Machine Learning at PredPol: Risks, Biases, and Opportunities for Predictive Policing
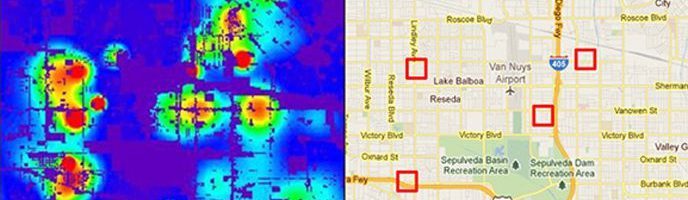
Law enforcement agencies are using machine learning tools to analyze, track, and attempt to predict crime.
Machine learning and artificial intelligence (AI) have the potential to revolutionize systems and power structures that have existed in society for generations. However, the performance of AI-enabled tools can either be limited or enhanced by the quality and quantity of the data on which their algorithms are based. This paper examines the role of machine learning in creating law enforcement tools to analyze, track, and ultimately attempt to predict crime. Predictive policing refers to the use of analytical techniques by law enforcement to make statistical predictions about potential criminal activity [1]. A 2011 report published by researchers at Harvard Kennedy School and the National Institute of Justice described the advancement of science (defined in this case as both technology and social science) in policing as essential to the retention of public support and legitimacy [2]. The success of these new tools is contingent on their ability to reconcile machine learning solutions for the future with a long history of biased practices and data from the past. PredPol is a private technology company based in Santa Cruz, California that aims to predict crime using cloud software technology that identifies the highest risk times and places in near real-time [3].
PredPol’s value proposition to law enforcement agencies is three-fold. First, the company offers predictive policing through its machine learning algorithm based on three data points: crime type, crime location, and crime date/time. The algorithm functions by predicting crime in 500 square foot boxes with the number of boxes deployed in each shift calibrated to the policing resources available. Second, their platform provides mission planning and location management services that use the algorithm results to determine high probability crime locations and track “patrol dosages” or the amount of time officers spend in PredPol boxes. Finally, PredPol offers an analytics and reporting module that allows for custom reports by any combination of crime types, missions, districts, etc., over a defined date range (See Exhibit 1 for a visual representation of services from PredPol) [4]. PredPol is currently being used by more than 50 police departments across the United States and a few forces in the UK [5]. The company appears energized by the success of their product in law enforcement and is actively entering new markets. This year, PredPol announced a new product offering for private sector and corporate customers with an emphasis on security, patrol, and prevention. They also announced a partnership with one of the largest sheriff departments in the country to tackle the Opioid epidemic. PredPol intends to leverage data from their machine learning algorithm around specific crime patterns to identify areas that signal higher risks of overdoses. Their goal is to share this data with partner entities (faith-based organizations, community groups, etc.) to take a proactive approach to solving the Opioid crisis [6].
Though PredPol’s efforts to leverage technology to make communities safer is admirable in theory, critics have expressed concern about the potential for algorithmic discrimination, ethical issues, and sociological implications. PredPol claims that no demographic, ethnic or socioeconomic information is ever used in its algorithm, thus eliminating privacy or civil rights violations [7]. However, this claim is predicated on the assumption that the data supporting the algorithm is also free of demographic, ethnic or socioeconomic information. In an article from statistics magazine Significance, researchers Kristin Lum and William Isaac argue, “using predictive policing algorithms to deploy police resources would result in the disproportionate policing of low-income communities and communities of color.” [8]. Leslie Gordon, a contributor to the American Bar Association Journal, agrees. She raises concerns about the potential for this technology to violate the fourth amendment and further stigmatize neighborhoods that are already heavily patrolled [9]. For the Los Angeles Police Department, an early adopter of predictive policing (using PredPol and services from other firms like Palantir), the controversy reached a turning point this summer. The Stop LAPD Spying Coalition released a report this past May critiquing the department’s data policing techniques and alleging that the data is biased against blacks and Latinos. The department uses multiple machine learning tools that employ targeting strategies that are people-based and place-based. The current police chief, Michel Moore, supports an inspector general audit of the existing practices to improve relations between his force and the community [10].
