BigML – Early to the Party

How BigML is democratizing the tools of Big Data, making machine learning 'easy & beautiful.'
Second only to the surfer, concern for where & when the next wave’ll occur is nearly a full-time job for some in the tech industry (in fact, for people like Shingy, AOL’s ‘Digital Prophet,’ pictured right, it is a full-time job).
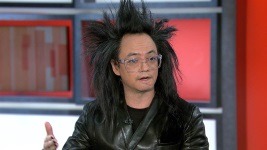
While even the layest of persons might’ve heard about Big Data as the next wave, though, the details surrounding the actual implications and implementations haven’t quite made it down from the clouds. Everyone knows that the glut of data will change everything, but few seem to know how.
As of this writing, the benefits of machine learning & data mining (used interchangeably with “ML” hereafter, for simplicity)–i.e., the use of algorithms to recognize patterns and make predictions based on inferences from large piles of data, are realized only by those with the expertise to employ the techniques, or those who can afford their services. But much in the same way that spreadsheets began as tools only for the most adventurous circa 1960s, the tools of the ML trade won’t remain esoteric for very long.
It’s into this transitional phase, from dumb data to smart data, that BigML entered less than half-a-decade ago. BigML offers machine learning in a SaaS framework (MLaaS, as they call it), in an effort to “democratize machine learning.” Their product allows users to leverage world-class machine-learning tech for minimal subscription costs, all without having to learn a programming language like R or Python. The company presents some of its users’ creations, from the significant (what factors best predict poverty?), to the… well, interesting, at least (can whether or not you’ve been skydiving predict how you like your steak cooked?).
Providing user-friendly (or, at least, developer-friendly) ML isn’t new. In fact, it’s often hard to differentiate today’s ML insights from yesterday’s analytics-driven decisions. In that respect, many of the analytics players are on the field at the enterprise-level, ML being a natural extension of the services with which they’ve always buttered their bread. But like their historical offerings, the services aren’t even remotely accessible to end-users.
The next tier contains the more household-names: Amazon, Microsoft, Google, and others. Just like many of their SaaS products, companies can integrate these products with relative ease compared to their mega-enterprise rivals, but the services aren’t without limitation. BigML made their intern do a comparison that’s worth a read, but in summary, these services often tie the use of their MLaaS to the use of their other products.
As you can imagine, this leaves a good deal of whitespace–everything from the hacker in her basement trying to figure out forest-fire trajectories, to the medium-sized business with more locally-housed data than they know what to do with. With BigML, these folks have access to the knowledge within their data that would’ve otherwise remained hidden–a pretty stellar creation of value.
The value capture is simple–subscription fees. The risk of so simple a platform, though, is stickiness of users–while a learning curve can keep users out, it can also keep them in. By remaining committed to the philosophy of democratizing these tools, though, I suspect BigML will make up in loyalty what they may lack in structural barriers to churn.
ML can do some remarkable things. From the interesting (predicting a young woman’s pregnancy before she told her parents), to the dubiously important (writing a neural network program that teaches itself how to play Mario), to the actually-important (reshaping the fight against cancer). But just like computer programming, when the wider world gets its hands on it, innovation will grow by orders of magnitude. BigML is one of the first players to this space, and while the future will be fraught with competition against lethally capable entrants, their success will be remembered as the first of many steps in the Next Big Wave.
right?!?
I NO!
Really liked your post, especially the playful prose! BigML is certainly winning by expanding powerful data science (ML) tools to users without millions of dollars to invest in their own scientists and infrastructure. As you say, a lot of important research is facilitated by BigML, which is great. My two reservations revolve around unstructured data and inexperienced users. I’ve only given their site a cursory glance; but it would appear that their tools, while sophisticated, don’t help users where they most often need it – when “wrangling” disparate, often dirty, unstructured data. As someone mentioned in class, this is the newest “wave” in business analytics and poses one of the greatest challenges to even the most seasoned data scientists. Though it may be the case that their target market is only interested in highly structured data such as transaction history.
Secondly, one of the most common pitfalls we see is the old “correlation does not imply causation” issue. Data science is an art as much as it is a science, and it’s often the case (spoken from personal experience) that results that come out of ML algorithms are almost too good to be true because they are. Perhaps an incredible correlation appears between bank patrons experiencing bad service and time of day on weekdays, leading the bank to invest heavily in customer service reps only to discover that people going to the bank at 2:30pm on a Wednesday are probably out of work and likely to have more financial stresses than employed individuals. Patrons are unhappy no matter the quality of service because their external situation taints their banking experience and so the bank has wasted a lot of money attempting to address an issue that isn’t as easily remedied as they thought. An experienced data scientist would account and test these hypotheses, but a green ML user would not. BigML certainly offers its users a lot of rope for analyzing data, I just worry it may offer more than enough for customers to hang themselves.