Big Data in Sports: How S.L. Benfica is Using Machine Learning to Build a European Football Powerhouse
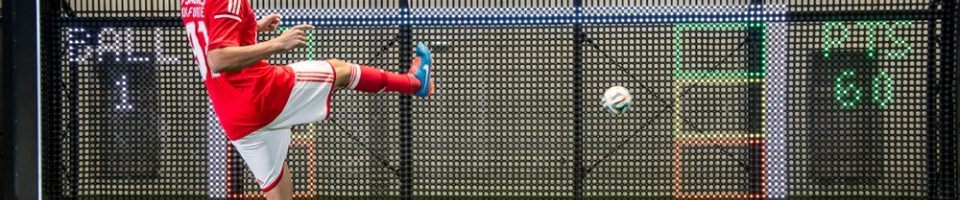
This article explores the use of machine-learning to optimize talent development and team management in European football.
Benfica
With 2 European cup titles and a record 36 Primera Liga victories, including four titles in the last five years, Sport Lisboa e Benfica (“Benfica”) is widely regarded as the most successful football club in Portuguese history [3]. A key driver of the club’s recent success has been its heavy investment in technological infrastructure at its Caixa Futebol Campus, a training center located on the outskirts of Lisbon. Fitted with state-of-the-art sensors and GPS tracking systems, this facility is being used to create one of the largest repositories of athletic performance data in Europe today [1]. In 2016, Microsoft Azure launched an engineering/technology partnership with Benfica to explore new ways in which the club’s copious amounts of accumulated data could be harvested and analyzed [2]. Together, this partnership is yielding a promising data machine that could revolutionize talent management in European football.
Competitive Pressures in European Football
While football has long been the most popular sport on the planet, growing viewership rates in previously untapped markets (such as the US and China) have increased the value of broadcasting rights for the world’s top leagues. Consequently, European football clubs have become extremely lucrative investments for global entrepreneurs and investors. Along with the massive inflow of capital into the sport, the cost of acquiring and developing top talent has also risen meaningfully as some of the best players have commanded transfer fees of over €200 million in recent years. With the advent of rising costs, clubs like Benfica have shifted their focus to developing and nurturing homegrown talent. Moreover, the frothy market for young, talented football players has allowed Benfica to monetize its core capability as a talent incubator by selling its players for a profit [1]
Benfica’s Big Data-Driven Solution
At Caixa Futebol, players in Benfica’s three professional teams practice on sensor-laden pitches that closely track individual player movement, speed, agility accuracy, heart rate, etc. [3]. Players’ sleep patterns and nutrition are also closely monitored, and all data is transmitted into a vast “data lake” hosted by Azure. Data scientists use these voluminous amounts of data to identify trends, patterns, and relationships between players’ habits and on-the-field performance. Coaching staff also use the insights gleaned from this data to develop personalized training programs for individual players, focusing on developing their strengths and working to improve areas of weakness [1][3]. Fitness staff also use predictive analytics to determine the likelihood of player injuries, aiding in roster selection for high-profile games [3].
In the medium term, Benfica and Azure are exploring innovative ways of collecting and analyzing data to grow the size of the “lake” and perform a broader range of analytics that optimize team management. Capability targets include predicting future fitness and performance, which will allow the team to strategically plan its line-ups for competitive tournaments. The club is also seeking subtler, “less invasive” data collection devices that players can wear during practice to replace the current bulky sensor systems [1]. More sophisticated monitoring equipment will also expand the data collection capabilities and minimize data integrity issues stemming from the use of elementary sensors.
What Does the Future Hold?
Going forward, it is critical to maintain the pace of investment in research and development to identify new ways to streamline data collection, and potentially expand the number of environments in which relevant data can be harnessed. While the focus is currently on monitoring player behavior during training at Caixa Futebol, Benfica and Azure should invest in hardware solutions and other technology to better track data on the pitch during actual games. This will complement current team optimization efforts by allowing for real-time analysis and data-driven decision-making during – rather than before – competitive games.