As techniques in machine learning and predictive policing evolve and allegations of algorithmic bias persist, PredPol’s management will need to consider how to manage risk, avoid misuse of its technology, and evaluate the potential for bias in its existing algorithm. I offer two questions that merit further reflection on this topic: What measures should be taken from a governance perspective to ensure that PredPol’s predictive policing algorithm is fair and unbiased? Should citizens be alerted when predictive policing algorithms are implemented in their communities?
[791 words]
Exhibit 1
Source: Law Enforcement at PredPol
Predictions displayed as red boxes on a web interface via Google Maps.

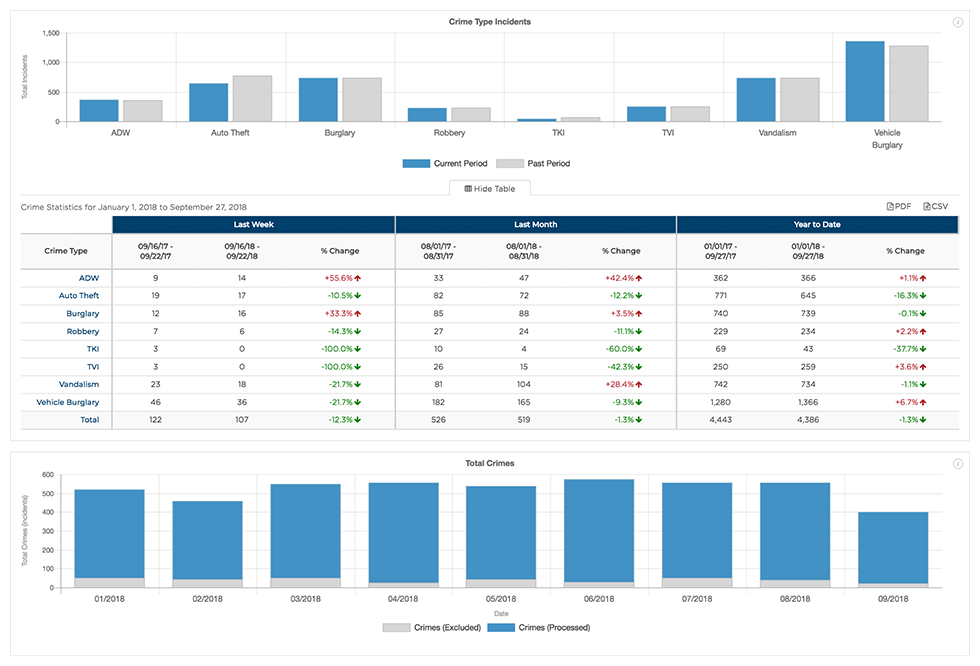
References
[1] RAND Corporation (2013). Predictive Policing: Forecasting Crime for Law Enforcement. [online] Santa Monica. Available at: https://www.rand.org/pubs/research_briefs/RB9735.html. [Accessed 12 Nov. 2018].
[2] Weisburd, D. and Neyroud, P. (2011). Police science: Toward a new paradigm. In: Executive Session on Policing and Public Safety. [online] National Institute of Justice. Available at: https://www.ncjrs.gov/pdffiles1/nij/228922.pdf [Accessed 12 Nov. 2018].
[3] Crunchbase. (2018). PredPol Overview. [online] Available at: https://www.crunchbase.com/organization/predpol#section-overview [Accessed 12 Nov. 2018].
[4] PredPol. (2018). The Three Pillars of Predictive Policing. [online] Available at: http://www.predpol.com/law-enforcement/ [Accessed 12 Nov. 2018].
[5] Smith, M. (2018). Can we predict when and where crime will take place?. BBC News. [online] Available at: https://www.bbc.com/news/business-46017239 [Accessed 12 Nov. 2018].
[6] Attacking the Opioid Overdose Epidemic One Prediction at a Time. (2018). [Blog] PredPol Blog. Available at: http://blog.predpol.com/attacking-the-opioid-overdose-epidemic-one-prediction-at-a-time [Accessed 12 Nov. 2018].
