Autonomous Stock Replenishment at Online Retailer OTTO
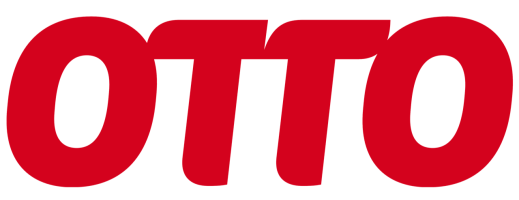
How a leading German E-commerce player has employed machine learning algorithms to forecast with 90% accuracy what SKUs will be sold over the next 30 days and reduce inventory levels by 20%.
“Through the artificial intelligence algorithm, our forecasts are constantly improving. This way, we get precise results that help us plan and control a future-oriented product range.”1 Mathias Stüben, Director Category Processes, OTTO
With the proliferation of online shopping data, the advances in data science and the ease of access to machine learning solutions, the e-commerce landscape is rapidly changing2. With €3.0bn of sales, OTTO is Germany’s second largest e-commerce retailers behind Amazon (€8.8bn) and ahead of Zalando (€1.3bn)3. The company is predominately active in the highly seasonal fashion and electronics retailing categories and offers 2.2M SKUs, approximately 50% of which are sourced from brand partners.
In a highly competitive e-commerce environment with increasing cost pressures4 and customers expecting OTTO’s own and partner-branded products to be available immediately, how can OTTO offer fast product delivery while ensuring that its inventory levels are minimized and no stock is left at the end of the season?
Autonomous Product Replenishment through Machine Learning
In order to balance product availability and inventory levels, OTTO has begun to utilize the machine learning algorithms of Blue Yonder, a data science and machine learning company it helped back and whose algorithms originally stem from elementary particle experiments at the CERN laboratory in Geneva5.
The algorithms use hundreds of different input variables including internal data (historical sales, traffic and searches, buy/no buy decisions, placement on website, location-based parameters) and external data (competitor data, weather forecasts, day of the week and public holidays) to predict precise SKU-level sales forecasts on a daily basis1, 6.
OTTO’s daily product replenishment function for partner-branded products, traditionally performed by humans based on data, intuition, and domain knowledge7 , is now driven by the algorithm. As a result, the application has allowed OTTO to predict with 90% accuracy on any given day what items will be sold over the next 30 days and reduce inventory levels by 20%5.
While competitors such as Amazon have deployed machine learning predominantly to inform its customer recommendation engine, OTTO is already advancing the use of machine learning by adopting a fully autonomous and data driven replenishment function5.
Recommendations for OTTO’s Management
Initially, I believe OTTO should drive further cost-savings across a range of tasks including autonomous staffing plans in distribution centers based on sales forecasts and transportation cost reductions through pre-arranged shipments based on expected customer demand. Moreover, OTTO should expand the application of its machine learning algorithm from its fashion and electronics products to its furniture and home wares portfolio as these products carry higher inventory costs.
Over the medium term, OTTO should seek to expand machine learning across their entire business from product development and manufacturing through to supply chain management and customer engagement. For example, machine learning algorithms should help OTTO advance their product catalogue by identifying trends in social media, customer behavior and transaction data. These unique insights will help OTTO differentiate its own product development from CPGs who are further upstream in the supply chain and do not have full order visibility. Moreover, the company should leverage its daily rolling customer demand forecast in its production process to build products by size and color “just-in-demand”. Also, the algorithms will re-shape OTTO’s supply chain configuration and decision making as long-term sales forecasts will drive the placement of its distributions centers. Finally, machine learning algorithms will enable OTTO to develop a total view of the customer, which will enable it to build deeper relationships and improve customer engagement and loyalty.
Considerations
OTTO is advancing the use of machine learning in its operations, a move that is seen as extremely unusual by industry experts5 and that will have sparked intense internal debates, particularly as a meaningful portion of the retailer’s staff is employed in merchandising and replenishment functions. With its radical shift to autonomous decision-making, how can OTTO avoid technology fear among employees and stakeholders and build a culture that embraces technology-led innovation8?
Moreover, although Blue Yonder’s machine learning and OTTO’s autonomous deployment of the algorithms are currently serving as a competitive advantage, how sustainable is that advantage when other retailers replace core functions with machine learning algorithms? How would a world look like in which product assortment, pricing and customer experience across retailers are driven by the same algorithms?
(703 words)
References
- Blue Yonder OTTO Case Study, “Replenishment and Price Optimization at OTTO,” https://www.blueyonder.ai/sites/default/files/by-case-study-otto-en.pdf, accessed November 2018.
- David Simchi-Levi, Michelle Xiao Wu, “Powering retailers’ digitization through analytics and automation,” International Journal of Production Research, Jan2018, Vol. 56 Issue 1/2: 809–816.
- Ecommerce News, “Top 100 online shops in Germany 2018”, Ecommerce News, https://ecommercenews.eu/top-100-online-shops-in-germany-2018, accessed November 2018.
- John R. Yuva, “Consumers Sit in THE E-COMMERCE DRIVER’S SEAT,” Supply & Demand Chain Executive, Sep2017, Vol. 18 Issue 4: 6–10.
- The Economist, “How Germany’s Otto uses artificial intelligence,” The Economist, https://www.economist.com/business/2017/04/12/how-germanys-otto-uses-artificial-intelligence, accessed November 2018.
- NOAH Conference 2016 Presentation Blue Yonder, https://de.slideshare.net/NOAHAdvisors/blue-yonder-noah16-london, accessed November 2018.
- Anastassia Fedyk, “How to tell if machine learning can solve your business problem,” Harvard Business Review Digital Articles, (November 15, 2016).
- Andy Hawkins, “Machine learning and the supply chain”, Logistics & Transport Focus. Sep2018, Vol. 20 Issue 9: 30–31.
Coincidentally I just read another piece on Amazon Go, where a similar automated replenishment capacity will likely take hold, albeit less via predictive models, more by analyzing real time consumer behavior. It seems likely that in a fairly short time, all e-commerce companies will employ some level of automated replenishment – does OTTO even have an exclusivity agreement with Blue Yonder? I’d be very curious to hear how a data analytics firm with its roots at CERN lab ended up forecasting fashion orders. But I digress.
Does the company have any sort of predictive algorithm for product returns? Usually returns are huge cost factors for these types of e-commerce companies. I’d be curious to know if return predictions are forecasted and included in future replenishment plans.
I like this article! I was not aware that Otto was using machine learning to this degree. I find your second question (is this advantage sustainable) super interesting. Businesses are moving towards a world of automation in which the efficiency of a company is mainly driven by the data available and the quality of the AI/ML algorithms. Large companies will have more data and therefore an advantage (at least temporarily). But the main sustainable advantage will be the ability of a company to hire people who are able to develop superior algorithms. Companies will therefore need to switch from algorithms provided by 3rd parties to algorithms that they developed in-house and are better than the rest.
Thanks for a great read! I think you brought up a great question about what other portions of its business OTTO can apply machine learning techniques. I really liked your suggestions regarding manufacturing, supply chain management and customer engagement but paused a little at your suggestion regarding product development. I’m skeptical about how machine learning can deliver a sustainable competitive advantage in product development given that its main strength is in predicting outcomes based on existing data sets and in my view, building great new products is only partially related to what was successful in the past and therefore predictable . I think a larger part of successful product development in fashion relates to having a new/better/more creative vision for the future than you had in the past, and the ability to deliver this vision, season after season, is what generates an enviable competitive advantage in fashion. I’m curious about how to leverage machine learning in more “creative” processes like new product development in fashion.