Open Questions
As Benfica’s data-driven machine becomes the cornerstone of the club’s talent-development strategy, several questions remain about the extent to which it can be relied on. For example, data points fed into the analytical tools are based on relatively small sample sizes when compared to the entire quality spectrum of football players. The extent to which these insights can be generalized and applied to subsequent generations of Benfica players will depend on the machine’s ability to learn and develop a constant feedback loop. Would Benfica need to expand enrollment at its academy to mitigate this? What lessons can be gleaned from the use of machine learning in more technically advanced sports? While Benfica may be one of the earlier adopters of machine-learning, to what extent will this translate into a sustainable competitive advantage, particularly as larger teams catch up to this model?
(Word count: 754)
Sources:
- Sebastian Anthony. “Football: A deep dive into the tech and data behind the best players in the world” Net, 2017. Ars Technica, https://arstechnica.com/science/2017/05/football-data-tech-best-players-in-the-world/
- “The unlikely secret behind Benfica’s fourth consecutive Primeira Liga title” Net, 2017. WIRED, https://www.wired.co.uk/article/microsoft-sl-benfica
- Harry Petit. “How Benfica uses technology and data science to be one of the world’s best football clubs” Net, 2017. Daily Mail, https://www.dailymail.co.uk/sciencetech/article-4544900/How-world-s-best-football-clubs-use-data.html
Compelling look into taking sports Analytics, one step further into AI. As to the final question, I definitely believe that this can be a sustainable competitive advantage for Benfica, as long at they keep developing the tool. For this system to be useful, a key component will be the amount of data, which will only grow the longer they maintain it. They have first mover advantage and so if they keep refining the system and ideally sustain a dedicated team, they could build it to strengthen an ever-growing features set.
It’s a very comprehensive article to summerize the machine learning technology implementation at a top football club.
Along with the questions mentioned at the end of article, I have two more concerns:
1. The data gathered during the training may not fully reflect the whole picture of a player’s talent. You may see a player who is very moderate in training, but will be very energetic and excellent during an official match. So the hardware to capture the during match performance is mandatory to conduct this research.
2. The players have a learning curve, we can use machine learning to predict the players’ future potential based on their current data. This requires a big sample size, which includes data from the current stars and data from their youth. This may take a long time to gather, otherwise, the results now may somehow not very accurate.
Thanks for your comment, and completely agree with (2). With (1), my only concern is that it may be difficult to capture the data during the match and still maintain its proprietary nature. Much of the data is captured using high-tech cameras. So I am not sure whether there is a way to single out Benfica players using such a camera, without capturing the opposing team’s data. Also, domestic leagues pay clubs for the exclusive right to record competitive games, so these cameras would have to be embedded in the League’s infrastructure (and not Benfica’s)
Interesting to see the interplay between hardware and software used at Benfica. Collecting this data seems like a rather complicated undertaking. This model does indeed sound ideal for a club like Benfica, which is known for developing young players and selling them off to larger clubs in more respected leagues. I can definitely see analytics and machine learning helping Benfica identify the most talented players early on, and in a way weed out those who are not cut out for the top leagues. However, can algorithms really identify top talent potential? Given the fact that these algorithms make decisions based off of historical data, are they not inherently biased to past performance, therefore making it difficult for them to accurately predict the future? If anything, we see athletes in sports like Michael Jordan and Tom Brady, who initially showed average potential, but ended up being some of the great in their respective sports.
Completely agree with the inherent bias point. The data machine does indeed rely on precedent indicators of success, which – as you said – are not necessarily foolproof.
Titi, I really enjoyed this piece. Unlike other sports, in football data and analysis have not become mainstream and are only use by a handful of teams and managers. I think there is a big opportunity for advancements like these to make a very big positive impact on the game. I think being able to measure a player’s performance and physical condition during a match in order to make data driven decisions, just like it is done in basketball, is where the sport needs to go and the fact that a team like Benfica is supporting it could be the major breakthrough.
Completely agree. More teams should adopt similar models – Leceister City apparently has since 2014.
Great read! It is fascinating to see football clubs taking advantage of data analytics and machine learning to improve their performance. It is a great evolution from the time when clubs hired people to count how many minutes each player kept the ball, how many passes they completed, and so on. I believe that discipline and tactics informed by machine learning analysis can be a competitive advantage in soccer. Germany, the 2014 world champion, is a good example of a team that relied on a very tactical and disciplined game, informed by data, to win the biggest soccer tournament.
