelehealth.org/sites/main/files/file-attachments/hariprasad.pdf, accessed November 2018.
[3] Sean Captain, “Can Artificial Intelligence Help The Mentally Ill?”, July 21, 2016. https://www.fastcompany.com/3059995/can-artificial-intelligence-help-the-mentally-ill, accessed November 2018.
[4] World Economic Forum, “Empowering mental health care patients through personalization”, 2018. http://reports.weforum.org/digital-transformation/ginger-io/, accessed November 2018.
[5] Ginger.io press; “Ginger.io to Expand Access to Emotional-Support Coaching Through Ginger.io App For BuzzFeed Employees Outside of the United States”, July 27, 2018. https://globenewswire.com/news-release/2018/06/27/1530424/0/en/Ginger-io-to-Expand-Access-to-Emotional-Support-Coaching-Through-Ginger-io-App-For-BuzzFeed-Employees-Outside-of-the-United-States.html, accessed November 2018.
[6] University of Vermont, “When you’re blue, so are your Instagram photos: Researchers discover an early-warning system for depression in social media images”, August 9, 2017. https://www.sciencedaily.com/releases/2017/08/170809083209.htm, accessed November 2018.
Me – Thank you so much for sharing this, Ginger.io is an incredibly interesting product offering for the mental health space and a competing product to the topic of my submission, Mindstrong Health.
The suggested change to include additional data sets such as social media is one point that I believe merits additional discussion. I would be concerned about machines evaluating content rather than just how we engage with content. False positives can easily arise if we start to evaluate content as well so this would have to be monitored carefully. I would also be concerned users would not want to adopt the product if they were monitored on the content they viewed?
Ginger.io is an exciting product in a competitive space and can play a meaningful opportunity in improving the massive mental health problem.
I think you posed some incredibly interesting questions around responsibility. Will this, in the future, become the sole determinant of future help in avoiding mental health episodes and if so, who takes blame for missing an episode. My opinion would be that this would always have to act as a supplemental technology and never a sole indicator. That doesn’t mean however that it’s importance can’t grow, but it should not take over responsibility fully even if it gets better than humans at predicting such an episode.