[7] PredPol (2018). Science and Testing of Predictive Policing. White Paper. [online] PredPol. Available at: http://www.predpol.com/how-predictive-policing-works/ [Accessed 12 Nov. 2018].
[8] Lum, K. and Isaac, W. (2016). To predict and serve?. Significance, 13(5), pp.14-19.
[9] Gordon, L. (2013). A Byte Out of Crime: Predictive policing may help bag burglars—but it may also be a constitutional problem. ABA Journal, [online] 99(9). Available at: https://www.jstor.org/stable/i24595886 [Accessed 12 Nov. 2018].
[10] Chang, C. (2018). LAPD officials defend predictive policing. LA Times. [online] Available at: http://www.latimes.com/local/lanow/la-me-lapd-data-policing-20180724-story.html [Accessed 12 Nov. 2018].
This application of machine learning and AI is very interesting, as it ideally allows police to scale their presence while reducing human biases that lead to critical failures in judgement. As you mentioned, the issue is that any historical datasets used to train any AI or machine learning models are already seeded with bias which could lead to flawed algorithms. In order to avoid repetition of errors and the multiplication of biased machines, introduction of this technology needs to be done slowly, with excessive human monitoring and intervention. Unlike other applications, machine learning in enforcement needs to be more of a predictive guide and less of driving force, with multiple touch points available for human correction.
I enjoyed reading this article because the idea of bias in machine learning is very thought provoking. Just as in any industry, it makes sense for law enforcement to utilize big data and machine learning to better utilize their resources to keep the public safe. While removing prejudice and bias from data sounds on some levels easier then removing bias in real life, it obviously is quite difficult to do in reality. Moving forward I think it is very important that organizations work hand in hand with the partner entities identified in paragraph two. This is important not only to provide a comprehensive approach to solving the issue of crime, but also working with them to quickly identify when the machine learning algorithm may be displaying biases. Having partner entities work with police departments and PredPol engineers to identify potential police interactions that then become data points affecting bias in the learning algorithm, there is a higher likelihood that the PrePol engineers can correct the effect these instances have on the AI.
Thanks for bringing important issues like this one to our attention. Your piece raises the critical problem in using policing data for forward-looking predictions: the sampling of crimes that police departments detect and/or uncover is not necessarily representative of the crimes committed in the entire population. However, if utilized correctly, I do see ways that a larger, more in-depth set of data could lead to fairer policing over time. In the past we have only worked with quantity metrics like number of arrests in a community, without taking into account the number of patrol minutes per arrest or the average number of cars pulled over per arrest. If this data is properly tracked, these types of efficiency metrics could encourage departments to move away from heavy police presences in communities of color or other forms of overpolicing if they show poor returns. Achieving this outcome would also require that police officers are not incentivized or evaluated based on these efficiency metrics, but that they are only reviewed for insights and strategic decision-making.
To your first question, I think there are two measures that can be taken to analyze and combat the level of bias in PredPol’s algorithm. One is to conduct controlled experiments comparing the algorithm to a control as well as running simulations to assess hypothetical Type I and Type II errors that may result from the model. Upon detecting what may be some blind spots of the algorithm, human intervention protocols can be incorporated to confirm that the appropriate decisions are being made. Ultimately, given the bias inherent in policing data and the likelihood that it would result in the disproportionate policing of low-income communities and communities of color, both rigorous experimentation and a specific and effective human-machine partnership is necessary to ensure its longer term success.
Leila, thank you for sharing this interesting and potentially concerning application of machine learning and predictive analytics with respect to our communities. As someone who has spent most of my life in Los Angeles and the Bay Area, I had no idea that technologies like PredPol have been implemented in the communities I live in or around. I agree with Nancy that statistics and data science are ways to check against biases, particularly with respect to particularly race and socioeconomic status. I would further add that I think at a local, state, and federal government level, policies need to be enacted to regulate how these technologies are used — with input from experts in the field. Unchecked, I could see technologies like PredPol being used as “quantitative” proof reinforcing existing systemic bias.