Interesting article! I particularly enjoyed your description of the natural disadvantages a “small market team” like Benfica faces in acquiring and retaining its top players and almost seeing them as investments. Getting them cheap, developing them and selling them for a profit. I think you raise some valid concerns about the future of Benfica’s edge. You know that the bigger market teams will eventually catch on to what Benfica is doing and replicate it, similar to how the Oakland A’s Moneyball model was copied and now used by most baseball teams. Also it’s hard to know how reliable the data will be if the data set isn’t large enough.
Great article. As data becomes more pervasive in all aspects of our life, it becomes critical to collect as much quality data as possible in order to detect what are the key variables that will create a competitive advantage. As you highlighted, they currently have little data to have enough of a sample set to isolate key performance drivers. Many times, clubs sponsor junior league clubs which might be a prime arena to incorporate sensors and understand what are the key variables that drive players to become more successful.
Great read. I actually almost wrote on the Benfica as well. I think what they are doing is fascinating and will become the standard across professional sports as teams become increasingly sophisticated with their training and data analytics. I also was confused why this technology isn’t used in their home stadium. As far as generalizing the data and creating a larger data set, my thought was they could try to partner with other clubs in different countries to share data through Azure on an anonymous basis.
I like your idea about partnering with other clubs to share data anonymously. I think the reason they don’t have this technology in their home stadium is that they sell the broadcast rights for each game to the league/competition hosting the game. Therefore, they relinquish the right to record matches using their sensor-laden cameras. There’s also the issue of recording and obtaining the other teams’ data, which may open up some legal questions. But it will be interesting to see what new innovative methods they come up with for harnessing more relevant data.
Super interesting article, really enjoyed it! While I do think that machine learning can yield benefits in terms of estimating likelihood of injury, or developing a personalized sleeping schedule and nutrition plan, I am less bought into the upside of taking it a step further to, for instance, prepare the optimal starting line up for a top team. This is because I am wary of its ability to capture exogenous data inputs. For instance a player’s on the pitch performance can be strongly affected by his on-the-day psychological state, which in turn can be affected by his personal life, the crowd, a successful tackle/drible early-on in the game, etc. These influences are hard to predict/capture in a model and can lead to confounding/limit the model’s ability to predict performance. That being said, I think there is opportunity to mitigate this lack of data by collecting a player’s health during the match.
Finally, I also question the ability of machine learning to identify future talent given the different development patterns of different players (i.e. some players physically/mentally develop superior abilities later in life).
Very valid points and completely agree with all of them, especially the point on future talent indicators. Another comment mentioned the fact that several star-athletes in basketball and other sports were mediocre in their youth, and only started to blossom later in their careers. The model, as it currently stands, would fail to recognize that kind of talent early on.
Great article highlighting how Benfica has leveraged machine data to adjust their strategy as a club and improve player performance over time. I think this use of data analysis is incredibly unique in the footballing world and over time will be seen across most of Europe’s top clubs. Given the initial repository they have built up, do you think Benfica should license their model to other clubs to establish it as the gold standard? I wonder if other clubs build their own proprietary models that emphasize different variables, Benfica’s primary means for negotiating player transfer fees might be questioned.
Good questions. I fear that by licensing out their model, Benfica may eliminate the comparative advantage they have in talent development. That said, they could potentially make up for it in licensing fees.
Awesome article on machine learning applied to football — I think you raise great questions about how this data can be translated into improved player development and performance across the different teams for Benefica. I’d be really interested in learning how the insights from the data can also help the other core element of the player development process – scouting and choosing the right youth players to enter the academy.
Thanks for the interesting read TH14. I think the methodology that Benfica is using to analyze its young players is revolutionary to the sport, but I do question how this can be effectively translated into a feedback loop. As some of the commenters above mentioned, there is a gap between when you are measuring the characteristics of the players (in development camp) and their actual performance. I would think that player’s characteristics will change over time, and this could miss players who develop at a later time. I also worry that the technology will never be nimble enough to be used in a game setting, and therefore will not truly be reflective of game preparedness. I do however see how this could be one of a set of tools used to help analyze young talent.
Very interesting article and trend. Many basketball and baseball teams are using similar methods. Currently, teams like the Boston Red Sox use predictive analytics to forecast a player’s future performance. This has had a major impact within baseball since teams are now not only paying players based on their past performance, but also leveraging forecasting data to determine a player’s future value.