AI in Predicting Candidate Potential
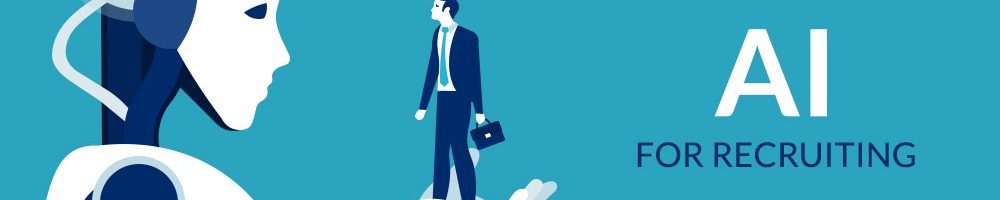
Can an algorithm really evaluate a person's potential?
The use of analytics to predict candidate potential is growing in popularity. Examples abound, such as Teach for America which uses analytics to supplement their selection process for new teachers, or Pymetrics which does analytics as a service for other companies seeking to hire top potential candidates.
The article “Can AI predict candidate potential” (https://www.ciodive.com/news/can-ai-predict-candidate-potential/531828/) reflects on both the promise and dangers of such use. One statement in the article is that algorithms “think” in a way different from humans, and can find patterns such as paramedic experience being correlated with future leadership. I agree that AI can be valuable in surfacing such patterns, but they can also be valuable in surfacing a lack of pattern.
Human recruiters will often look to brand name schools or companies as indicators of potential. However, an algorithm may find that the effect of such a brand name is practically insignificant, and that factors such as years of experience in a functional role matter more instead. Consequently, the lack of pattern can allow the team to consider potentially talented candidates who would otherwise have been missed.
However, humans should continue to be involved – as the article suggests, AI should be a complement, not a replacement. For instance, human guidance is needed to correct for irrelevant patterns like the correlation between a Swiss origin and being a good fit for the clock industry. On this, I entirely agree: during my time with IBM Watson, I worked on multiple products (using AI, though not people analytics) where I constantly emphasized to clients that it was not meant to replace humans, only to assist in their work and provide a second viewpoint. However, not all the clients were happy to hear this: more than one wanted to replace their workforce with the algorithm, for cost-cutting purposes. It’s important for businesses to understand that although AI is a valuable supplement, final decisions should always be given to a human, because (1) they can correct for irrelevant patterns, (2) it gives a sense of control and ownership, and (3) it means a decision can be appealed to a human – not an algorithm.
The article also discusses how AI can unintentionally “replicate, and magnify, existing disparities in a workplace”. Consequently, the company Gloat in the article purposely excludes variables such as gender, race, or age, as well as qualities like golfing or skiing which can indicate socioeconomic status. While I view this as good in theory, I think solving the bias problem by excluding variables is very difficult in practice.
For one example, we can look to Amazon’s failed project in using AI to hire: https://www.reuters.com/article/us-amazon-com-jobs-automation-insight/amazon-scraps-secret-ai-recruiting-tool-that-showed-bias-against-women-idUSKCN1MK08G. The Amazon algorithm showed a bias against women, because its dataset was based on the last 10 years of applicants, reflecting a pipeline of mostly men. The algorithm had ‘learned’ that mostly men had been selected in the past, so it began to favor verbs more commonly found on male engineers’ resumes (e.g. ‘executed’ and ‘captured’), among other biased behaviors like penalizing the names of women’s colleges. In my view, it would not be practical to exclude the verbs of every resume bullet point, because they are closely tied into the person’s description of what they accomplished. Consequently, controlling for ‘bias variables’ may be more practical than removing them all, but it’s a difficult issue to solve. Possibly a better dataset (e.g. less male in Amazon’s case) would help, but then bias may be introduced in the selection of the dataset.
To summarize, the key to using AI well in predicting candidate potential lies in (1) seeking both patterns and lack of patterns (2) using AI only as a supplement to a human’s final decision (3) carefully considering how to deal with the possibility of perpetuating bias through the AI results.
Very interesting article, and in a way very related to our blg post about using predictive analysis and algorithms to scout talent for soccer clubs.
A couple of thoughts. First, i completely agree that these platforms and systems should act more as compliments rather than substitutes for human based recruitment. I think that together, both can capture both types of patterns identified above.
Additionally, i keep thinking whether this discussion would be different based on the level of employees or job functions corporations are recruiting for. Thinking back on our Promo exercise, it was evident that we cant rely solely on data to select the next VP or high ranking executive. However, would data work perfectly to sort through hundreds of applicants for an order fulfillment job or grocery store cashier? Not that these jobs are in any way less important than others, particularly true in these current days, but the technical and soft skills required are less demanding.
Katherine, thank you for your thoughtful post!
Your point about bias reminded me of GROW case, where we discussed the gender bias inherent in data. The fact that MC used the existing talents as a way to predict best hires likely resulted in reinforcing gender bias since they only have 20% of women in the entire organization, 10% at managerial level, and 0% at the executive level.
To prevent us from falling prey to these gender bias, I think it is important for humans to have sufficient understanding about the dangers behind analytics. Otherwise, we may want to rely too much on analytics, as your former client suggested to replace analytics with humans. In fact, I connected with the team at MC after the class about their current practices (I knew them as I helped them find female talents before), and they told me that all the team members are taking People Analytics course on coursera, as they felt that they were not being able to harness the power of analytics in the best way. (https://www.coursera.org/learn/wharton-people-analytics)
Thank you for sharing your perspective on this matter, Katherine. I fully agree that highlighting both the patterns and the lack of patterns is crucial to taking full advantage of AI. After all, these two boxes are mutually exclusive, so by considering both, we are being completely exhaustive. That said, being exhaustive comes with costs as well (resources, money, time, etc.). An open question I have is how do we draw guidelines on what patterns or what “lack of patterns” to consider before running the risk of boiling the ocean.
Katherine – thank you for this interesting post.
Your example of bias in Amazon’s hiring practices made me think about whether companies have a legal responsibility to ensure that their algorithms do not disciminate any of the protected classes (gender, age, country of origin, etc.). I would like to say yes, but i also see how that could be very constraining for companies trying to leverage more analytics. Your specific example of how certain verbs are used more often by men is interesting because it is non-obvious unless you have specifically investigated the correlation. Do companies need to exhaustively look for all bias across all variables and try to eliminate them? Is this even possible? Or can we tolerate some level of bias? Where do we draw the line?
Thanks again
Thank you for sharing this! I agree with all of your conclusions. I thought about the same point Georges mentioned: whether this discussion is relevant for all job levels and functions. Maybe an algorithm can predict candidates’ potential if the job is relatively basic.