A Tech Titan in Healthcare: All Eyes on Deep Learning
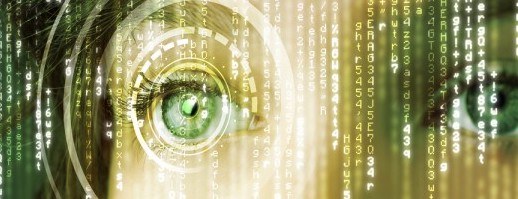
In the realm of deep learning, your eyes can be a window to your health. Google aims to leverage machine learning for high tech healthcare solutions.
In an industry undergoing transformation in the modern era of computing, technology giant Google is bringing machine learning to the forefront of medical discovery. Using deep learning algorithms to parse through patient data, Google researchers have produced preliminary results demonstrating predictive capabilities of artificial intelligence (AI) for clinical diagnosis. By mobilizing its machine learning expertise in the nascent area of AI-based healthcare, Google is uniquely positioned to disrupt an industry where innovation traditionally errs on the side of caution.
The company’s application of deep learning in medical research enables it to synthesize a massive volume of patient data across countless variables and identify areas of opportunity for product development. This technology has the potential to drive new innovation in patient screening, diagnosis, and treatment.
In a recent study using retinal fundus images of 284,335 patients to train a deep learning model, Google researchers were able to predict a number of cardiovascular risk factors, including age and smoking status within 3.26 years and 71% accuracy, respectively.[1] The researchers also used a set of 12,026 patients, of which 150 experienced a major cardiac event, to determine the model’s accuracy in predicting the onset of said event. The algorithm’s accuracy score was 70%, comparable to the current standard’s accuracy of 72%.[2]
In another study targeting retinal diseases, Google DeepMind researchers developed a deep learning model with referral recommendation performance rivaling that of experts after training on 14,884 optical coherence tomography scans.[3] The results have promising implications for early diagnosis of sight-threatening conditions.
Google’s studies are powerful demonstrations of machine learning as a platform for its AI-based healthcare pipeline.
Clinical implementation of this technology, however, requires Google to address the core issue of trust—from physicians, regulatory bodies, and patients. The “black box” dilemma of AI—in which scientists currently do not fully understand how complex AI models encode information—poses a significant problem to its adoption in healthcare. An algorithm may implicitly learn to recognize predictive disease markers that even the scientists are unaware of, but if the machine cannot explain this knowledge, patients and physicians will be reluctant to act on its recommendation. The understanding behind the answer is built into the network, rather than the scientist.[4]
At the study level, Google has sought to unveil the underlying mechanisms of its deep learning algorithms. In the two studies mentioned earlier, the algorithm for the retinal disease study indicated features in the scans that could result in ambiguous interpretation; in the cardiovascular risk factors study, the model highlighted which anatomical structures were used as the basis for the algorithm’s predictions.[5]
Another major impediment to the development of a robust and explainable model is the limited set of available data—a challenge that Google has taken steps to address. Google’s DeepMind has partnered with Moorsfield Eye Hospital in London in a research endeavor that has provided Google with enriched access to data in the near term, as well as potential for broad usage across the UK in the long term, depending on the technology’s validation status.[6]
In a partnership with Verily, Duke University, and Stanford Medicine, Google has also started Project Baseline, a study to collect and analyze data from 10,000 participants over the span of four years. This project is expected to provide a diverse and continuous set of data across a broad range of health-related measurements, including clinical, molecular, behavioral, psychological, and more.[7]
In order to build upon the current momentum of machine learning in healthcare, Google can also benefit from using rigorous and targeted reverse engineering methods on its models. A comprehensive explanation for solutions given by machine learning may not necessarily exist; complex phenomena may not always boil down to an organized set of parts. For instance, it is difficult to verbalize precisely why years of experience can make someone a better doctor.[8] However, by developing a systematic analysis of the algorithm’s approach to a recommendation, researchers will be able to present a more compelling case for the clinical application of this technology.
