Betting on machine learning to save lives
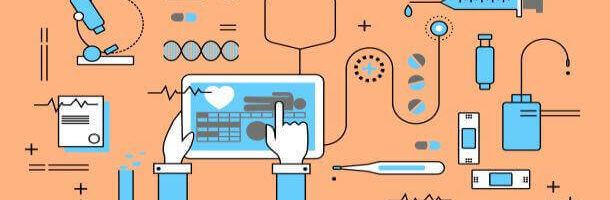
Machine learning is disrupting traditional industries such as transportation (ride-hailing) and financial services (credit scoring, robo-advisory). Can it also transform the traditionally human-intensive healthcare industry too?
In recent months, there has been a flurry of activity in healthcare deals, including CVS’s proposed US$69 billion buyout of insurer Aetna, Amazon, JPMorgan Chase and Berkshire Hathaway’s joint venture and Cigna’s acquisition of pharmacy benefits manager Express Scripts for more than US$50 billion[1].
Most recently, Oscar Health, an Obamacare health startup raised US$375M from Alphabet (Google’s parent company)[2] – bringing its total to US$1.3B – to use technology and design to make healthcare simple, intuitive and human.
The underlying promise of these deals is that data, with the help of technology can radically transform healthcare as we know today. The main technology in question is machine learning which was defined by Professor Arthur Lee Samuel as the “field of study that gives computers the ability to learn without being explicitly programmed”[3].
Machine learning is particularly important for Oscar as it seeks to guide a patient through the many hoops needed to get to the healthcare that they need in an efficient manner. One of the main questions that Oscar faces is whether its data is complete as it forms the basis for its vision. There are 4 main challenges on this front[4]:
- Data accessibility
- Data interoperability
- Data cleanliness
- Data structure
Data structure is crucial because doctors typically write patient visits and diagnosis in their own distinct manner which can be different from other doctors. Because of the subjectivity of such notes, it takes additional time and effort to fully understand the patient’s context.
However, with the proper data structure in place, patients’ data records can be “labeled” as seen in the example above. Through data labeling[5], Oscar can process data through machine learning models to develop clinical insights for doctors.
In June 2017, Oscar rolled out its Clinical Dashboard to provide a holistic data-backed view into a patient’s medical history, highlight key information and more importantly predict potential health problems[6][7]. This is made possible because of Oscar’s deep understanding of its patients journey through the healthcare system.
The Clinical Dashboard provides benefits to both doctors and patients.
For doctors, they will be able to see both confirmed diagnoses and machine learning-predicted analysis. The former allows a doctor to reduce time spent in understanding patients’ lengthy medical histories and administering duplicated tests and procedures. The latter enables a doctor to identify potential health problems based on predictive machine learning models even before performing any diagnosis. This can help to speed up doctor’s diagnosis and expedite any tests and procedures if required. The efficiency gains from the Clinical Dashboard can help to reduce cycle time at clinics and enable doctors to see more patients over the same period.
For patients, they would no longer need to bring a copy of their medical history to every single doctor’s appointment they go to or fill out lengthy forms detailing their medical history or allergies. This will lower the stress level of a patient who is already sick and yet needs to remember such information.
The potential downside of the Clinical Dashboard is whether its predictive insights are accurate. While other use cases of machine learning such as credit scoring in lending or shopping recommendations on Amazon.com do not require 100% confidence in its predictions, healthcare is one use case that requires 100% confidence level given its potential consequences. I believe it is in Oscar’s best interest to only display predictions which has a 100% confidence level while continuing to test and validate its less-accurate predictions through supervised machine learning[8] in the backend until they are ready for production use.
For Oscar to pass along the benefits of its game-changing technology to everyone in America, it needs to expand its operations to cover the entire country. It is doing so through its core technology – machine learning – where machine learning models utilize data such as provider networks and population demographics to develop predictive scorecards and identify the best cities to expand to next[9].
An interesting question that I have with regards to healthcare diagnosis is whether machines should be held to a higher standard than humans. According to recent research, doctors significantly outperform algorithms with an accuracy rate of 84.3% vs 51.2% in diagnostic predictions[10]. This means that even doctors are unable to diagnose health problems with 100% accuracy.
Is it then considered “ethical” if Oscar provides its predictions to doctors when its accuracy rate is “merely” on par with current physicians? Or is it only “ethical” to do so when it has a (close to) 100% accuracy rate? Should algorithms be blamed more than doctors for its failed predictions if both of them have the same accuracy rate?
(Word count: 766)
[1] Fry, Erika, and Sy Mukherjee. 2018. “Http://Fortune.Com”. Fortune. http://fortune.com/2018/03/19/big-data-digital-health-tech/.
[2] Tracer, Zachary. 2018. “Bloomberg – Are You A Robot?”. Bloomberg.Com. https://www.bloomberg.com/news/articles/2018-08-14/alphabet-makes-a-375-million-bet-on-obamacare-startup-oscar.
[3] Ng, Andrew. 2018. “What Is Machine Learning? – Introduction | Coursera”. Coursera. https://www.coursera.org/lecture/machine-learning/what-is-machine-learning-Ujm7v.
[4] Xin, Xin. 2018. “The Promises And Limits Of Precision Health – Xin Xin – Medium”. Medium. https://medium.com/@spicyhappy/the-promises-and-limits-of-precision-health-3f29d297caf7.
[5] “Collecting Labeled Data – Amazon Machine Learning”. 2018. Docs.Aws.Amazon.Com. https://docs.aws.amazon.com/machine-learning/latest/dg/collecting-labeled-data.html.
[6] Ramsey, Lydia. 2018. “Health Insurance Startup Oscar Has A New Way To Get All Your Medical Details In One Place For Your Doctor”. Business Insider. https://www.businessinsider.com/oscar-health-clinical-dashboard-2017-6.
[7] Warren, Alan. 2018. “Oscar | Smart, Simple Health Insurance.”. Hioscar.Com. https://www.hioscar.com/blog/connecting-the-dots-with-the-clinical-dashboard.
[8] Soni, Devin. 2018. “Supervised Vs. Unsupervised Learning – Towards Data Science”. Towards Data Science. https://towardsdatascience.com/supervised-vs-unsupervised-learning-14f68e32ea8d.
[9] Thompson, Nicholas. 2018. “Health Care Is Broken. Oscar Health Thinks Tech Can Fix It”. WIRED. https://www.wired.com/story/oscar-health-ceo-mario-schlosser-interview/.
[10] Semigran, BA, Hannah L., David M. Levine, MD, MA, and Shantanu Nundy, MD. 2016. “Comparison Of Physician And Computer Diagnostic Accuracy”. JAMA Internal Medicine. https://jamanetwork.com/journals/jamainternalmedicine/fullarticle/2565684.
If you believe all human lives are equally valuable, then not using AI seems to be clearly unethical – as the technology can dramatically increase the number of patients receiving (even close to) quality care. Yet on an individual level, implementing AI in healthcare means that some people will die because of algorithmic mistakes – which many will find objectionable. Navigating this balance will be a key challenge in the coming years.