To ease the adoption process of machine learning in healthcare, Google can also establish new joint research initiatives with incumbent leaders in the industry, including both companies and regulatory bodies. Introducing interpretable models with incremental levels of complexity, and validating these models across larger patient populations, will be critical in gaining the trust of the medical community and bringing machine learning closer to clinical implementation.
The performance of Google’s deep learning algorithms in healthcare has been promising thus far, but Google is far from replacing physicians with machines in the patient journey. The clinical decision rendered must still be driven by human judgement, but at what point in the physician’s decision-making process should the algorithm enter?
(779 words)
References
[1] Loria, Kevin. “Google Has Developed A Way To Predict Your Risk Of A Heart Attack Just By Scanning Your Eye.” Business Insider, February 20, 2018. https://www.businessinsider.com/google-verily-predicts-cardiovascular-disease-with-eye-scans-2018-2, accessed November 2018.
[2] Poplin, Ryan, Avinash V. Varadarajan, Katy Blumer, Yun Liu, Michael V. McConnell, Greg S. Corrado, Lily Peng, and Dale R. Webster. “Prediction Of Cardiovascular Risk Factors From Retinal Fundus Photographs Via Deep Learning”. Nature Biomedical Engineering 2 (3): 158-164, 2018. doi:10.1038/s41551-018-0195-0, accessed November 2018.
[3] De Fauw, Jeffrey, Joseph R. Ledsam, Bernardino Romera-Paredes, Stanislav Nikolov, Nenad Tomasev, Sam Blackwell, and Harry Askham et al. “Clinically Applicable Deep Learning For Diagnosis And Referral In Retinal Disease”. Nature Medicine 24 (9): 1342-1350, 2018. doi:10.1038/s41591-018-0107-6, accessed November 2018.
[4] Castelvecchi, Davide. “Can We Open The Black Box Of AI?”. Nature 538 (7623): 20-23, 2016. doi:10.1038/538020a, accessed November 2018.
[5] “Towards Trustable Machine Learning”. Nature Biomedical Engineering 2 (10): 709-710, 2018. doi:10.1038/s41551-018-0315-x, accessed November 2018.
[6] Suleyman, Mustafa. “A Major Milestone For The Treatment Of Eye Disease | Deepmind”. Deepmind, August 13, 2018. https://deepmind.com/blog/moorfields-major-milestone/, accessed November 2018.
[7] “Project Baseline – Verily Life Sciences”. Verily, 2018. https://verily.com/projects/precision-medicine/baseline-study/, accessed November 2018.
[8] Castelvecchi. “Can We Open The Black Box Of AI?”, accessed November 2018.
Explanatory artificial intelligence (XAI), as you’ve highlighted, is crucial to gaining trust across various clinical entities. To your point about when the algorithm should enter, I believe recent XAI research [1] into decision tree modelling and automated rule extraction may provide some clues. Intervention into the decision making process is really a subset of the supervisory capability of a machine learning model to understand where in the overall process of diagnosis/intervention the physician is.
Put another way, understanding the decision making workflow of a physician, and mapping this to the ML capabilities of a parallel decision making system, provides a good way for a human to query an ML system as they see fit. Alternatively, the ML system can also serve in an advisory (or early warning) capacity to prevent a physician from deviating from some optimal decision making path and nudge them towards an optimal solution.
Your point about annotation by a human expert also underscores the importance of how ML models should sequence data interpretation into buckets that mirror their clinical workflow counterparts (especially during the R&D design process). This enables not only regulatory and clinical bodies to communicate functional capabilities/limitations of the system to others, but also engineers to trouble shoot and benchmark system capabilities to gold standards.
In this way, I feel that designing ML models for healthcare and enabling transparency are really two sides of the same coin that, if implemented correctly, can hit two birds with one stone.
[1] https://arxiv.org/pdf/1806.00069.pdf